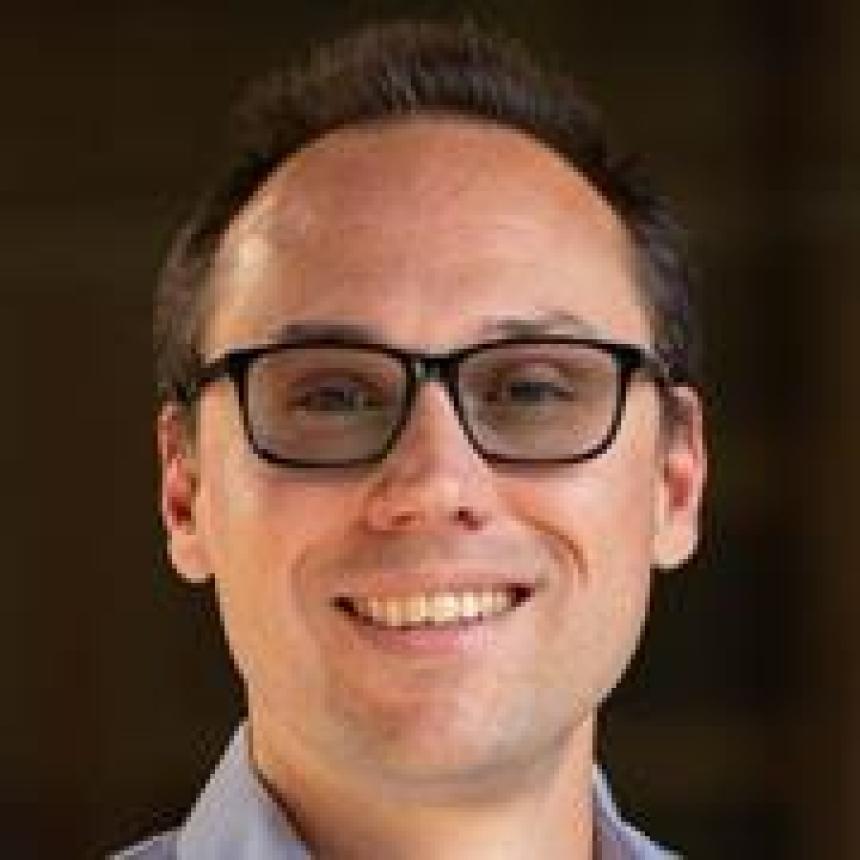
Professor Dino Sejdinovic
Professor
School of Computer and Mathematical Sciences
Faculty of Sciences, Engineering and Technology
Eligible to supervise Masters and PhD - email supervisor to discuss availability.
Dino Sejdinovic is a Professor at the School of Computer and Mathematical Sciences, University of Adelaide. He was previously a Lecturer and an Associate Professor at the Department of Statistics, University of Oxford (2014-2022). He held postdoctoral positions at the Gatsby Computational Neuroscience Unit, University College London (2011-2014) and at the Institute for Statistical Science, University of Bristol (2009-2011). He received a PhD in Electrical and Electronic Engineering from the University of Bristol (2009) and a Diplom in Mathematics and Theoretical Computer Science from the University of Sarajevo (2006).
My research spans a variety of topics at the interface between statistical methodology and machine learning, including:
- Large-scale nonparametric and kernel methods,
- Robust and trustworthy machine learning,
- Multiresolution data and data fusion,
- Measures of dependence and multivariate interaction.
-
Appointments
Date Position Institution name 2022 - ongoing Professor University of Adelaide 2016 - 2022 Associate Professor University of Oxford 2014 - 2016 Lecturer University of Oxford 2011 - 2014 Postdoctoral Fellow University College London 2009 - 2011 Brunel Postdoctoral Fellow University of Bristol -
Education
Date Institution name Country Title 2009 University of Bristol United Kingdom PhD 2006 University of Sarajevo Bosnia and Herzegovina Dipl.Math.-Inf. -
Research Interests
-
Journals
Year Citation 2025 Matabuena, M., Vidal, J. C., Padilla, O. H. M., & Sejdinovic, D. (2025). Kernel biclustering algorithm in Hilbert spaces. Advances in Data Analysis and Classification.
