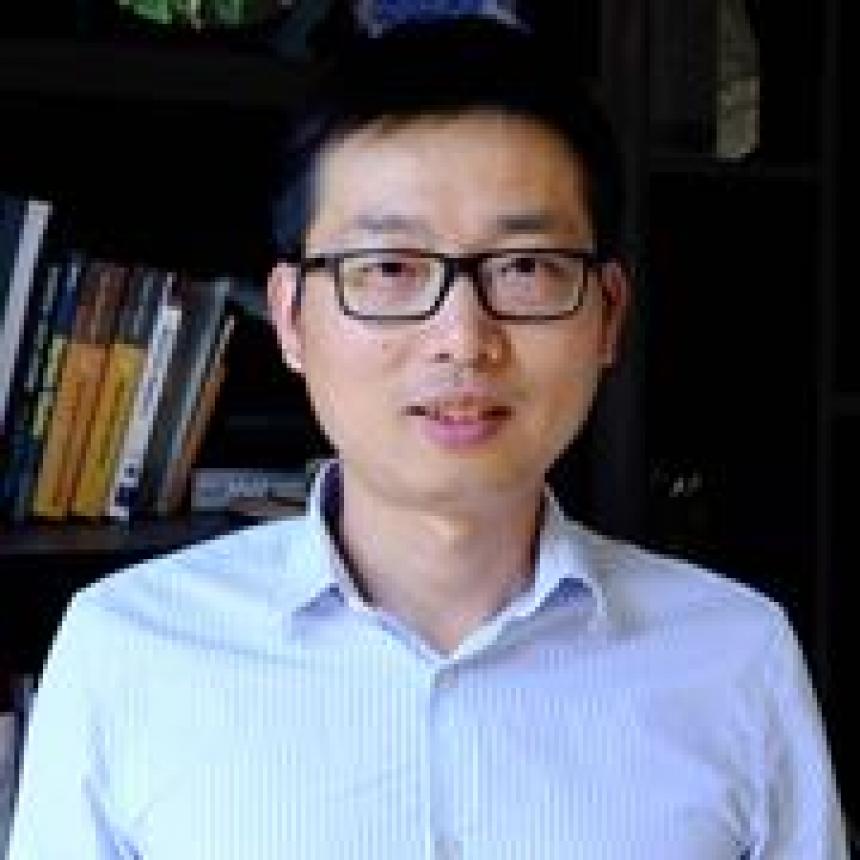
Professor Javen Shi
Professor
School of Computer and Mathematical Sciences
Faculty of Sciences, Engineering and Technology
Eligible to supervise Masters and PhD - email supervisor to discuss availability.
Professor Javen Qinfeng Shi is the Founding Director of Causal AI Group at the University of Adelaide, and one of the directors at Australian Institute for Machine Learning (AIML). His research interests include causation, AI, mind and metaphysics. Google Scholar ranks him 4th globally in causation and 7th in probabilistic graphical models. He served as a panellist for the Responsible AI Think Tank from 2022 to 2024 and currently holds the position of an AI Industry Forum panellist from 2024 onward, actively contributing to the cultivation of the national and state AI ecosystem. He has transferred his research to diverse industries including material discovery, agriculture, mining, sport, manufacturing, bushfire, health and education.
Recent awards include: 1) 1st place at Open Catalyst Challenge at NeurIPS AI for Science 2023, using AI to discover energy material; 2) Won AUS/NZ Bushfire Data Quest 2020 using AI to predict fire spread, which led to winning a Citizen Science Grant in 2021, and released a bushfire app NOBURN in 2023 (over 50 media coverages); 3) Finalist of SA Department of Energy and Mining Gawler Challenge 2020 (over 2k participants from 100+ countries) with his team's work being considered as ''The most innovative modelling'' by the judge panel; 4) 2nd place in Explorer Challenge 2019 (over 1k entries from 62 countries); 5) 1st place at SAIC Volkswagen Logistics Innovation Day for Smart Manufacturing 2019.
