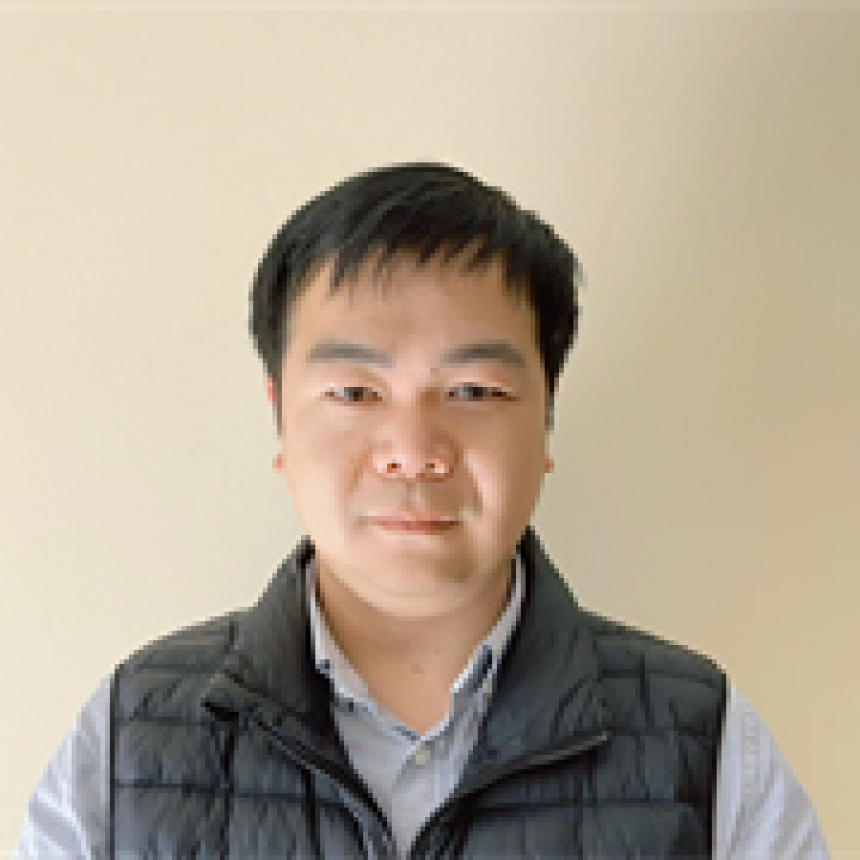
Dr Yuhang Liu
Postdoc
Australian Institute for Machine Learning - Projects
Faculty of Sciences, Engineering and Technology
Eligible to supervise Masters and PhD (as Co-Supervisor) - email supervisor to discuss availability.
My current major research topics are about:
-
building the bridge between causality and machine learning, e.g., causal representation learning, multi domain/modal Learning;
-
building the bridge between Bayesian learning and deep learning, e.g., Bayesian deep learning and deep Bayesian learning;
-
inverse problems in various applications, e.g., computer vision, signal processing.
-
Journals
Year Citation 2025 Liu, Y., Zhang, Z., Gong, D., Gong, M., Huang, B., van den Hengel, A., . . . Shi, J. Q. (2025). Latent Covariate Shift: Unlocking Partial Identifiability for Multi-Source Domain Adaptation. Transactions on Machine Learning Research, 2025-April. 2025 Shu, Y., Liu, Y., Cao, X., Chen, Q., Zhang, B., Zhou, Z., . . . Liu, L. (2025). Seeing Beyond Labels: Source-Free Domain Adaptation via Hypothesis Consolidation of Prediction Rationale. Transactions on Machine Learning Research, 2025-June. 2024 Cao, H., Zou, J., Liu, Y., Zhang, Z., Abbasnejad, E., Hengel, A. V. D., & Shi, J. Q. (2024). InvariantStock: Learning Invariant Features for Mastering the Shifting Market. Transactions on Machine Learning Research, 2024. 2024 Yan, Q., Wang, H., Ma, Y., Liu, Y., Dong, W., Woźniak, M., & Zhang, Y. (2024). Uncertainty estimation in HDR imaging with Bayesian neural networks. Pattern Recognition, 156, 110802.
Scopus452020 Wen, S., Deng, L., & Liu, Y. (2020). Distributed optimization via primal and dual decompositions for delay-constrained FANETs. Ad Hoc Networks, 109, 1-14.
Scopus152018 Liu, Y., Dong, W., & Zhou, M. (2018). Frame-Based Variational Bayesian Learning for Independent or Dependent Source Separation. IEEE Transactions on Neural Networks and Learning Systems, 29(10), 4983-4996.