2025 Dan, S., Ling, Z., Chen, Y., Tegegne, J., Jaeger, V. K., Karch, A., . . . Bhatt, S. (2025). Addressing survey fatigue bias in longitudinal social contact studies to improve pandemic preparedness. Scientific Reports, 15(1), 17935.
2024 Severin, B., Lennon, D. T., Camenzind, L. C., Vigneau, F., Fedele, F., Jirovec, D., . . . Ares, N. (2024). Cross-architecture tuning of silicon and SiGe-based quantum devices using machine learning. Scientific Reports, 14(1), 17281.
Scopus2 Europe PMC12024 Lenhardt, J., Quaas, J., & Sejdinovic, D. (2024). Marine cloud base height retrieval from MODIS cloud properties using machine learning. ATMOSPHERIC MEASUREMENT TECHNIQUES, 17(18), 5655-5677.
2024 Bouabid, S., Watson-Parris, D., Stefanović, S., Nenes, A., & Sejdinovic, D. (2024). Aerosol optical depth disaggregation: toward global aerosol vertical profiles. Environmental Data Science, 3, e16-1-e16-33.
2024 Hu, R., Sejdinovic, D., & Evans, R. J. (2024). A Kernel Test for Causal Association via Noise Contrastive Backdoor Adjustment. Journal of Machine Learning Research, 25, 160-1-160-56. 2024 Craig, D. L., Moon, H., Fedele, F., Lennon, D. T., Van Straaten, B., Vigneau, F., . . . Ares, N. (2024). Bridging the Reality Gap in Quantum Devices with Physics-Aware Machine Learning. Physical Review X, 14(1).
Scopus52024 Bouabid, S., Sejdinovic, D., & Watson-Parris, D. (2024). FaIRGP: A Bayesian Energy Balance Model for Surface Temperatures Emulation. JOURNAL OF ADVANCES IN MODELING EARTH SYSTEMS, 16(6), 53 pages.
Scopus32023 Pérez-Suay, A., Gordaliza, P., Loubes, J. M., Sejdinovic, D., & Camps-Valls, G. (2023). Fair Kernel Regression through Cross-Covariance Operators. Transactions on Machine Learning Research, 2023.
Scopus12023 Hu, R., & Sejdinovic, D. (2023). Towards Deep Interpretable Features. Journal of Computational Mathematics and Data Science, 6, 100067.
Scopus12023 Chau, S. L., Cucuringu, M., & Sejdinovic, D. (2023). Spectral Ranking with Covariates. Lecture Notes in Computer Science (including subseries Lecture Notes in Artificial Intelligence and Lecture Notes in Bioinformatics), 13717 LNAI, 70-86.
Scopus22023 Li, Z., Su, W. J., & Sejdinovic, D. (2023). Benign Overfitting and Noisy Features. Journal of the American Statistical Association, 118(544), 2876-2888.
Scopus5 WoS12023 Schuff, J., Lennon, D. T., Geyer, S., Craig, D. L., Fedele, F., Vigneau, F., . . . Ares, N. (2023). Identifying Pauli spin blockade using deep learning. Quantum, 7, 1077.
Scopus22022 Wild, V. D., Hu, R., & Sejdinovic, D. (2022). Generalized Variational Inference in Function Spaces: Gaussian Measures meet Bayesian Deep Learning. Advances in Neural Information Processing Systems, 35.
Scopus92022 Hu, R., Chau, S. L., Sejdinovic, D., & Glaunès, J. A. (2022). Giga-scale Kernel Matrix-Vector Multiplication on GPU. Advances in Neural Information Processing Systems, 35.