We try to understand and causally influence the underlying distributions and processes to help and serve humanity.
Our world is undergoing inevitable and tumultuous changes. Causality, operating beneath the veneer of cause and effect, is essentially the way of change. Our causal AI methods can identify the root causes, discover latent variables, build immunity from spurious correlations, improve generalistion to diverse domains and distribution shifts, model the consequence of interventions, and answer What-If counterfactual questions. More importantly, causal AI holds the key to answer the reverse question: What is the ideal sequence of interventions, given resources or budgets, to optimise future outcomes?
-
Appointments
Date Position Institution name 2020 - ongoing Professor University of Adelaide 2018 - 2019 Associate Professor University of Adelaide 2015 - 2017 Senior Lecturer University of Adelaide 2012 - 2014 ARC DECRA fellow University of Adelaide 2010 - 2011 Senior Research Associate University of Adelaide -
Awards and Achievements
Date Type Title Institution Name Country Amount 2012 Award ARC Discovery Early Career Researcher Award ARC Australia - -
Language Competencies
Language Competency Chinese (Mandarin) Can read, write, speak, understand spoken and peer review English Can read, write, speak, understand spoken and peer review -
Education
Date Institution name Country Title 2006 - 2010 Australian National University, Canberra Australia PhD 2003 - 2006 Northwestern Polytechnical University, Xi'an China Master 1999 - 2003 Northwestern Polytechnical University, Xi'an China Bachelor -
Research Interests
-
Journals
Year Citation 2024 Zhang, X., Zhang, Z., Chinnici, A., Sun, Z., Shi, J. Q., Nathan, G. J., & Chin, R. C. (2024). Physics-informed data-driven unsteady Reynolds-averaged Navier-Stokes turbulence modeling for particle-laden jet flows. Physics of Fluids, 36(5), 23 pages.
2023 Yan, Q., Gong, D., Wang, P., Zhang, Z., Zhang, Y., & Shi, J. Q. (2023). SharpFormer: Learning Local Feature Preserving Global Representations for Image Deblurring. IEEE Transactions on Image Processing, 32, 2857-2866.
Scopus5 WoS12023 Meng, J., Wang, Z., Ying, K., Zhang, J., Guo, D., Zhang, Z., . . . Chen, S. (2023). Human Interaction Understanding With Consistency-Aware Learning. IEEE Transactions on Pattern Analysis and Machine Intelligence, 45(10), 11898-11914.
Scopus3 Europe PMC12023 Yan, Q., Liu, S., Xu, S., Dong, C., Li, Z., Shi, J. Q., . . . Dai, D. (2023). 3D Medical image segmentation using parallel transformers. Pattern Recognition, 138, 1-14.
Scopus222023 Li, X., Li, H., Zhang, Z., Shi, J. Q., Jiao, Y., & Qiao, S. -Z. (2023). Active-learning accelerated computational screening of A₂B@NG catalysts for CO₂ electrochemical reduction. Nano Energy, 115, 108695-1-108695-9.
Scopus32023 Yan, Q., Fruzangohar, M., Taylor, J., Gong, D., Walter, J., Norman, A., . . . Coram, T. (2023). Improved genomic prediction using machine learning with Variational Bayesian sparsity. Plant Methods, 19(1), 1-14.
2023 Li, X., Shi, J. Q., & Page, A. J. (2023). Discovery of Graphene Growth Alloy Catalysts Using High-Throughput Machine Learning. Nano Letters, 23(21), 9796-9802.
Scopus12023 Yuwono, J. A., Li, X., Doležal, T. D., Samin, A. J., Shi, J. Q., Li, Z., & Birbilis, N. (2023). A computational approach for mapping electrochemical activity of multi-principal element alloys. npj Materials Degradation, 7(1), 87-1-87-11.
