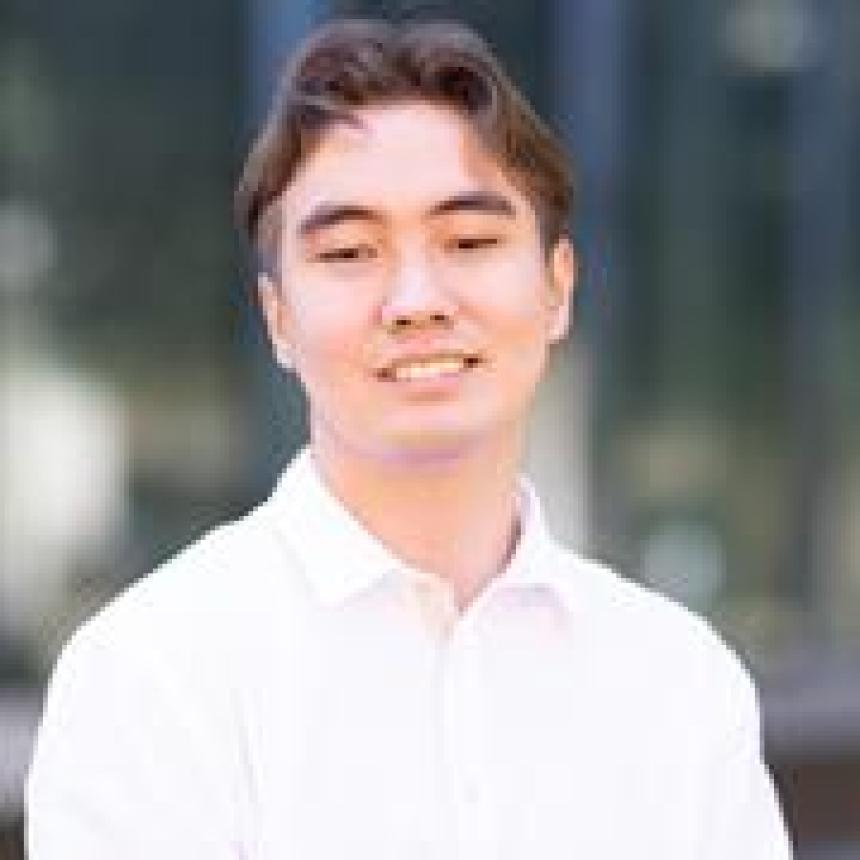
Sultan Abulkhair
Higher Degree by Research Candidate
School of Chemical Engineering
Faculty of Sciences, Engineering and Technology
Sultan is a PhD student at the ARC Training Centre for Integrated Operations for Complex Resources, University of Adelaide. He currently works on an HDR project entitled "Rapid updating of resource knowledge with sensor information including structures", which aims to use data assimilation to update geostatistical models with sensor observations.
In 2021, he obtained an MSc in Mining Engineering and graduated with honours from Nazarbayev University in Kazakhstan. From 2015 to 2019, Sultan was a student at Kazakh-British Technical University, where he received a BEng in Geology and Mineral Exploration.
Rapid, stochastic updating of resource models with sensor information for high resolution reconciliation and rapid decision-making.
This project will focus on rapidly updating the resource knowledge with upstream (drill) and downstream (belt) sensor information, with partner company Maptek. The upstream and downstream information will constrain the resource knowledge close to the mining stage and allow rapid reconciliation of the ore attributes for upstream and downstream use.
Resource models are generally constructed from directly observed data (e.g., grades of drill cores) that have relatively high accuracy. Resource models are, however, limited by the scale on which the data are collected. As mining progresses more information becomes available on different scales from various types and sources of data. Drill cuttings from blast holes provide in situ data on smaller scales. Sensors on drilling rigs and on conveyor belts provide data in near real-time as do draw-point sensing and Load Haul Dump (LHD) sensors.
All these types of data – drill core, drill sensors, draw-point sensors, LHD sensors, belt sensors – measure different volumes in different ways and have different levels of accuracy, all of which must be accounted for when integrating the various types of data. Although most data will be quantitative (e.g., grades) some will be qualitative (e.g., structures such as geology or domain types). Integrating these types of data requires a stochastic approach to account for the different levels of accuracy (uncertainty) and the different volumes of measurement. To enable rapid decision-making, the resource model must be updated with newly acquired data in near real-time.
The project will involve calibrating the various types of data, integrating/fusing the data and developing and/or adapting methods for rapidly (near real-time) updating resource models with newly acquired data. Data integration will include accounting for the different levels of uncertainty of the various types of data. Methods to be explored for updating resource models include the various forms of the Kalman filter.
-
Appointments
Date Position Institution name 2021 - ongoing PhD Candidate University of Adelaide 2019 - 2021 Research Assistant Nazarbayev University -
Awards and Achievements
-
Language Competencies
Language Competency English Can read, write, speak, understand spoken and peer review Kazakh Can read, write, speak, understand spoken and peer review Russian Can read, write, speak, understand spoken and peer review -
Education
Date Institution name Country Title 2019 - 2021 Nazarbayev University Kazakhstan Master of Science 2015 - 2019 Kazakh-British Technical University Kazakhstan Bachelor of Engineering and Technology -
Research Interests
-
Journals
-
Conference Papers
-
Conference Items
- ARC Training Centre for Integrated Operations for Complex Resources Scholarship (2021 - 2024)
- IAMG Student Travel Grant (2022)
- IAMG Student Research Grant: Mathematical Geosciences Student Award (2022)
-
Memberships
Date Role Membership Country 2022 - ongoing Member Society for Mining, Metallurgy & Exploration United States 2020 - ongoing Member International Association of Mathematical Geosciences United States -
Presentation
Date Topic Presented at Institution Country 2022 - 2022 Rapid updating of resource knowledge with sensor information South Australian Exploration & Mining Conference SAEMC Australia 2022 - 2022 Comparison of multiGaussian transforms in multivariate geostatistical simulation 21st Annual Conference of the International Association for Mathematical Geosciences IAMG France 2021 - 2021 Reproduction of inequality constraint between iron and silica for accurate production scheduling Iron Ore 2021 AusIMM Australia 2021 - 2021 Mineral resource modelling of variables with inequality constraints: a case study of an iron ore deposit APCOM 2021 SAIMM South Africa 2021 - 2021 Application of multiple-point statistics for stratigraphic modelling of coal layers 11th International Geostatistics Congress Geostats Canada
Connect With Me
External Profiles