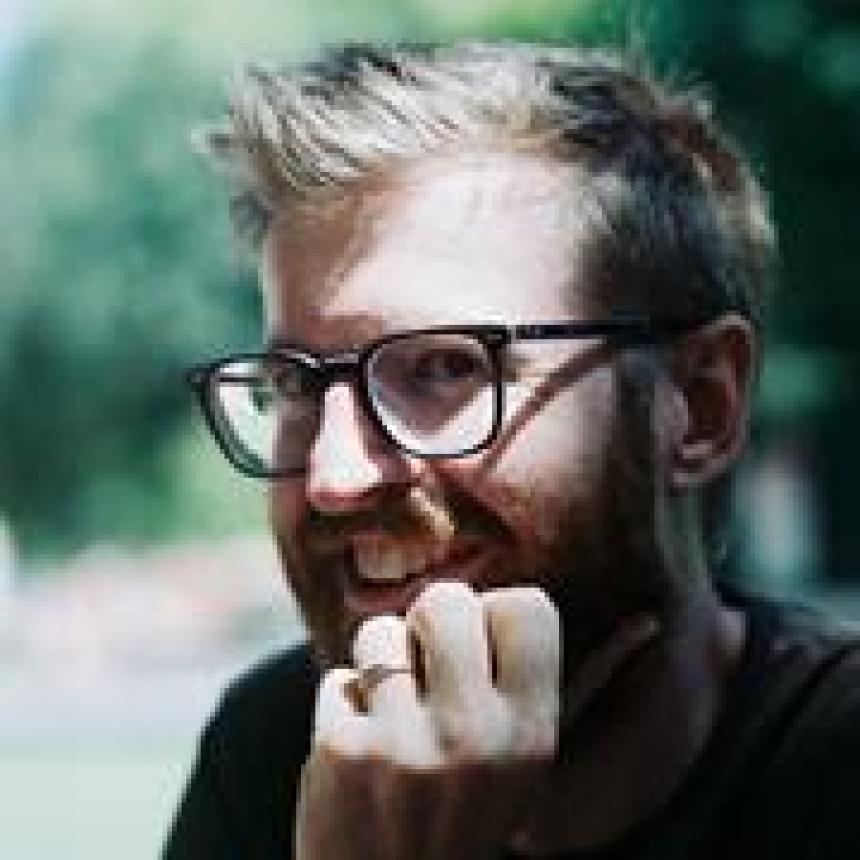
Dr John Maclean
Lecturer
School of Computer and Mathematical Sciences
Faculty of Sciences, Engineering and Technology
Eligible to supervise Masters and PhD - email supervisor to discuss availability.
I am a Lecturer in Data Science and Statistics, with chief interests in Data Assimilation and numerical multiscale methods. For access to preprints, please follow the arXiv link below `External Profiles', at the bottom of the page.
Prospective students are encouraged to contact me directly, but may find some inspiration in the following:
Data Assimilation (DA) - the mathematical and statistical question of how to combine an uncertain model forecast with data. I am interested in:
- Coherent structure DA, that is, employing low-dimensional structures in the DA update in place of the original data. Research questions here may tend towards statistics (how do measurement errors in data create measurement errors in coherent structures?) or applied maths (from the oceanographic/atmospheric sciences literature; how should we employ coherent structures, and on what scales?).
- Projected DA, that is, employing projections to split one DA problem into several. The advantage is that one can use a DA method with high accuracy on key parts of the DA problem, and use a DA method suited to high-dimensional inference on the remainder of the DA problem. Some overlap with coherent structures; the key in projected DA is translating dynamical information to statistical information.
- Non-Gaussian problems; DA algorithms are often founded on the assumption that measurement errors are distributed normally. However there are counter-examples, and work has shown that DA methods constructed for non-Gaussian measurement errors are promising. (This would be a new research area for me - based on work by Craig Bishop.)
- Surrogate DA, the design of DA methods where a statistical surrogate is trained to empower a large ensemble of forecasts to be approximated from a small number of computationally intensive runs of the physical model. Many open questions - see my preprint with A/Prof. Elaine Spiller.
Numerical Multiscale Methods - key focus is on Projective Integration, that accelerates simulation of stiff systems, and patch dynamics, that accelerates simulation of systems with fine and coarse spatial components.
- Projective Integration for stochastic systems; there is a wealth of literature on this topic, but to my view two questions remain. First is how to implement an accurate, fast PI solver for stochastic systems with unknown slow and fast variables. Second is how to modify such methods to nonstandard slow-fast systems of the sort discussed in http://dx.doi.org/10.1137/19M1242677
- Patch dynamics; recent work has developed an adaptive moving patch scheme. The scheme can simulate moving fine-scale meshes that come together to form shocks at unknown locations in the spatial domain. A project here might focus on extending and applying the moving patch dynamics to travelling wave problems.
