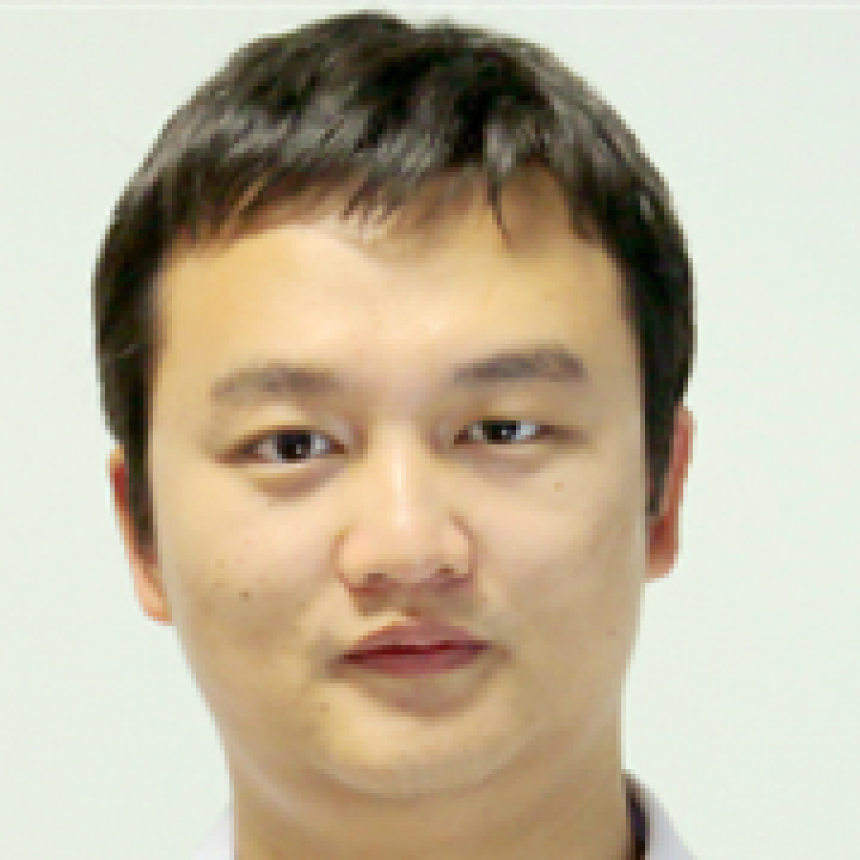
Dr Yongliang Qiao
Research Fellow
Australian Institute for Machine Learning - Projects
Faculty of Sciences, Engineering and Technology
My research focuses on agricultural artificial intelligence and robotics, which optimize agricultural production and improve the sustainability of farming practices.
My research interests include artificial intelligence, agricultural robots, smart farming, and intelligent perception. My vision is to integrate cutting-edge technologies such as artificial intelligence and robotics with agriculture to realize smart farming. The ultimate goal is to develop intelligent agricultural systems covering "information-agronomy-agricultural machinery" to support sustainable production and promote unmanned farms.
-
Appointments
Date Position Institution name 2023 - ongoing Co-chair IEEE Agricultural Robotics and Automation (AgRA) technical committee 2022 - ongoing Associate Editor Computers and Electronics in Agriculture -
Education
Date Institution name Country Title University Bourgogne Franche Comté France PhD -
Research Interests
-
Journals
Year Citation 2024 Wang, L., Zheng, Y., Jin, D., Li, F., Qiao, Y., & Pan, S. (2024). Contrastive Graph Similarity Networks. ACM Transactions on the Web, 18(2), 20 pages.
Scopus22024 Hu, N., Su, D., Wang, S., Wang, X., Zhong, H., Wang, Z., . . . Tan, Y. (2024). Segmentation and tracking of vegetable plants by exploiting vegetable shape feature for precision spray of agricultural robots. Journal of Field Robotics, 41(3), 570-586.
2024 Lin, D., Chen, Y., Qiao, Y., Qin, D., Miao, Y., Sheng, K., . . . Wang, Y. (2024). A study on an accurate modeling for distinguishing nitrogen, phosphorous and potassium status in summer maize using in situ canopy hyperspectral data. Computers and Electronics in Agriculture, 221, 14 pages.