Scopus22023 Zhao, Y., Li, H., Shan, J., Zhang, Z., Li, X., Shi, J. Q., . . . Li, H. (2023). Machine Learning Confirms the Formation Mechanism of a Single-Atom Catalyst via Infrared Spectroscopic Analysis. Journal of Physical Chemistry Letters, 14(49), 11058-11062.
2022 Sun, W., Gong, D., Shi, J. Q., van den Hengel, A., & Zhang, Y. (2022). Video super-resolution via mixed spatial-temporal convolution and selective fusion. Pattern Recognition, 126, 1-14.
Scopus11 WoS62022 Yan, Q., Gong, D., Shi, J. Q., den Hengel, A. V., Sun, J., Zhu, Y., & Zhang, Y. (2022). High dynamic range imaging via gradient-aware context aggregation network. Pattern Recognition, 122, 16 pages.
Scopus18 WoS122022 Doan, B. G., Abbasnejad, E., Shi, J. Q., & Ranashinghe, D. C. (2022). Bayesian Learning with Information Gain Provably Bounds Risk for a Robust Adversarial Defense. INTERNATIONAL CONFERENCE ON MACHINE LEARNING, VOL 162, 162, 15 pages. 2022 Ghiasi, A., Moghaddam, M. K., Ng, C. T., Sheikh, A. H., & Shi, J. Q. (2022). Damage classification of in-service steel railway bridges using a novel vibration-based convolutional neural network. Engineering Structures, 264, 114474-1-114474-16.
Scopus24 WoS52022 Wang, X., Liu, L., & Shi, J. Q. (2022). Computationally Efficient Dilated Convolutional Model for Melody Extraction. IEEE Signal Processing Letters, 29, 1599-1603.
Scopus12022 Parvaneh, A., Abbasnejad, E., Wu, Q., Shi, Q., & Van Den Hengel, A. (2022). Show, price and negotiate: a negotiator with online value look-ahead. IEEE Transactions on Multimedia, 24, 1426-1434.
Scopus22021 Abedin, A., Ehsanpour, M., Shi, Q., Rezatofighi, H., & Ranasinghe, D. C. (2021). Attend And Discriminate: Beyond the State-of-the-Art for Human Activity Recognition using Wearable Sensors.. Proceedings of the ACM on Interactive, Mobile, Wearable and Ubiquitous Technologies, 5(1), 1-22.
Scopus47 WoS262021 Wang, Y., Gong, D., Yang, J., Shi, Q., Hengel, A. V. D., Xie, D., & Zeng, B. (2021). Deep Single Image Deraining via Modeling Haze-Like Effect. IEEE Transactions on Multimedia, 23, 2481-2492.
Scopus15 WoS72021 Neshat, M., Nezhad, M. M., Abbasnejad, E., Mirjalili, S., Groppi, D., Heydari, A., . . . Wagner, M. (2021). Wind turbine power output prediction using a new hybrid neuro-evolutionary method. Energy, 229, 120617-1-120617-24.
Scopus71 WoS512021 Moghaddam, M. K., Abbasnejad, E., Wu, Q., Shi, J., & Hengel, A. V. D. (2021). Learning for Visual Navigation by Imagining the Success. 2021 Yan, Q., Wang, B., Zhang, W., Luo, C., Xu, W., Xu, Z., . . . You, Z. (2021). An attention-guided deep neural network with multi-scale feature fusion for liver vessel segmentation. IEEE Journal of Biomedical and Health Informatics, 25(7), 2629-2642.
Scopus66 WoS43 Europe PMC182021 Yan, Q., Wang, B., Zhang, L., Zhang, J., You, Z., Shi, Q., & Zhang, Y. (2021). Towards accurate HDR imaging with learning generator constraints. Neurocomputing, 428, 79-91.
Scopus10 WoS102021 Sun, W., Gong, D., Shi, Q., van den Hengel, A., & Zhang, Y. (2021). Learning to zoom-in via learning to zoom-out: real-world super-resolution by generating and adapting degradation. IEEE Transactions on Image Processing, 30, 1-16.