Scopus132016 Dong, W. Y., Kang, L. L., Liu, Y. H., & Li, K. S. (2016). Opposition-based particle swarm optimization with adaptive elite mutation and nonlinear inertia weight. Tongxin Xuebao/Journal on Communications, 37(12), 1-10.
Scopus19 -
Conference Papers
Year Citation 2024 Liu, Y., Zhang, Z., Gong, D., Gong, M., Huang, B., van den Hengel, A., . . . Shi, J. Q. (2024). IDENTIFIABLE LATENT POLYNOMIAL CAUSAL MODELS THROUGH THE LENS OF CHANGE. In 12th International Conference on Learning Representations, ICLR 2024. Online: ICLR.
Scopus42024 Cai, Y., Liu, Y., Zhang, Z., & Shi, J. Q. (2024). CLAP: Isolating Content from Style Through Contrastive Learning with Augmented Prompts. In Lecture Notes in computer science Vol. 15079 (pp. 130-147). Milan, Italy: Springer Nature Switzerland.
DOI2022 Yan, Q., Zhang, S., Chen, W., Liu, Y., Zhang, Z., Zhang, Y., . . . Gong, D. (2022). A Lightweight Network for High Dynamic Range Imaging. In IEEE Computer Society Conference on Computer Vision and Pattern Recognition Workshops Vol. 2022-June (pp. 823-831). Online: IEEE.
DOI Scopus11 WoS12022 Perez-Pellitero, E., Catley-Chandar, S., Shaw, R., Leonardis, A., Timofte, R., Zhang, Z., . . . Park, C. Y. (2022). NTIRE 2022 Challenge on High Dynamic Range Imaging: Methods and Results. In IEEE Computer Society Conference on Computer Vision and Pattern Recognition Workshops Vol. 2022-June (pp. 1008-1022). Online: IEEE.
DOI Scopus31 WoS72022 Yan, Q., Gong, D., Liu, Y., Van Den Hengel, A., & Shi, J. Q. (2022). Learning Bayesian Sparse Networks with Full Experience Replay for Continual Learning. In Proceedings of the IEEE Computer Society Conference on Computer Vision and Pattern Recognition Vol. 2022-June (pp. 109-118). Online: IEEE.
DOI Scopus44 WoS52022 Zhang, Z., Ng, I., Gong, D., Liu, Y., Abbasnejad, E. M., Gong, M., . . . Shi, J. Q. (2022). Truncated Matrix Power Iteration for Differentiable DAG Learning. In Advances in Neural Information Processing Systems Vol. 35 (pp. 13 pages). Online: Neural information processing systems foundation.
Scopus132020 Yang, L., Liu, Y., & Fan, W. (2020). Axial Data Modeling with Collapsed Nonparametric Watson Mixture Models and Its Application to Depth Image Analysis. In Lecture Notes in Computer Science (including subseries Lecture Notes in Artificial Intelligence and Lecture Notes in Bioinformatics) Vol. 12306 LNCS (pp. 17-28). Switzerland: Springer International Publishing.
DOI Scopus12019 Liu, Y., Dong, W., Song, W., & Zhang, L. (2019). Bayesian nonnegative matrix factorization with a truncated spike-and-slab prior. In Proceedings - IEEE International Conference on Multimedia and Expo Vol. 2019-July (pp. 1450-1455). online: IEEE.
DOI Scopus22019 Liu, Y., Dong, W., Zhang, L., Gong, D., & Shi, Q. (2019). Variational bayesian dropout with a hierarchical prior. In Proceedings: 2019 IEEE/CVF Conference on Computer Vision and Pattern Recognition Vol. 2019-June (pp. 7117-7126). online: IEEE.
DOI Scopus19 WoS102018 Liu, Y., Dong, W., Gong, D., Zhang, L., & Shi, Q. (2018). Deblurring natural image using super-gaussian fields. In Proceedings of the 15th European Conference on Computer Vision as published in Lecture Notes in Computer Science Vol. 11205 LNCS (pp. 467-484). Switzerland: Springer Nature.
DOI Scopus9 WoS17
-
Current Higher Degree by Research Supervision (University of Adelaide)
Date Role Research Topic Program Degree Type Student Load Student Name 2024 Co-Supervisor Unraveling Opinion Polarization Dynamics in Social Network Echo Chambers: An Graph Modeling Approach with Causality Doctor of Philosophy Doctorate Full Time Mr Wenkang Jiang
Connect With Me
External Profiles