Scopus12022 Schrab, A., Jitkrittum, W., Szabo, Z., Sejdinovic, D., & Gretton, A. (2022). Discussion of 'Multi-scale Fisher's independence test for multivariate dependence'. BIOMETRIKA, 109(3), 597-603.
Scopus12022 Hu, R., Chau, S. L., Huertas, J. F., & Sejdinovic, D. (2022). Explaining Preferences with Shapley Values. Advances in Neural Information Processing Systems, 35.
Scopus42022 Chau, S. L., Hu, R., Gonzalez, J., & Sejdinovic, D. (2022). RKHS-SHAP: Shapley Values for Kernel Methods. Advances in Neural Information Processing Systems, 35.
Scopus112022 Bouabid, S., Watson-Parris, D., Stefanović, S., Nenes, A., & Sejdinovic, D. (2022). AODisaggregation: toward global aerosol vertical profiles. 2022 Li, Z., Pérez-Suay, A., Camps-Valls, G., & Sejdinovic, D. (2022). Kernel dependence regularizers and Gaussian processes with applications to algorithmic fairness. Pattern Recognition, 132, 11 pages.
Scopus32022 Zhang, Q., Wild, V., Filippi, S., Flaxman, S., & Sejdinovic, D. (2022). Bayesian Kernel Two-Sample Testing. Journal of Computational and Graphical Statistics, 31(4), 1164-1176.
Scopus2 WoS12022 Hu, R., Nicholls, G. K., & Sejdinovic, D. (2022). Large scale tensor regression using kernels and variational inference. Machine Learning, 111(7), 2663-2713.
Scopus22022 Cortés-Andrés, J., Camps-Valls, G., Sippel, S., Székely, E., Sejdinovic, D., Diaz, E., . . . Reichstein, M. (2022). Physics-aware nonparametric regression models for Earth data analysis. Environmental Research Letters, 17(5), 054034.
Scopus11 WoS42022 Fawkes, J., Evans, R. J., & Sejdinovic, D. (2022). Selection, Ignorability and Challenges with Causal Fairness. Proceedings of Machine Learning Research, 177, 275-289.
Scopus52022 Chau, S. L., Gonzalez, J., & Sejdinovic, D. (2022). Learning Inconsistent Preferences with Gaussian Processes. INTERNATIONAL CONFERENCE ON ARTIFICIAL INTELLIGENCE AND STATISTICS, VOL 151, 151, 16 pages.
Scopus42022 Martinez-Taboada, D., & Sejdinovic, D. (2022). Sequential Decision Making on Unmatched Data using Bayesian Kernel
Embeddings.2022 Martinez-Taboada, D., & Sejdinovic, D. (2022). Bayesian Counterfactual Mean Embeddings and Off-Policy Evaluation. 2022 Matabuena, M., Vidal, J. C., Padilla, O. H. M., & Sejdinovic, D. (2022). Kernel Biclustering algorithm in Hilbert Spaces. 2021 Zhu, H., Howes, A., Eer, O. V., Rischard, M., Li, Y., Sejdinovic, D., & Flaxman, S. (2021). Aggregated Gaussian Processes with Multiresolution Earth Observation
Covariates.2021 Craig, D. L., Moon, H., Fedele, F., Lennon, D. T., Straaten, B. V., Vigneau, F., . . . Ares, N. (2021). Bridging the reality gap in quantum devices with physics-aware machine
learning.2021 Severin, B., Lennon, D. T., Camenzind, L. C., Vigneau, F., Fedele, F., Jirovec, D., . . . Ares, N. (2021). Cross-architecture Tuning of Silicon and SiGe-based Quantum Devices
Using Machine Learning.2021 Wild, V., Kanagawa, M., & Sejdinovic, D. (2021). Connections and Equivalences between the Nyström Method and Sparse
Variational Gaussian Processes.2021 Rindt, D., Sejdinovic, D., & Steinsaltz, D. (2021). Consistency of permutation tests of independence using distance covariance, HSIC and dHSIC. Stat, 10(1), 10 pages.