Scopus21 WoS17 Europe PMC12021 Yan, Q., Wang, B., Gong, D., Luo, C., Zhao, W., Shen, J., . . . You, Z. (2021). COVID-19 chest CT image segmentation network by multi-scale fusion and enhancement operations. IEEE Transactions on Big Data, 7(1), 13-24.
Scopus75 WoS43 Europe PMC182021 Yan, Q., Gong, D., Shi, J. Q., van den Hengel, A., Shen, C., Reid, I., & Zhang, Y. (2021). Dual-attention-guided network for ghost-free high dynamic range imaging. International Journal of Computer Vision, 130(1), 19 pages.
Scopus24 WoS92021 Rezatofighi, H., Kaskman, R., Taghizadeh Motlagh, S. F., Shi, Q., Milan, A., Cremers, D., . . . Reid, I. D. (2021). Learn to Predict Sets Using Feed-Forward Neural Networks. IEEE Transactions on Pattern Analysis and Machine Intelligence, 44(12), 1-15.
Scopus52020 Yan, Q., Zhang, L., Liu, Y., Zhu, Y., Sun, J., Shi, Q., & Zhang, Y. (2020). Deep HDR imaging via A non-local network. IEEE Transactions on Image Processing, 29, 4308-4322.
Scopus128 WoS39 Europe PMC72020 Gong, D., Zhang, Z., Shi, Q., van den Hengel, A., Shen, C., & Zhang, Y. (2020). Learning deep gradient descent optimization for image deconvolution. IEEE Transactions on Neural Networks and Learning Systems, 31(12), 5468-5482.
Scopus64 WoS46 Europe PMC22020 Yan, Y., Tan, M., Tsang, I., Yang, Y., Shi, Q., & Zhang, C. (2020). Fast and Low Memory Cost Matrix Factorization: Algorithm, Analysis and Case Study. IEEE Transactions on Knowledge and Data Engineering, 32(2), 288-301.
Scopus3 WoS32020 Yan, Q., Wang, B., Gong, D., Luo, C., Zhao, W., Shen, J., . . . You, Z. (2020). COVID-19 Chest CT Image Segmentation -- A Deep Convolutional Neural
Network Solution.2020 Zhang, L., Wei, W., Shi, Q., Shen, C., van den Hengel, A., & Zhang, Y. (2020). Accurate tensor completion via adaptive low-rank representation. IEEE Transactions on Neural Networks and Learning Systems, 31(1), 4170-4184.
Scopus16 WoS132020 Abbasnejad, M. E., Shi, Q., van den Hengel, A., & Liu, L. (2020). GADE: A Generative Adversarial Approach to Density Estimation and its Applications. International Journal of Computer Vision, 128(10-11), 2731-2743.
Scopus2 WoS22020 Yan, Q., Wang, B., Li, P., Li, X., Zhang, A., Shi, Q., . . . Zhang, Y. (2020). Ghost removal via channel attention in exposure fusion. Computer Vision and Image Understanding, 201, 1-8.
Scopus22 WoS172020 Dendorfer, P., Rezatofighi, H. S., Milan, A., Shi, Q. J., Cremers, D., Reid, I. D., . . . Leal-Taixé, L. (2020). MOT20: A benchmark for multi object tracking in crowded scenes. CoRR, abs/2003.09003, 7 pages. 2020 Guo, Y., Chen, J., Du, Q., Van Den Hengel, A., Shi, Q., & Tan, M. (2020). Multi-way backpropagation for training compact deep neural networks.. Neural Netw, 126, 250-261.
Scopus21 WoS15 Europe PMC32020 Liu, C., Yao, R., Rezatofighi, S. H., Reid, I., & Shi, Q. (2020). Model-Free Tracker for Multiple Objects Using Joint Appearance and Motion Inference. IEEE Transactions on Image Processing, 29, 277-288.