Thanks for coming this far! Please accept a beautiful picture of adaptive moving patches simulating a problem with heterogeneous advection and diffusion terms; the inset shows details of the little black box.
-
Appointments
Date Position Institution name 2021 - ongoing Lecturer University of Adelaide 2018 - 2021 Postdoc University of Adelaide 2015 - 2018 Postdoc Univerity of North Carolina at Chapel Hill -
Education
Date Institution name Country Title 2011 - 2014 University of Sydney Australia PhD
-
Journals
Year Citation 2025 Xiourouppa, A. H., Mikhin, D., Humphries, M., & Maclean, J. (2025). Theoretical Insights for Bearings-Only Tracking in Log-Polar Coordinates. IEEE Transactions on Aerospace and Electronic Systems, 1-12.
Scopus12025 Shorten, D. P., Humphries, M., Maclean, J., Yang, Y., & Roughan, M. (2025). Optimal proposal particle filters for detecting anomalies and manoeuvres from two line element data. Acta Astronautica, 228, 709-723.
2025 Blake, L. A. A., Maclean, J., & Balasuriya, S. (2025). Rigorous convergence bounds for stochastic differential equations with application to uncertainty quantification. Physica D Nonlinear Phenomena, 481, 134742.
2024 O’loughlin, L., Maclean, J., & Black, A. (2024). Neural Likelihood Approximation for Integer Valued Time Series Data. Transactions on Machine Learning Research, 2024. 2024 Shermon, S., Maclean, J., Shim, R., & Kim, C. H. (2024). Neuropsychiatric Side Effects After Lumbosacral Epidural Steroid Injections: A Prospective Cohort Study. PAIN PHYSICIAN, 27(3), 12 pages. 2024 Maclean, J., Remick, S., Shim, W. J., Chakravorty, A., & Kim, C. (2024). Electronic Cigarette (E-Cig) Use in the Chronic Pain Population. PAIN PHYSICIAN, 27(2), 6 pages. 2024 Morris, D., Maclean, J., & Black, A. J. (2024). Computation of random time-shift distributions for stochastic population models.. Journal of mathematical biology, 89(3), 33.
2024 Loch, A., Sexton, S., Maclean, J., O’Connor, P., Adamson, D., & Scholz, G. (2024). Increased monetary equity and health wellbeing benefits for marginal urban socioeconomic groups from access to green space. Urban Forestry and Urban Greening, 102, 128576-1-128576-10.
Scopus42024 Maclean, J., & Van Vleck, E. S. (2024). DECOMPOSITION OF LIKELIHOODS AND TECHNIQUES FOR MULTI-SCALE DATA ASSIMILATION. DISCRETE AND CONTINUOUS DYNAMICAL SYSTEMS-SERIES B, 0(0), 24 pages.
2024 Santeramo, F. G., Martinez-Gomez, V., Marquez-Ramos, L., & Lamonaca, E. (2024). The import effects of the Entry Price System. Journal of Agricultural and Resource Economics, 49(1), 143-161.
Scopus22023 Robbins, C., Blyth, M. G., MacLean, J., & Binder, B. J. (2023). A method to calculate inverse solutions for steady open channel free-surface flow. Journal of Fluid Mechanics, 977, 22 pages.
Scopus22023 O'Loughlin, L., Maclean, J., & Black, A. (2023). Neural Likelihood Approximation for Integer Valued Time Series Data. 2023 Shorten, D. P., Yang, Y., Maclean, J., & Roughan, M. (2023). Wide-Scale Monitoring of Satellite Lifetimes: Pitfalls and a Benchmark Dataset. Journal of Spacecraft and Rockets, 60(6), 1-5.
Scopus52022 Albarakati, A., Budišić, M., Crocker, R., Glass-Klaiber, J., Iams, S., Maclean, J., . . . Van Vleck, E. S. (2022). Model and data reduction for data assimilation: Particle filters employing projected forecasts and data with application to a shallow water model. Computers and Mathematics with Applications, 116, 194-211.
Scopus14 WoS52022 Maclean, J., Bunder, J. E., Kevrekidis, I. G., & Roberts, A. J. (2022). Adaptively Detect and Accurately Resolve Macro-scale Shocks in an Efficient Equation-Free Multiscale Simulation. SIAM Journal on Scientific Computing, 44(4), A2557-A2581.
Scopus22022 Johnson, S., Maclean, J., Vozzo, R. F., Koerber, A., & Humphries, M. A. (2022). Don't throw the student out with the bathwater: online assessment strategies your class won't hate. International Journal of Mathematical Education in Science and Technology, 53(3), 1-12.
