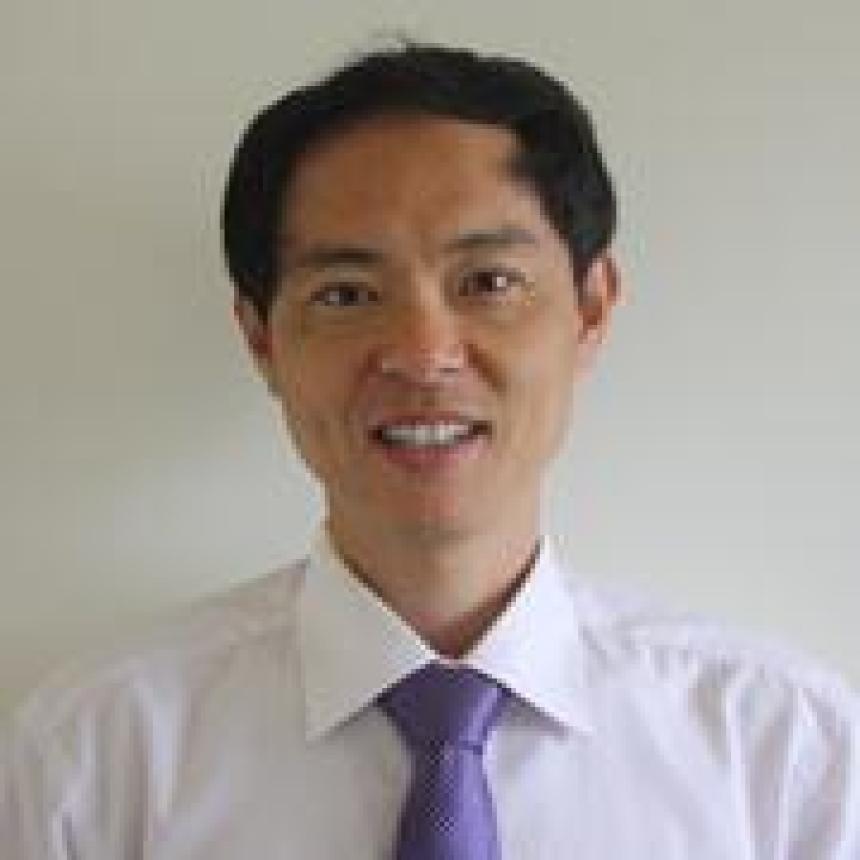
Dr Huajian Liu
Grant-Funded Researcher (B)
School of Agriculture, Food and Wine
Faculty of Sciences, Engineering and Technology
Eligible to supervise Masters and PhD - email supervisor to discuss availability.
In 2018, I finished my PhD study of machine vision for invertebrate detection on crops at the University of South Australia. In the same year, I joined The Plant Accelerator, the Adelaide node of the Australian Plant Phenomic Facility, located at the Waite campus of The University of Adelaide, as a post-doctoral researcher. Since then, I have been specialising in machine vision and machine learning for plant phenotyping and precision agriculture, especially for hyperspectral imaging-based plant phenotyping. I am currently a grant-funded researcher and my research interests include plant nutrient estimation, plant disease detection, drought and salt stress tolerance, plant growing status estimation, invertebrate pest detection, machine learning and deep learning, optical sensing system design, bio-inspired machine vision system, bird vision, insect vision, high-dimensional colour space, 3D model reconstruction and object recognition.
-
Appointments
Date Position Institution name 2018 - ongoing Postdoctoral Fellow/Grant-funded Researcher University of Adelaide -
Education
Date Institution name Country Title 2014 - 2018 University of South Australia Australia PhD -
Research Interests
-
Journals
Year Citation 2024 Xie, Y., Plett, D., Evans, M., Garrard, T., Butt, M., Clarke, K., & Liu, H. (2024). Hyperspectral imaging detects biological stress of wheat for early diagnosis of crown rot disease. Computers and Electronics in Agriculture, 217, 108571.
Scopus182024 Tzeis, S., Gerstenfeld, E. P., Kalman, J., Saad, E. B., Sepehri Shamloo, A., Andrade, J. G., . . . Trines, S. A. (2024). 2024 European Heart Rhythm Association/Heart Rhythm Society/Asia Pacific Heart Rhythm Society/Latin American Heart Rhythm Society expert consensus statement on catheter and surgical ablation of atrial fibrillation.. Europace : European pacing, arrhythmias, and cardiac electrophysiology : journal of the working groups on cardiac pacing, arrhythmias, and cardiac cellular electrophysiology of the European Society of Cardiology, 26(4), 921-1072.