Scopus11 WoS82019 Guo, Y., Chen, Q., Chen, J., Wu, Q., Shi, Q., & Tan, M. (2019). Auto-Embedding Generative Adversarial Networks for High Resolution Image Synthesis. IEEE Transactions on Multimedia, 21(11), 2726-2737.
Scopus59 WoS442019 Wang, Y., Gong, D., Yang, J., Shi, Q., Hengel, A. V. D., Xie, D., & Zeng, B. (2019). An Effective Two-Branch Model-Based Deep Network for Single Image
Deraining.2019 Wang, Y., Shi, Q., Abbasnejad, E., Ma, C., Ma, X., & Zeng, B. (2019). Deep Single Image Deraining Via Estimating Transmission and Atmospheric
Light in rainy Scenes.2019 Kang, L., Liu, J., Liu, L., Shi, Q., & Ye, D. (2019). Creating Auxiliary Representations from Charge Definitions for Criminal
Charge Prediction.2019 Wang, Y., Zhang, H., Liu, Y., Shi, Q., & Zeng, B. (2019). Gradient Information Guided Deraining with A Novel Network and
Adversarial Training.2019 Liu, Y., Liu, L., Rezatofighi, H., Do, T. -T., Shi, Q., & Reid, I. (2019). Learning Pairwise Relationship for Multi-object Detection in Crowded
Scenes.2019 Liu, W., Gong, D., Tan, M., Shi, Q., Yang, Y., & Hauptmann, A. G. (2019). Learning Distilled Graph for Large-scale Social Network Data Clustering. IEEE Transactions on Knowledge and Data Engineering, 32(7), 1393-1404.
Scopus3 WoS12019 Dendorfer, P., Rezatofighi, H., Milan, A., Shi, J., Cremers, D., Reid, I., . . . Leal-Taixe, L. (2019). CVPR19 Tracking and Detection Challenge: How crowded can it get?. 2019 Zhang, L., Wei, W., Shi, Q., Shen, C., van den Hengel, A., & Zhang, Y. (2019). Accurate imagery recovery using a multi-observation patch model. Information Sciences, 501, 724-741.
Scopus12019 Yao, R., Lin, G., Shen, C., Zhang, Y., & Shi, Q. (2019). Semantics-Aware Visual Object Tracking. IEEE Transactions on Circuits and Systems for Video Technology, 29(6), 1687-1700.
Scopus28 WoS262019 Gong, D., Tan, M., Shi, Q., van den Hengel, A., & Zhang, Y. (2019). MPTV: matching pursuit based total variation minimization for image deconvolution. IEEE Transactions on Image Processing, 28(4), 1851-1865.
Scopus18 WoS15 Europe PMC12019 Suwanwimolkul, S., Zhang, L., Gong, D., Zhang, Z., Chen, C., Ranasinghe, D. C., & Qinfeng Shi, J. (2019). An adaptive markov random field for structured compressive sensing. IEEE Transactions on Image Processing, 28(3), 1556-1570.
Scopus9 WoS62019 Suwanwimolkul, S., Zhang, L., Ranasinghe, D. C., & Shi, Q. (2019). One-step adaptive Markov random field for structured compressive sensing. Signal Processing, 156, 116-144.
Scopus5 WoS22018 Yao, R., Lin, G., Shi, Q., & Ranasinghe, D. C. (2018). Efficient dense labelling of human activity sequences from wearables using fully convolutional networks. Pattern Recognition, 78, 252-266.
Scopus72 WoS542018 Zhang, L., Wei, W., Zhang, Y., Shen, C., van den Hengel, A., & Shi, Q. (2018). Cluster sparsity field: an internal hyperspectral imagery prior for reconstruction. International Journal of Computer Vision, 126(8), 797-821.