Scopus32021 Maclean, J., & Spiller, E. T. (2021). A surrogate-based approach to nonlinear, non-Gaussian joint state-parameter data assimilation. Foundations of Data Science, 3(3), 589-614.
2021 Maclean, J., Bunder, J. E., Kevrekidis, I. G., & Roberts, A. J. (2021). An Equation Free algorithm accurately simulates macroscale shocks arising from heterogeneous microscale systems. IEEE Journal on Multiscale and Multiphysics Computational Techniques, 6, 8-15.
Scopus3 WoS22021 Maclean, J., & Van Vleck, E. S. (2021). Particle filters for data assimilation based on reduced-order data models. Quarterly Journal of the Royal Meteorological Society, 147(736), 1892-1907.
Scopus9 WoS42021 Maclean, J., Bunder, J. E., & Roberts, A. J. (2021). A toolbox of equation-free functions in Matlab/Octave for efficient system level simulation. Numerical Algorithms, 87(4), 1729-1748.
Scopus9 WoS42021 McCradden, M. D. (2021). When is accuracy off-target?. Translational Psychiatry, 11(1), 369.
Scopus8 Europe PMC52020 Maclean, J., Bunder, J. E., Roberts, A. J., & Kevrekidis, I. G. (2020). A multiscale scheme accurately simulates macroscale shocks in an
equation-free framework.2020 Zhang, H., Lapointe, B. T., Anthony, N., Azevedo, R., Cals, J., Correll, C. C., . . . Barr, K. (2020). Discovery of <i>N</i>-(Indazol-3-yl)piperidine-4-carboxylic Acids as RORγt Allosteric Inhibitors for Autoimmune Diseases. ACS MEDICINAL CHEMISTRY LETTERS, 11(2), 114-119.
2017 Maclean, J., Santitissadeekorn, N., & Jones, C. K. (2017). A coherent structure approach for parameter estimation in Lagrangian Data Assimilation. Physica D: Nonlinear Phenomena, 360, 36-45.
Scopus13 WoS92015 Maclean, J., & Gottwald, G. (2015). On convergence of higher order schemes for the projective integration method for stiff ordinary differential equations. Journal of Computational and Applied Mathematics, 288, 44-69.
Scopus7 WoS62015 Maclean, J. (2015). A note on implementations of the Boosting Algorithm and Heterogeneous Multiscale Methods. SIAM Journal on Numerical Analysis, 53(5), 2472-2487.
Scopus2 WoS22015 Bethapudi, S., Ritchie, D., Bongale, S., Gordon, J., MacLean, J., & Mendl, L. (2015). Data analysis and review of radiology services at Glasgow 2014 Commonwealth Games. SKELETAL RADIOLOGY, 44(10), 1477-1483.
2014 Maclean, J., & Gottwald, G. (2014). On convergence of the projective integration method for stiff ordinary differential equations. Communications in Mathematical Sciences, 12(2), 235-255.
Scopus4 WoS3 -
Book Chapters
Year Citation 2024 Gilbert, S., Glorie, S., & Zack, T. (2024). In situ beta decay dating by LA-ICP-MS/MS: applications. In J. G. Shellnutt, S. W. Denyszyn, & K. Suga (Eds.), Methods and Applications of Geochronology (pp. 243-295). Elsevier.
DOI Scopus32023 Johnson, S., Maclean, J., Vozzo, R. F., Koerber, A., & Humphries, M. A. (2023). Don't throw the student out with the bathwater: online assessment strategies your class won't hate. In Takeaways from Teaching through a Pandemic (pp. 69-80). Routledge.
DOI2019 Budhiraja, A., Friedlander, E., Guider, C., Jones, C. K., & Maclean, J. (2019). Assimilating Data into Models. In A. E. Gelfand, M. Fuentes, J. A. Hoeting, & R. L. Smith (Eds.), Handbook of Environmental and Ecological Statistics (1 ed., pp. 687-708). Florida; USA: CRC Press. -
Conference Papers
Year Citation 2022 Piyevsky, B., Maclean, J., Li, A., Rhodes, S., Prunty, M., Jesse, E., . . . Callegari, M. (2022). IMPACT AND IMPLICATIONS OF THE COVID-19 PANDEMIC ON UROLOGIC TRAINING. In JOURNAL OF UROLOGY Vol. 207 (pp. E513-E514). LIPPINCOTT WILLIAMS & WILKINS. 2017 Pesnot, T., Mahale, S., MacFaul, P., Maclean, J., Phillips, C., Bingham, M., . . . Armer, R. (2017). Development of 2" generation indoleamine 2,3-dioxygenase 1 (IDO1) selective inhibitors. In CANCER RESEARCH Vol. 77 (pp. 2 pages). DC, Washington: AMER ASSOC CANCER RESEARCH.