2024 Zhang, B., & Qiao, Y. (2024). AI, Sensors, and Robotics for Smart Agriculture. Agronomy, 14(6), 3 pages.
2023 Fu, J., Chen, C., Zhao, R., Chen, Z., Li, D., & Qiao, Y. (2023). Frame vibration states identification for corn harvester based on joint improved empirical mode decomposition - Support vector machine method. Frontiers in Plant Science, 14, 15 pages.
Scopus22023 Guo, Y., Du, S., Qiao, Y., & Liang, D. (2023). Advances in the Applications of Deep Learning Technology for Livestock Smart Farming. Smart Agriculture, 5(1), 52-65.
Scopus22023 Jiang, H., Yang, X., Ding, R., Wang, D., Mao, W., & Qiao, Y. (2023). Identification of Apple Leaf Diseases Based on Improved ResNetl8. Nongye Jixie Xuebao/Transactions of the Chinese Society for Agricultural Machinery, 54(4), 295-303.
Scopus52023 Su, D., Qiao, Y., Jiang, Y., Valente, J., Zhang, Z., & He, D. (2023). Editorial: AI, sensors and robotics in plant phenotyping and precision agriculture, volume II. Frontiers in Plant Science, 14, 1215899.
Scopus1 Europe PMC12023 Wang, S., Su, D., Jiang, Y., Tan, Y., Qiao, Y., Yang, S., . . . Hu, N. (2023). Fusing Vegetation Index and Ridge Segmentation for Robust Vision Based Autonomous Navigation of Agricultural Robots in Vegetable Farms.
2023 Wang, S., Jiang, H., Qiao, Y., & Jiang, S. (2023). A Method for Obtaining 3D Point Cloud Data by Combining 2D Image Segmentation and Depth Information of Pigs. Animals, 13(15), 17 pages.
2023 Feng, T., Guo, Y., Huang, X., & Qiao, Y. (2023). Cattle Target Segmentation Method in Multi-Scenes Using Improved DeepLabV3+ Method. Animals, 13(15), 15 pages.
Scopus22023 Fu, J., Li, J., Fu, Q., & Qiao, Y. (2023). Development and verification of adhesion models for track shoes operating on clay soils. Biosystems Engineering, 235, 69-82.
Scopus12023 Guo, Y., Aggrey, S. E., Yang, X., Oladeinde, A., Qiao, Y., & Chai, L. (2023). Detecting broiler chickens on litter floor with the YOLOv5-CBAM deep learning model. Artificial Intelligence in Agriculture, 9, 36-45.
Scopus72023 Wang, S., Su, D., Jiang, Y., Tan, Y., Qiao, Y., Yang, S., . . . Hu, N. (2023). Fusing vegetation index and ridge segmentation for robust vision based autonomous navigation of agricultural robots in vegetable farms. Computers and Electronics in Agriculture, 213, 15 pages.
Scopus32023 Zhang, L., Han, G., Qiao, Y., Xu, L., Chen, L., & Tang, J. (2023). Interactive Dairy Goat Image Segmentation for Precision Livestock Farming. Animals, 13(20), 1-17.
2023 Guo, Y., Hong, W., Wu, J., Huang, X., Qiao, Y., & Kong, H. (2023). Vision-Based Cow Tracking and Feeding Monitoring for Autonomous Livestock Farming: The YOLOv5s-CA+DeepSORT-Vision Transformer. IEEE Robotics and Automation Magazine, 30(4), 68-76.
2023 Qiao, Y., Guo, Y., & He, D. (2023). Cattle body detection based on YOLOv5-ASFF for precision livestock farming. Computers and Electronics in Agriculture, 204, 9 pages.
Scopus35 WoS162023 He, C., Qiao, Y., Mao, R., Li, M., & Wang, M. (2023). Enhanced LiteHRNet based sheep weight estimation using RGB-D images. Computers and Electronics in Agriculture, 206, 1-10.
Scopus14 WoS72022 Brown, J., Qiao, Y., Clark, C., Lomax, S., Rafique, K., & Sukkarieh, S. (2022). Automated aerial animal detection when spatial resolution conditions are varied. Computers and Electronics in Agriculture, 193, 1-11.
Scopus17 WoS72022 Qiao, Y., Guo, Y., Yu, K., & He, D. (2022). C3D-ConvLSTM based cow behaviour classification using video data for precision livestock farming. Computers and Electronics in Agriculture, 193, 11 pages.