Scopus67 WoS802018 Rezatofighi, S. H., Kaskman, R., Motlagh, F. T., Shi, Q., Cremers, D., Leal-Taixé, L., & Reid, I. (2018). Deep Perm-Set Net: Learn to predict sets with unknown permutation and
cardinality using deep neural networks.2017 Zhang, L., Wei, W., Shi, Q., Shen, C., Hengel, A. V. D., & Zhang, Y. (2017). Beyond Low Rank: A Data-Adaptive Tensor Completion Method. 2017 Shinmoto Torres, R., Shi, Q., van den Hengel, A., & Ranasinghe, D. (2017). A hierarchical model for recognizing alarming states in a batteryless sensor alarm intervention for preventing falls in older people. Pervasive and Mobile Computing, 40, 1-16.
Scopus12 WoS102017 Yao, R., Shi, Q., Shen, C., Zhang, Y., & Van Den Hengel, A. (2017). Part-based robust tracking using online latent structured learning. IEEE Transactions on Circuits and Systems for Video Technology, 27(6), 1235-1248.
Scopus17 WoS132017 Abbasnejad, M., Shi, Q., Abbasnejad, I., Hengel, A., & Dick, A. (2017). Bayesian Conditional Generative Adverserial Networks.. CoRR, abs/1706.05477. 2016 Zhang, L., Wei, W., Zhang, Y., Shen, C., Van Den Hengel, A., & Shi, Q. (2016). Dictionary learning for promoting structured sparsity in hyperspectral compressive sensing. IEEE Transactions on Geoscience and Remote Sensing, 54(12), 7223-7235.
Scopus46 WoS392016 Torres, R. L. S., Ranasinghe, D. C., Shi, Q., & Hengel, A. V. D. (2016). Learning from Imbalanced Multiclass Sequential Data Streams Using
Dynamically Weighted Conditional Random Fields.2016 Guo, Y., Chen, J., Du, Q., Hengel, A. V. D., Shi, Q., & Tan, M. (2016). The Shallow End: Empowering Shallower Deep-Convolutional Networks
through Auxiliary Outputs.2015 Tan, M., Xiao, S., Gao, J., Xu, D., Hengel, A. V. D., & Shi, Q. (2015). Scalable Nuclear-norm Minimization by Subspace Pursuit Proximal
Riemannian Gradient.2015 Shi, Q., Reid, M., Caetano, T., Van Den Hengel, A., & Wang, Z. (2015). A hybrid loss for multiclass and structured prediction. IEEE Transactions on Pattern Analysis and Machine Intelligence, 37(1), 2-12.
Scopus3 WoS22015 Li, H., Shen, C., Van Den Hengel, A., & Shi, Q. (2015). Worst case linear discriminant analysis as scalable semidefinite feasibility problems. IEEE Transactions on Image Processing, 24(8), 2382-2392.
Scopus9 WoS62015 Shen, F., Shen, C., Shi, Q., Van Den Hengel, A., Tang, Z., & Shen, H. (2015). Hashing on nonlinear manifolds. IEEE Transactions on Image Processing, 24(6), 1839-1851.
Scopus139 WoS127 Europe PMC132015 Yin, M., Gao, J., Lin, Z., Shi, Q., & Guo, Y. (2015). Dual graph regularized latent low-rank representation for subspace clustering. IEEE Transactions on Image Processing, 24(12), 4918-4933.
Scopus120 WoS106 Europe PMC82014 Paisitkriangkrai, S., Shen, C., Shi, Q., & van den Hengel, A. (2014). RandomBoost: simplified multiclass boosting through randomization. IEEE Transactions on Neural Networks and Learning Systems, 25(4), 764-779.
Scopus6 WoS7 Europe PMC12013 Zhang, Z., Shi, Q., Zhang, Y., Shen, C., & Hengel, A. V. D. (2013). Constraint Reduction using Marginal Polytope Diagrams for MAP LP
Relaxations.2012 Gao, J., Shi, Q., & Caetano, T. (2012). Dimensionality reduction via compressive sensing. Pattern Recognition Letters, 33(9), 1163-1170.