Scopus241 Europe PMC752024 Liu, H., Perry, K. D., Lu, T. F., Wu, T., & Buhl, C. (2024). Machine vision solutions for monitoring pest snails in australian no-till cropping fields: An exploration of spectral characteristics and detectability. Journal of Agriculture and Food Research, 16, 12 pages.
2024 Hume, R., Marschner, P., Mason, S., Schilling, R. K., Liu, H., Jewell, N., . . . Mosley, L. M. (2024). Assessing wheat responses to soil acidification and liming using hyperspectral imaging. Plant and Soil, 19 pages.
2023 Wu, T., Dai, J., Shen, P., Liu, H., & Wei, Y. (2023). Seedscreener: A novel integrated wheat germplasm phenotyping platform based on NIR-feature detection and 3D-reconstruction. Computers and Electronics in Agriculture, 215, 108378.
Scopus22023 Liu, H., & Xu, Z. (2023). Editorial: Machine vision and machine learning for plant phenotyping and precision agriculture. Frontiers in Plant Science, 14, 1331918.
Scopus6 Europe PMC32022 Xie, Y., Plett, D., & Liu, H. (2022). Detecting Crown Rot Disease in Wheat in Controlled Environment Conditions Using Digital Color Imaging and Machine Learning. AgriEngineering, 4(1), 141-155.
Scopus10 WoS22022 Ball, K. R., Liu, H., Brien, C., Berger, B., Power, S. A., & Pendall, E. (2022). Hyperspectral imaging predicts yield and nitrogen content in grass-legume polycultures. PRECISION AGRICULTURE, 23(6), 2270-2288.
Scopus12 WoS22021 Liu, H., & Chahl, J. S. (2021). Proximal detecting invertebrate pests on crops using a deep residual convolutional neural network trained by virtual images. Artificial Intelligence in Agriculture, 5, 13-23.
Scopus25 WoS82021 Xie, Y., Plett, D., & Liu, H. (2021). The Promise of Hyperspectral Imaging for the Early Detection of Crown Rot in Wheat. AgriEngineering, 3(4), 924-941.
Scopus15 WoS42020 Liu, H., Bruning, B., Garnett, T., & Berger, B. (2020). Hyperspectral imaging and 3D technologies for plant phenotyping: From satellite to close-range sensing. Computers and Electronics in Agriculture, 175, 13 pages.
Scopus106 WoS412020 Bruning, B., Berger, B., Lewis, M., Liu, H., & Garnett, T. (2020). Approaches, applications, and future directions for hyperspectral vegetation studies: An emphasis on yield‐limiting factors in wheat. The Plant Phenome Journal, 3(1), 22 pages.
Scopus352020 Liu, H., Bruning, B., Garnett, T., & Berger, B. (2020). The performances of hyperspectral sensors for proximal sensing of nitrogen levels in wheat. Sensors (Switzerland), 20(16), 1-21.
Scopus32 WoS11 Europe PMC102019 Bruning, B., Liu, H., Brien, C., Berger, B., Lewis, M., & Garnett, T. (2019). The Development of Hyperspectral Distribution Maps to Predict the Content and Distribution of Nitrogen and Water in Wheat (Triticum aestivum).. Frontiers in plant science, 10, 1380.
Scopus64 WoS33 Europe PMC152018 Liu, H., & Chahl, J. (2018). A multispectral machine vision system for invertebrate detection on green leaves. Computers and Electronics in Agriculture, 150, 279-288.
Scopus38 WoS17 Europe PMC12018 Liu, H., Lee, S. -H., & Chahl, J. S. (2018). Registration of multispectral 3D points for plant inspection. Precision Agriculture, 19(3), 513-536.
Scopus26 WoS142017 Liu, H., Lee, S. -H., & Chahl, J. S. (2017). A review of recent sensing technologies to detect invertebrates on crops. Precision Agriculture, 18(4), 635-666.
Scopus57 WoS37 Europe PMC52017 Liu, H., Lee, S. -H., & Chahl, J. S. (2017). An evaluation of the contribution of ultraviolet in fused multispectral images for invertebrate detection on green leaves. Precision Agriculture, 18(4), 667-683.