Scopus4 WoS22021 Bradley, V. C., Kuriwaki, S., Isakov, M., Sejdinovic, D., Meng, X. L., & Flaxman, S. (2021). Unrepresentative big surveys significantly overestimated US vaccine uptake. Nature, 600(7890), 695-700.
Scopus160 Europe PMC1002021 Fernández, T., Gretton, A., Rindt, D., & Sejdinovic, D. (2021). A Kernel Log-Rank Test of Independence for Right-Censored Data. Journal of the American Statistical Association, 118(542), 1-12.
Scopus3 WoS12021 Nguyen, V., Orbell, S. B., Lennon, D. T., Moon, H., Vigneau, F., Camenzind, L. C., . . . Ares, N. (2021). Deep reinforcement learning for efficient measurement of quantum devices. npj Quantum Information, 7(1), 9 pages.
Scopus28 WoS142021 Li, Z., Ton, J. F., Oglic, D., & Sejdinovic, D. (2021). Towards a unified analysis of random fourier features. Journal of Machine Learning Research, 22(1), 4887-4937.
Scopus42 WoS82021 Pu, X., Chau, S. L., Dong, X., & Sejdinovic, D. (2021). Kernel-Based Graph Learning from Smooth Signals: A Functional Viewpoint. IEEE Transactions on Signal and Information Processing over Networks, 7, 192-207.
Scopus17 WoS112021 Blair, G. S., Bassett, R., Bastin, L., Beevers, L., Borrajo, M. I., Brown, M., . . . Watkins, J. (2021). The Role of Digital Technologies in Responding to the Grand Challenges of the Natural Environment: The Windermere Accord. Patterns, 2(1), 100156.
Scopus6 WoS32021 Rindt, D., Sejdinovic, D., & Steinsaltz, D. (2021). A kernel- And optimal transport- based test of independence between covariates and right-censored lifetimes. International Journal of Biostatistics, 17(2), 331-348.
Scopus2 WoS1 Europe PMC12020 Van Esbroeck, N. M., Lennon, D. T., Moon, H., Nguyen, V., Vigneau, F., Camenzind, L. C., . . . Ares, N. (2020). Quantum device fine-tuning using unsupervised embedding learning. New Journal of Physics, 22(9), 9 pages.
Scopus18 WoS112020 Moon, H., Lennon, D. T., Kirkpatrick, J., van Esbroeck, N. M., Camenzind, L. C., Yu, L., . . . Ares, N. (2020). Machine learning enables completely automatic tuning of a quantum device faster than human experts. Nature Communications, 11(1), 10 pages.
Scopus57 WoS31 Europe PMC92020 Rindt, D., Sejdinovic, D., & Steinsaltz, D. (2020). Consistency of permutation tests for HSIC and dHSIC. 2020 Sejdinovic, D. (2020). Discussion of "Functional Models for Time-Varying Random Objects'' by
Dubey and Müller.2019 Watson-Parris, D., Sutherland, S., Christensen, M., Caterini, A., Sejdinovic, D., & Stier, P. (2019). Detecting anthropogenic cloud perturbations with deep learning. 2019 Briol, F. X., Oates, C. J., Girolami, M., Osborne, M. A., & Sejdinovic, D. (2019). Rejoinder: Probabilistic integration: A role in statistical computation?. Statistical Science, 34(1), 38-42.
Scopus6 WoS12019 Camps-Valls, G., Sejdinovic, D., Runge, J., & Reichstein, M. (2019). A perspective on Gaussian processes for Earth observation. National Science Review, 6(4), 616-618.