Scopus30 WoS252011 Shi, Q., Li, C., Wang, L., & Smola, A. (2011). Human action segmentation and recognition using discriminative semi-Markov models. International Journal of Computer Vision, 93(1), 22-32.
Scopus109 WoS752010 Shi, Q., Reid, M. D., & Caetano, T. (2010). Conditional Random Fields and Support Vector Machines: A Hybrid Approach. 2009 Shi, Q., Petterson, J., Dror, G., Langford, J., Smola, A., & Vishwanathan, S. (2009). Hash Kernels for Structured Data. Journal of Machine Learning Research (Print), 10, 2615-2637.
Scopus151 WoS942006 Li, Y., Shi, Q. F., Zhang, Y. N., & Zhao, R. C. (2006). Automatic segmentation for synthetic aperture radar images. Dianzi Yu Xinxi Xuebao/Journal of Electronics and Information Technology, 28(5), 932-935.
Scopus3- Li, H., Shen, C., & Shi, Q. (n.d.). Real-time Visual Tracking Using Sparse Representation. -
Conference Papers
-
Conference Items
Year Citation 2018 Bennett, B., Westra, S., Cavagnaro, T., Wheeler, S., Shi, Q., & Pagay, V. (2018). Unpacking Agricultural System Complexities for Improved Outcomes: Taking Advantage of Emerging Data, Technologies and Analysis. Poster session presented at the meeting of AGU Fall Meeting. -
Preprint
Year Citation 2019 Parvaneh, A., Abbasnejad, E., Wu, Q., & Shi, J. (2019). Show, Price and Negotiate: A Hierarchical Attention Recurrent Visual Negotiator..
Grants Summary
Total research funding awarded: $19.74M
- Total Australian Research Council (ARC) funding awarded: $4.34 M
- Lead (1st) Chief Investigator (CI) (2 DPs, 1 DECRA): $1M
- Co-CI (4 LPs): $3.34M
- Other funding (RDCs, Industry, ...): ~$15.4M
ARC Grants
- ARC Discovery Project Grant 2024-2027, 2nd co-Chief Investigator (CI)
Learning to Reason in Reinforcement Learning - ARC Linkage Grant 2021-2024, 3rd Chief Investigator (CI), and Machine Learning Lead
A Machine Learning driven flow modelling of fragmented rocks in cave mining - ARC Discovery Project Grant 2016-2019, 1st Chief Investigator (CI)
Probabilistic Graphical Models For Interventional Queries - ARC Linkage Project Grant 2014-2017, 2nd CI
Sentient Buildings - ARC Discovery Project Grant 2014-2016, 1st CI
Online Learning for Large Scale Structured Data in Complex Situations - ARC Linkage Grant 2013-2016, 4th CI
Semantic change detection through large-scale learning - ARC Linkage Grant 2012-2015, 3rd CI
Scalable classification for massive datasets: randomized algorithms - ARC DECRA fellowship, 2012-2014, Sole CI
Compressive Sensing Based Probabilistic Graphical Models
University Courses
AI, DL, ISML, MBD, ...
Tutorials
Probabilistic Graphical Models
- Representation [ pdf], ACVT, UoA, April 15, 2011
- Inference [ pdf], ACVT, UoA, May 6, 2011
- Learning [ pdf], ACVT, UoA, May 27, 2011
- Sampling-based approximate inference [ pdf], ACVT, UoA, June 10, 2011
- Temporal models [ pdf], ACVT, UoA, August 12, 2011
Generalisation Bounds
- Basics [ pdf], ACVT, UoA, April 13, 2012
- VC dimensions and bounds [ pdf], ACVT, UoA, April 27, 2012
- Rademacher complexity and bounds [ pdf], ACVT, UoA, August 17, 2012
- PAC Bayesian Bounds, [ pdf], ACVT, UoA, August 31, 2012
- Regret bounds for online learning, [ pdf], ACVT, UoA, Nov. 2, 2012
Please email me if you find errors or typos in the slides.