DOI2015 Khudoley, A., Chamberlain, K., Ershova, V., Sears, J., Prokopiev, A., MacLean, J., . . . Chipley, D. (2015). Proterozoic supercontinental restorations: Constraints from provenance studies of Mesoproterozoic to Cambrian clastic rocks, eastern Siberian Craton. In PRECAMBRIAN RESEARCH Vol. 259 (pp. 78-94). RUSSIA, Moscow: ELSEVIER.
DOI1984 MACLEAN, J., & BECKER, R. (1984). THE IMPACT OF THE INTRODUCTION OF GERIATRIC ASSESSMENT BEDS INTO A LONG-TERM CARE UNIT. In GERONTOLOGIST Vol. 24 (pp. 121). GERONTOLOGICAL SOCIETY AMER. -
Report for External Bodies
Year Citation 2022 Loch, A., Sexton, S., Scholz, G., Maclean, J., & O'Connor, P. (2022). Willingness to Pay and Avoided Health Costs associated with Metropolitan Parks. Adelaide, SA: South Australian Department for Environment and Water. -
Preprint
Year Citation 2024 Xiourouppa, A. H., Mikhin, D., Humphries, M., & Maclean, J. (2024). An insightful approach to bearings-only tracking in log-polar
coordinates.2024 Blake, L., Maclean, J., & Balasuriya, S. (2024). Unifying Lyapunov exponents with probabilistic uncertainty
quantification.2023 Shorten, D. P., Maclean, J., Humphries, M., Yang, Y., & Roughan, M. (2023). Optimal Proposal Particle Filters for Detecting Anomalies and Manoeuvres
from Two Line Element Data.2023 Blake, L., Maclean, J., & Balasuriya, S. (2023). The convergence of stochastic differential equations to their
linearisation in small noise limits.
-
Current Higher Degree by Research Supervision (University of Adelaide)
Date Role Research Topic Program Degree Type Student Load Student Name 2025 Co-Supervisor Predicting bottom topography underneath free-surface flow Master of Philosophy Master Full Time Miss Caitlin Lydia Anchor 2025 Co-Supervisor Methods for inference and forecasting in mechanistic models with unresolved processes Doctor of Philosophy Doctorate Full Time Mr Liam Andrew Alex Blake 2024 Principal Supervisor Scalable algorithms for non-linear target tracking Doctor of Philosophy Doctorate Full Time Miss Athena Helena Xiourouppa 2023 Co-Supervisor Kalman Filtering and Optimization Methods for Object Tracking Master of Philosophy Master Full Time Miss Vivienne Mei-Larn Niejalke 2023 Co-Supervisor Predicting Clinical Need During Dispatch to Guide Ambulance Response Doctor of Philosophy Doctorate Part Time Mr Trevor Paul Matthews 2022 Co-Supervisor More than just a result: using cycle threshold values in household epidemic modelling Doctor of Philosophy Doctorate Full Time Mr Dylan John Morris 2021 Co-Supervisor Modelling and Quantification of Food Deserts Doctor of Philosophy under a Jointly-awarded Degree Agreement with Doctorate Part Time Miss Tayla Paige Broadbridge -
Past Higher Degree by Research Supervision (University of Adelaide)
Date Role Research Topic Program Degree Type Student Load Student Name 2022 - 2024 Co-Supervisor Computable Characterisations of Uncertainty in Differential Equations Master of Philosophy Master Full Time Mr Liam Andrew Alex Blake 2022 - 2024 Co-Supervisor An Analysis of Bias in Australian Television Media Master of Philosophy Master Full Time Miss Irulan Claire Prowse Murphy 2022 - 2023 Co-Supervisor Likelihood-Free Inference for Discrete Time Series Data Using Machine Learning Master of Philosophy Master Full Time Mr Luke Phillip O'Loughlin 2021 - 2023 Co-Supervisor A Modelling Framework for Estimating the Risk of Importation of a Novel Disease Master of Philosophy Master Full Time Mr Antonio Max Parrella 2019 - 2021 Co-Supervisor Lagrangian Coherent Data Assimilation for Chaotic Geophysical Systems Master of Philosophy Master Full Time Ms Rose Joy Crocker
-
Community Engagement
Date Title Engagement Type Institution Country 2021 - 2021 Colloquium Organiser Scientific Community Engagement University of Adelaide - 2021 - ongoing Outreach Committee Scientific Community Engagement University of Adelaide -
Connect With Me
External Profiles