Scopus48 WoS34 Europe PMC142019 Briol, F. X., Oates, C. J., Girolami, M., Osborne, M. A., & Sejdinovic, D. (2019). Probabilistic integration: A role in statistical computation?. Statistical Science, 34(1), 1-22.
Scopus103 WoS672019 Runge, J., Nowack, P., Kretschmer, M., Flaxman, S., & Sejdinovic, D. (2019). Detecting and quantifying causal associations in large nonlinear time series datasets. Science Advances, 5(11), 15 pages.
Scopus606 WoS246 Europe PMC1122018 Ton, J. F., Flaxman, S., Sejdinovic, D., & Bhatt, S. (2018). Spatial mapping with Gaussian processes and nonstationary Fourier features. Spatial Statistics, 28, 59-78.
Scopus40 WoS26 Europe PMC82018 Zhang, Q., Filippi, S., Gretton, A., & Sejdinovic, D. (2018). Large-scale kernel methods for independence testing. Statistics and Computing, 28(1), 113-130.
Scopus75 WoS462018 Kanagawa, M., Hennig, P., Sejdinovic, D., & Sriperumbudur, B. K. (2018). Gaussian Processes and Kernel Methods: A Review on Connections and
Equivalences.2017 Flaxman, S., Teh, Y. W., & Sejdinovic, D. (2017). Poisson intensity estimation with reproducing kernels. Electronic Journal of Statistics, 11(2), 5081-5104.
Scopus15 WoS102016 Vukobratovic, D., Jakovetic, D., Skachek, V., Bajovic, D., Sejdinovic, D., Karabulut Kurt, G., . . . Fischer, I. (2016). CONDENSE: A Reconfigurable Knowledge Acquisition Architecture for Future 5G IoT. IEEE Access, 4, 3360-3378.
Scopus31 WoS172015 Kurth-Nelson, Z., Barnes, G., Sejdinovic, D., Dolan, R., & Dayan, P. (2015). Temporal structure in associative retrieval. Elife, 2015(4), 18 pages.
Scopus49 WoS40 Europe PMC462015 Strathmann, H., Sejdinovic, D., & Girolami, M. (2015). Unbiased Bayes for Big Data: Paths of Partial Posteriors. 2014 Johnson, O., Sejdinovic, D., Cruise, J., Piechocki, R., & Ganesh, A. (2014). Non-Parametric Change-Point Estimation using String Matching Algorithms. Methodology and Computing in Applied Probability, 16(4), 987-1008.
Scopus1 WoS12013 Sejdinovic, D., Sriperumbudur, B., Gretton, A., & Fukumizu, K. (2013). Equivalence of distance-based and RKHS-based statistics in hypothesis testing. Annals of Statistics, 41(5), 2263-2291.
Scopus471 WoS2952010 Sejdinović, D., Piechocki, R., Doufexi, A., & Ismail, M. (2010). Decentralised distributed fountain coding: Asymptotic analysis and design. IEEE Communications Letters, 14(1), 42-44.
Scopus18 WoS162009 Sejdinović, D., Piechocki, R. J., Doufexi, A., & Ismail, M. (2009). Fountain code design for data multicast with side information. IEEE Transactions on Wireless Communications, 8(10), 5155-5165.
Scopus19 WoS182009 Sejdinović, D., Vukobratović, D., Doufexi, A., Šenk, V., & Piechocki, R. J. (2009). Expanding window fountain codes for unequal error protection. IEEE Transactions on Communications, 57(9), 2510-2516.
Scopus155 WoS1202009 Vukobratović, D., Stankoyić, V., Sejdinović, D., Stanković, L., & Xiong, Z. (2009). Scalable video multicast using expanding window fountain codes. IEEE Transactions on Multimedia, 11(6), 1094-1104.