Scopus40 WoS222022 Xue, T., Qiao, Y., Kong, H., Su, D., Pan, S., Rafique, K., & Sukkarieh, S. (2022). One-Shot Learning-Based Animal Video Segmentation. IEEE Transactions on Industrial Informatics, 18(6), 3799-3807.
Scopus14 WoS122022 Wang, S., Jiang, H., Qiao, Y., Jiang, S., Lin, H., & Sun, Q. (2022). The Research Progress of Vision-Based Artificial Intelligence in Smart Pig Farming. Sensors, 22(17), 23 pages.
Scopus29 WoS11 Europe PMC102022 Liu, S., Qiao, Y., Li, J., Zhang, H., Zhang, M., & Wang, M. (2022). An Improved Lightweight Network for Real-Time Detection of Apple Leaf Diseases in Natural Scenes. Agronomy, 12(10), 17 pages.
Scopus9 WoS42022 Qiao, Y., Xue, T., Kong, H., Clark, C., Lomax, S., Rafique, K., & Sukkarieh, S. (2022). One-Shot Learning with Pseudo-Labeling for Cattle Video Segmentation in Smart Livestock Farming. Animals, 12(5), 16 pages.
Scopus5 WoS2 Europe PMC32022 Fu, J., Liu, J., Zhao, R., Chen, Z., Qiao, Y., & Li, D. (2022). Maize disease detection based on spectral recovery from RGB images. Frontiers in Plant Science, 13, 12 pages.
Scopus1 Europe PMC12022 Huang, X., Hu, Z., Qiao, Y., & Sukkarieh, S. (2022). Deep Learning-Based Cow Tail Detection and Tracking for Precision Livestock Farming. IEEE/ASME Transactions on Mechatronics, 28(3), 1213-1221.
Scopus13 WoS42022 Zhang, K., Fan, J., Huang, S., Qiao, Y., Yu, X., & Qin, F. (2022). CEKD:Cross ensemble knowledge distillation for augmented fine-grained data. Applied Intelligence, 52(14), 16640-16650.
2022 Qiao, Y., Valente, J., Su, D., Zhang, Z., & He, D. (2022). Editorial: AI, sensors and robotics in plant phenotyping and precision agriculture. Frontiers in Plant Science, 13, 5 pages.
Scopus10 Europe PMC32022 Hu, N., Wang, S., Wang, X., Cai, Y., Su, D., Nyamsuren, P., . . . Wei, H. (2022). LettuceMOT: A dataset of lettuce detection and tracking with re-identification of re-occurred plants for agricultural robots. Frontiers in Plant Science, 13, 6 pages.
Scopus2 WoS1 Europe PMC12022 Hu, N., Su, D., Wang, S., Nyamsuren, P., Qiao, Y., Jiang, Y., & Cai, Y. (2022). LettuceTrack: Detection and tracking of lettuce for robotic precision spray in agriculture. Frontiers in Plant Science, 13, 1-16.
Scopus12 Europe PMC52022 Li, J., Qiao, Y., Liu, S., Zhang, J., Yang, Z., & Wang, M. (2022). An improved YOLOv5-based vegetable disease detection method. Computers and Electronics in Agriculture, 202, 1-10.
Scopus48 WoS312022 Zhang, Z., Qiao, Y., Guo, Y., & He, D. (2022). Deep Learning Based Automatic Grape Downy Mildew Detection. Frontiers in Plant Science, 13, 1-12.
Scopus17 WoS7 Europe PMC52021 Su, D., Kong, H., Qiao, Y., & Sukkarieh, S. (2021). Data augmentation for deep learning based semantic segmentation and crop-weed classification in agricultural robotics. Computers and Electronics in Agriculture, 190, 12 pages.
Scopus63 WoS322021 Qiao, Y., Kong, H., Clark, C., Lomax, S., Su, D., Eiffert, S., & Sukkarieh, S. (2021). Intelligent perception for cattle monitoring: A review for cattle identification, body condition score evaluation, and weight estimation. Computers and Electronics in Agriculture, 185, 11 pages.
Scopus84 WoS452021 Su, D., Qiao, Y., Kong, H., & Sukkarieh, S. (2021). Real time detection of inter-row ryegrass in wheat farms using deep learning. Biosystems Engineering, 204, 198-211.