-
Current Higher Degree by Research Supervision (University of Adelaide)
Date Role Research Topic Program Degree Type Student Load Student Name 2024 Principal Supervisor Causality on Generative Model Doctor of Philosophy Doctorate Full Time Mr Jiaxin Wang 2024 Co-Supervisor Improving Machine Learning Models on the Out-Of-Distribution Generalization Doctor of Philosophy Doctorate Full Time Miss Seyedeh Mahdieh Mirmahdi 2023 Principal Supervisor Leveraging Causality for Robust Multi-Source Domain Adaptation Doctor of Philosophy Doctorate Full Time Miss Tianjiao Jiang 2023 Principal Supervisor Causal Discovery on Videos for Scene Graph Generation Doctor of Philosophy Doctorate Full Time Mr Hamed Damirchi 2023 Principal Supervisor Domain Adaptation via Causal Representation Learning Doctor of Philosophy Doctorate Full Time Mr Yichao Cai 2022 Principal Supervisor Causality and Deep Reinforcement Learning on Financial Trading. Doctor of Philosophy Doctorate Full Time Mr Haiyao Cao 2021 Principal Supervisor Research on lightweight intelligent models based on deep learning: incorporating artificial intelligence on end devices Doctor of Philosophy Doctorate Full Time Ms Hongrong Cheng 2021 Principal Supervisor Data efficient learning Doctor of Philosophy Doctorate Full Time Mr Yuhao Lin 2021 Principal Supervisor Recognizing Individual and Collective Activity Using Videos Doctor of Philosophy Doctorate Full Time Mr Bahram Mohammadi 2020 Principal Supervisor End-To-End semi-supervised text classification with automated text augmentation and character attention Master of Philosophy Master Full Time Mr Adrian John Orenstein -
Past Higher Degree by Research Supervision (University of Adelaide)
Date Role Research Topic Program Degree Type Student Load Student Name 2020 - 2021 Principal Supervisor Connecting Machine Learning to Causal Structure Learning with Jacobian Matrix Master of Philosophy Master Full Time Mr Xiongren Chen 2019 - 2022 Principal Supervisor Towards Optimistic, Imaginative, and Harmonious Reinforcement Learning in
Single-Agent and Multi-Agent EnvironmentsDoctor of Philosophy Doctorate Full Time Mr Mahdi Kazemi Moghaddam 2019 - 2023 Principal Supervisor Machine Learning and Natural Language Processing in Stock Prediction Doctor of Philosophy Doctorate Full Time Mr Jinan Zou 2018 - 2022 Principal Supervisor Interactive Vision and Language Learning Doctor of Philosophy Doctorate Full Time Mr Amin Parvaneh 2017 - 2020 Co-Supervisor Deep Learning Methods for Human Activity Recognition using Wearables Doctor of Philosophy Doctorate Full Time Mr Alireza Abedin Varamin 2016 - 2023 Principal Supervisor Deep Learning for Multipitch Detection and Melody Extraction Doctor of Philosophy Doctorate Part Time Mr Xian Wang 2016 - 2021 Principal Supervisor Deep Learning for Image Deblurring and Reflection Removal Doctor of Philosophy Doctorate Full Time Mr Jie Yang 2015 - 2018 Principal Supervisor Adaptive Markov Random Fields for Structured Compressive Sensing Doctor of Philosophy Doctorate Full Time Miss Suwichaya Suwanwimolkul 2014 - 2019 Principal Supervisor Joint Appearance and Motion Model for Multi-class Multi-object Tracking Doctor of Philosophy Doctorate Full Time Mr Chongyu Liu 2014 - 2016 Co-Supervisor Deep Learning for Multi-label Scene Classification Master of Philosophy Master Full Time Mr Junjie Zhang 2012 - 2014 Co-Supervisor Markov Random Fields with Unknown Heterogeneous Graphs Doctor of Philosophy Doctorate Full Time Mr Zhenhua Wang
Connect With Me
External Profiles