Scopus124 WoS105 -
Conference Papers
Year Citation 2025 Doan, B. G., Shamsi, A., Guo, X. Y., Mohammadi, A., Alinejad-Rokny, H., Sejdinovic, D., . . . Abbasnejad, E. (2025). Bayesian Low-Rank Learning (Bella): A Practical Approach to Bayesian Neural Networks. In T. Walsh, J. Shah, & Z. Kolter (Eds.), Proceedings of the AAAI Conference on Artificial Intelligence Vol. 39 (pp. 16298-16307). Philadelphia, Pennsylvania: Association for the Advancement of Artificial Intelligence (AAAI).
DOI2024 Oliveira, R., Sejdinovic, D., Howard, D., & Bonilla, E. V. (2024). Bayesian Adaptive Calibration and Optimal Design. In Advances in Neural Information Processing Systems Vol. 37. Vancouver: Neural information processing systems foundation Original language. 2024 Shimizu, E., Fukumizu, K., & Sejdinovic, D. (2024). Neural-Kernel Conditional Mean Embeddings. In Proceedings of Machine Learning Research Vol. 235 (pp. 45040-45059). Vienna: ML Research Press. 2024 Tsuchida, R., Ong, C. S., & Sejdinovic, D. (2024). Exact, Fast and Expressive Poisson Point Processes via Squared Neural Families. In Proceedings of the AAAI Conference on Artificial Intelligence Vol. 38 (pp. 20559-20566). Online: Association for the Advancement of Artificial Intelligence (AAAI).
DOI Scopus22023 Bouabid, S., Fawkes, J., & Sejdinovic, D. (2023). Returning The Favour: When Regression Benefits From Probabilistic Causal Knowledge. In Proceedings of Machine Learning Research Vol. 202 (pp. 2885-2913). Online: MLResearch Press. 2023 Chau, S. L., Muandet, K., & Sejdinovic, D. (2023). Explaining the Uncertain: Stochastic Shapley Values for Gaussian Process Models. In Proceedings of Advances in Neural Information Processing Systems (NeurIPS) Vol. 36 (pp. 27 pages). Online: Neural Information Processing Systems Foundation, Inc. (NeurIPS).
Scopus32023 Wild, V. D., Ghalebikesabi, S., Sejdinovic, D., & Knoblauch, J. (2023). A Rigorous Link between Deep Ensembles and (Variational) Bayesian Methods. In Advances in Neural Information Processing Systems (NeurIPS) Vol. 36. Online: Neural information processing systems foundation.
Scopus62023 Tsuchida, R., Ong, C. S., & Sejdinovic, D. (2023). Squared Neural Families: A New Class of Tractable Density Models. In Advances in Neural Information Processing Systems (NeurIPS) Vol. 36 (pp. 26 pages). Online: Neural information processing systems foundation.
Scopus62022 Wild, V. D., Hu, R., & Sejdinovic, D. (2022). Generalized Variational Inference in Function Spaces: Gaussian Measures meet Bayesian Deep Learning. In S. Koyejo, S. Mohamed, A. Agarwal, D. Belgrave, K. Cho, & A. Oh (Eds.), ADVANCES IN NEURAL INFORMATION PROCESSING SYSTEMS 35, NEURIPS 2022 (pp. 15 pages). Online: NEURAL INFORMATION PROCESSING SYSTEMS (NIPS). 2022 Hu, R., Chau, S. L., Huertas, J. F., & Sejdinovic, D. (2022). Explaining Preferences with Shapley Values. In S. Koyejo, S. Mohamed, A. Agarwal, D. Belgrave, K. Cho, & A. Oh (Eds.), ADVANCES IN NEURAL INFORMATION PROCESSING SYSTEMS 35 (NEURIPS 2022) (pp. 14 pages). Online: NEURAL INFORMATION PROCESSING SYSTEMS (NIPS). 2022 Hu, R., Chau, S. L., Sejdinovic, D., & Glaunes, J. A. (2022). Giga-scale Kernel Matrix-Vector Multiplication on GPU. In S. Koyejo, S. Mohamed, A. Agarwal, D. Belgrave, K. Cho, & A. Oh (Eds.), ADVANCES IN NEURAL INFORMATION PROCESSING SYSTEMS 35 (NEURIPS 2022) (pp. 13 pages). Online: NEURAL INFORMATION PROCESSING SYSTEMS (NIPS). 2022 Rindt, D., Hu, R., Steinsaltz, D., & Sejdinovic, D. (2022). Survival Regression with Proper Scoring Rules and Monotonic Neural Networks. In G. Camps-Valls, F. J. R. Ruiz, & I. Valera (Eds.), Proceedings of The 25th International Conference on Artificial Intelligence and Statistics Vol. 151 (pp. 1190-1205). Virtual Conference: JMLR-JOURNAL MACHINE LEARNING RESEARCH.