Scopus34 WoS232021 Zhang, W., Sun, X., Qiao, Y., Bai, P., Jiang, H., Wang, Y., . . . Zong, H. (2021). Tobacco disease identification based on InceptionV3. Acta Tabacaria Sinica, 27(5), 61-70.
Scopus62021 Qiao, Y., Kong, H., Clark, C., Lomax, S., Su, D., Eiffert, S., & Sukkarieh, S. (2021). Intelligent perception-based cattle lameness detection and behaviour recognition: A review. Animals, 11(11), 20 pages.
Scopus26 WoS13 Europe PMC102021 Guo, Y., Qiao, Y., Sukkarieh, S., Chai, L., & He, D. (2021). BIGRU-ATTENTION BASED COW BEHAVIOR CLASSIFICATION USING VIDEO DATA FOR PRECISION LIVESTOCK FARMING. Transactions of the ASABE, 64(6), 1823-1833.
Scopus14 WoS102021 Qiao, Y., Clark, C., Lomax, S., Kong, H., Su, D., & Sukkarieh, S. (2021). Automated Individual Cattle Identification Using Video Data: A Unified Deep Learning Architecture Approach. FRONTIERS IN ANIMAL SCIENCE, 2, 1-14.
Scopus9 WoS32020 Kong, H., Shan, M., Su, D., Qiao, Y., Al-Azzawi, A., & Sukkarieh, S. (2020). Filtering for systems subject to unknown inputs without a priori initial information. Automatica, 120, 12 pages.
Scopus17 WoS142020 Jiang, H., Zhang, C., Qiao, Y., Zhang, Z., Zhang, W., & Song, C. (2020). CNN feature based graph convolutional network for weed and crop recognition in smart farming. Computers and Electronics in Agriculture, 174, 11 pages.
Scopus150 WoS862019 Qiao, Y., Truman, M., & Sukkarieh, S. (2019). Cattle segmentation and contour extraction based on Mask R-CNN for precision livestock farming. Computers and Electronics in Agriculture, 165, 9 pages.
Scopus145 WoS942019 Qiao, Y., Cappelle, C., Ruichek, Y., & Yang, T. (2019). ConvNet and LSH-based visual localization using localized sequence matching. Sensors (Switzerland), 19(11), 23 pages.
Scopus18 WoS13 Europe PMC32017 Qiao, Y. (2017). Visual localization across seasons using sequence matching based on multi-feature combination. Sensors (Switzerland), 17(11), 22 pages.
Scopus17 WoS8 Europe PMC22017 Qiao, Y., & Zhang, Z. (2017). Visual Localization by Place Recognition Based on Multifeature (D- LBP++HOG). Journal of Sensors, 2017, 18 pages.
Scopus4 WoS22015 Li, Y., Qiao, Y., & Ruichek, Y. (2015). Multiframe-based high dynamic range monocular vision system for advanced driver assistance systems. IEEE Sensors Journal, 15(10), 5433-5441.
Scopus25 WoS172014 Qiao, Y., Cappelle, C., & Ruichek, Y. (2014). Image based place recognition and lidar validation for vehicle localization. Lecture Notes in Computer Science (including subseries Lecture Notes in Artificial Intelligence and Lecture Notes in Bioinformatics), 8856, 304-315.
Scopus12013 He, D., Qiao, Y., Li, P., Gao, Z., Li, H., & Tang, J. (2013). Weed recognition based on SVM-DS multi-feature fusion. Nongye Jixie Xuebao/Transactions of the Chinese Society for Agricultural Machinery, 44(2), 182-187.
Scopus402013 Zhao, C., He, D., & Qiao, Y. (2013). Identification method of multi-feature weed based on multi-spectral images and data mining. Nongye Gongcheng Xuebao/Transactions of the Chinese Society of Agricultural Engineering, 29(2), 192-198.
Scopus33 -
Books
Year Citation 2022 Qiao, Y., Chai, L., & He, D. (2022). Advances in Sensors, Big Data and Machine Learning in Intelligent Animal Farming. Mdpi AG. -
Conference Papers
Year Citation 2023 Qiao, Y., Guo, Y., & He, D. (2023). Deep Learning-Based Autonomous Cow Detection for Smart Livestock Farming. In Lecture Notes in Computer Science (including subseries Lecture Notes in Artificial Intelligence and Lecture Notes in Bioinformatics) Vol. 13744 LNCS (pp. 246-258). Online: Springer International Publishing.