Scopus10 WoS72017 Liu, H., Lee, S. -H., & Chahl, J. S. (2017). A multispectral 3-D vision system for invertebrate detection on crops. IEEE Sensors Journal, 17(22), 7502-7515.
Scopus33 WoS192017 Liu, H., Lee, S. -H., & Chahl, J. S. (2017). Transformation of a high-dimensional color space for material classification. Journal of the Optical Society of America A, 34(4), 523.
Scopus18 WoS15 Europe PMC32014 Liu, H., Lee, S. H., & Saunders, C. (2014). Development of a machine vision system for weed detection during both of off-season and in-season in broadacre no-tillage cropping lands. American Journal of Agricultural and Biological Sciences, 9(2), 174-193.
Scopus242013 Liu, H., Saunders, C., & Lee, S. H. (2013). Development of a proximal machine vision system for off-season weed mapping in broadacre No-Tillage fallows. Journal of Computer Science, 9(12), 1803-1821.
Scopus12 -
Conference Papers
Year Citation 2018 Chahl, J., & Liu, H. (2018). Bioinspired invertebrate pest detection on standing crops. In Bioinspiration, Biomimetics, and Bioreplication VIII Vol. 10593 (pp. 105930B-1-105930B-14). online: SPIE.
DOI Scopus3 WoS12015 Liu, H., & Lee, S. (2015). Stitching of video sequences for weed mapping. In 2015 International Conference on Intelligent Information Hiding and Multimedia Signal Processing (IIH-MSP) (pp. 441-444). Online: IEEE.
DOI Scopus4 WoS52013 Liu, H. (2013). Development of a green plant image segmentation method of machine vision system for no-tillage fallow weed detection. In innovative agricultural technologies for a sustainable futur. Mandurah, WA, Australia. -
Conference Items
Year Citation 2019 Liu, H., Bruning, B., Berger, B., & Garnett, T. (2019). Green plant segmentation in hyperspectral images using SVM and hyper-hue. Poster session presented at the meeting of Proceedings: 7th International Workshop on Image Analysis Methods for the Plant Sciences (IAMPS 2019). Lyon, France. 2018 Liu, H. (2018). Invertebrate pest detection on crops using multispectral images and 3D vision. Poster session presented at the meeting of The 5th International Plant Phenotyping Symposium. Adelaide. -
Theses
Year Citation 2018 Liu, H., Lee, S. -H., & Chahl, J. (2018). MACHINE VISION FOR DETECTION OF INVERTEBRATES ON CROPS. 2015 Liu, H., Saunder, C., & Lee, S. -H. (2015). Development of the Algorithms and Mechanism of a Machine Vision System for Both Summer and In-season Weed Mapping in Broadacre No-till Cropping Lands. -
Datasets
Year Citation - Liu, H., Berger, B., Garnett, T., & Bruning, B. (n.d.). public data for wheat_n experiment.
DOI- Liu, H. (n.d.). The Promise of Hyperspectral Imaging for the Early Detection of Crown Rot in Wheat.
DOI- Liu, H. (n.d.). Detecting Crown Rot Disease in Wheat in Controlled Environment Conditions Using Digital Color Imaging and Machine Learning.
DOI- Liu, H., Ball, K., & Brien, C. (n.d.). Hyperspectral imaging predicts yield and nitrogen content in grass-legume polyculturesem.
DOI
Dates | Roles | Grants |
---|---|---|
2023-2026 | CI | In-field high-throughput phenotyping for barley net blotch, $830,000 |
2022-2024 | CI | GRDC project (PROC-9176394) "More effective control of pest molluscs (snails and slugs) in Australian grain crops (RFT)", AUD$2, 831, 392. |
2021-2022 | CI | Research Roadmap "Improving detection and monitoring of biosecurity threats using drones, field robots and machine learning", AUD$43, 330. |
2020-2021 | Lead CI | Yitpi Foundation Awards “Hyperspectral phenotyping for the rapid identification and quantification of crown rot in wheat”, AUD$18,150. |
2019 | CI | GRDC Project (1977340) "New methods for snail control ", AUD$140,250. |
-
Current Higher Degree by Research Supervision (University of Adelaide)
Date Role Research Topic Program Degree Type Student Load Student Name 2022 Principal Supervisor Application of Imaging Technologies in Agricultural Phenotyping and Plant Phenomics Research Doctor of Philosophy Doctorate Full Time Mr Yiting Xie
Connect With Me
External Profiles