Scopus212022 Chau, S. L., Hu, R., Gonzalez, J., & Sejdinovic, D. (2022). RKHS-SHAP: Shapley Values for Kernel Methods. In S. Koyejo, S. Mohamed, A. Agarwal, D. Belgrave, K. Cho, & A. Oh (Eds.), ADVANCES IN NEURAL INFORMATION PROCESSING SYSTEMS 35 (NEURIPS 2022) (pp. 14 pages). Online: NEURAL INFORMATION PROCESSING SYSTEMS (NIPS). 2021 Ton, J. -F., Chan, L., Teh, Y. W., & Sejdinovic, D. (2021). Noise Contrastive Meta-Learning for Conditional Density Estimation using Kernel Mean Embeddings. In A. Banerjee, & K. Fukumizu (Eds.), 24TH INTERNATIONAL CONFERENCE ON ARTIFICIAL INTELLIGENCE AND STATISTICS (AISTATS) Vol. 130 (pp. 11 pages). Online virtual: PMLR Publisher.
Scopus82021 Chau, S. L., Bouabid, S., & Sejdinovic, D. (2021). Deconditional Downscaling with Gaussian Processes. In M. Ranzato (Ed.), Advances in Neural Information Processing Systems Vol. 22 (pp. 17813-17825). Online conference, Canada: Neural Information Processing Systems Foundation, Inc. (NeurIPS).
Scopus92021 Chau, S. L., Ton, J. F., González, J., Teh, Y. W., & Sejdinovic, D. (2021). BAYESIMP: Uncertainty Quantification for Causal Data Fusion. In M. Ranzato, A. Beygelzimer, Y. Dauphin, P. S. Liang, & J. Wortman Vaughan (Eds.), Proceedings of Advances in Neural Information Processing Systems 34 Vol. 5 (pp. 3466-3477). Online: NEURAL INFORMATION PROCESSING SYSTEMS (NIPS).
Scopus82021 Ton, J. F., Sejdinovic, D., & Fukumizu, K. (2021). Meta Learning for Causal Direction. In 35th AAAI Conference on Artificial Intelligence, AAAI 2021 Vol. 11B (pp. 9897-9905). Online: AAAI.
DOI Scopus122021 Caterini, A., Cornish, R., Sejdinovic, D., & Doucet, A. (2021). Variational Inference with Continuously-Indexed Normalizing Flows. In C. de Campos, & M. H. Maathuis (Eds.), Proceedings of Machine Learning Research Vol. 161 (pp. 44-53). online: AUAI Press.
Scopus92020 Rudner, T. G. J., Sejdinovic, D., & Gal, Y. (2020). Inter-domain deep gaussian processes. In Proceedings of the 37th International Conference on Machine Learning, ICML 2020 Vol. PartF168147-11 (pp. 8256-8264). Vienna, Austria: Journal of Machine Learning Research.
Scopus12020 Raj, A., Law, H. C. L., Sejdinovic, D., & Park, M. (2020). A Differentially Private Kernel Two-Sample Test. In Lecture Notes in Computer Science (including subseries Lecture Notes in Artificial Intelligence and Lecture Notes in Bioinformatics) Vol. 11906 LNAI (pp. 697-724). Online: Springer International Publishing.