2022 Ding, R., Qiao, Y., Yang, X., Jiang, H., Zhang, Y., Huang, Z., . . . Liu, H. (2022). Improved ResNet Based Apple Leaf Diseases Identification. In IFAC-PapersOnLine Vol. 55 (pp. 78-82). Online: ELSEVIER.
Scopus10 WoS12022 Qiao, Y., Zhang, Z., Guo, Y., & He, D. (2022). Deep learning based grape mildew disease severity classification. In 2022 ASABE Annual International Meeting. St Joseph, Michigan, USA: American Society of Agricultural and Biological Engineers.
Scopus22022 Qiao, Y., Guo, Y., He, D., & Chai, L. (2022). Deep Learning-based Autonomous cow body detection for smart livestock farming. In American Society of Agricultural and Biological Engineers (ASABE) (pp. 110-115). Houston, Texas, USA: American Society of Agricultural and Biological Engineers.
2020 Qiao, Y., Su, D., Kong, H., Sukkarieh, S., Lomax, S., & Clark, C. (2020). BiLSTM-based Individual Cattle Identification for Automated Precision Livestock Farming. In 2020 IEEE 16th International Conference on Automation Science and Engineering (CASE) Vol. 2020-August (pp. 967-972). New York, NY, USA: IEEE.
Scopus26 WoS152020 Qiao, Y., Su, D., Kong, H., Sukkarieh, S., Lomax, S., & Clark, C. (2020). Data Augmentation for Deep Learning based Cattle Segmentation in Precision Livestock Farming. In 2020 IEEE 16th International Conference on Automation Science and Engineering (CASE) Vol. 2020-August (pp. 979-984). New York, NY, USA: IEEE.
Scopus20 WoS152019 Qiao, Y., Su, D., Kong, H., Sukkarieh, S., Lomax, S., & Clark, C. (2019). Individual Cattle Identification Using a Deep Learning Based Framework. In R. Fitch, J. Katupitiya, & M. Whitty (Eds.), IFAC-PapersOnLine Vol. 52 (pp. 318-323). Amsterdam, Netherlands: ELSEVIER.
Scopus62 WoS362017 Qiao, Y., Cappelle, C., Yang, T., & Ruichek, Y. (2017). Visual localization based on place recognition using multi-feature combination (D- λ LBP++HOG). In J. BlancTalon, R. Penne, W. Philips, D. Popescu, & P. Scheunders (Eds.), Advanced Concepts for Intelligent Vision Systems. ACIVS 2017 Vol. 10617 LNCS (pp. 275-287). Antwerp, BELGIUM: SPRINGER INTERNATIONAL PUBLISHING AG.
Scopus12016 Qiao, Y., Cappelle, C., Ruichek, Y., & Dornaika, F. (2016). Visual localization based on sequence matching using ConvNet features. In IECON Proceedings (Industrial Electronics Conference) (pp. 1067-1074). ITALY, Florence: IEEE.
Scopus3 WoS12016 Qiao, Y., Cappelle, C., & Ruichek, Y. (2016). Visual localization using sequence matching based on multi-feature combination. In J. BlancTalon, C. Distante, W. Philips, D. Popescu, & P. Scheunders (Eds.), Lecture Notes in Computer Science (including subseries Lecture Notes in Artificial Intelligence and Lecture Notes in Bioinformatics) Vol. 10016 LNCS (pp. 324-335). Lecce, ITALY: SPRINGER INTERNATIONAL PUBLISHING AG.
Scopus12015 Qiao, Y., Cappelle, C., & Ruichek, Y. (2015). Place recognition based visual localization using lbp feature and SVM. In O. P. Lagunas, O. H. Alcantara, & G. A. Figueroa (Eds.), Lecture Notes in Computer Science (including subseries Lecture Notes in Artificial Intelligence and Lecture Notes in Bioinformatics) Vol. 9414 (pp. 393-404). Polytechn Univ Morelos, Cuernavaca, MEXICO: SPRINGER-VERLAG BERLIN.
Scopus6 WoS52013 Li, P., He, D., Qiao, Y., & Yang, C. (2013). An application of soft sets in weed identification. In American Society of Agricultural and Biological Engineers Annual International Meeting 2013, ASABE 2013 Vol. 5 (pp. 4279-4288). American Society of Agricultural and Biological Engineers.
Scopus4
Connect With Me
External Profiles