DOI Scopus3 WoS12019 Law, H. C. L., Zhao, P., Chan, L., Huang, J., & Sejdinovic, D. (2019). Hyperparameter learning via distributional transfer. In H. Wallach, H. Larochelle, A. Beygelzimer, F. d'Alche-Buc, E. Fox, & R. Garnett (Eds.), Advances in Neural Information Processing Systems 32 (NeurIPS 2019) Vol. 32 (pp. 23 pages). Washington, D.C., USA: American Chemical Society (ACS).
Scopus14 WoS12019 Li, Z., Ton, J. F., Oglic, D., & Sejdinovic, D. (2019). Towards a unified analysis of random Fourier features. In K. Chaudhuri, & R. Salakhutdinov (Eds.), 36th International Conference on Machine Learning, ICML 2019 Vol. 2019-June (pp. 6916-6936). Long Beach, California, USA: Curran Associates, Inc.
Scopus162019 Mitrovic, J., Sejdinovic, D., & Teh, Y. W. (2019). Causal inference via kernel deviance measures. In Advances in Neural Information Processing Systems Vol. 2018-December (pp. 6986-6994). Montreal, Canada: Curran Associates, Inc.
Scopus29 WoS92018 Leon Law, H. C., Sejdinovic, D., Cameron, E., Lucas, T. C. D., Flaxman, S., Battle, K., & Fukumizu, K. (2018). Variational learning on aggregate outputs with Gaussian processes. In S. Bengio, H. Wallach, H. Larochelle, K. Grauman, N. Cesa-Bianchi, & R. Garnett (Eds.), Advances in Neural Information Processing Systems Vol. 31 (pp. 6081-6091). San Francisco. CA., USA.: Association for Computing Machinery.
Scopus18 WoS12018 Caterini, A. L., Doucet, A., Sejdinovic, D., Bengio, S., Wallach, H., Larochelle, H., . . . Garnett, R. (2018). Hamiltonian variational auto-encoder. In Advances in Neural Information Processing Systems Vol. 2018-December (pp. 8167-8177). Cambridge, Massachusetts, USA: MIT Press.
Scopus47 WoS182018 Law, H. C. L., Sutherland, D. J., Sejdinovic, D., & Flaxman, S. (2018). Bayesian approaches to distribution regression. In S. Fernando, & F. Perez-Cruz (Eds.), Proceedings of the Twenty-First International Conference on Artificial Intelligence and Statistics Vol. 84 (pp. 1167-1176). Lanzarote, Canary Islands: PMLR.
Scopus142017 Law, H. C. L., Yau, C., & Sejdinovic, D. (2017). Testing and learning on distributions with symmetric noise invariance. In Advances in Neural Information Processing Systems Vol. 2017-December (pp. 1344-1354). Long Beach, CA, USA: Curran Associattes.
Scopus42017 Mitrovic, J., Sejdinovic, D., & Teh, Y. W. (2017). Deep kernel machines via the kernel reparametrization trick. In 5th International Conference on Learning Representations, ICLR 2017 - Workshop Track Proceedings (pp. 4 pages). Toulon, France: Open Review.
Scopus12017 Schuster, I., Strathmann, H., Paige, B., & Sejdinovic, D. (2017). Kernel Sequential Monte Carlo. In Machine learning and Knowledge Discovery in Databases Vol. 10534 (pp. 390-409). Skopje, Macedonia: Springer International Publishing.
DOI Scopus4 WoS32017 Zhang, Q., Filippi, S., Flaxman, S., & Sejdinovic, D. (2017). Feature-to-feature regression for a two-step conditional independence test. In Uncertainty in Artificial Intelligence - Proceedings of the 33rd Conference, UAI 2017 (pp. 10 pages). Sydney, Aust: Proceedings of Machine Learning Research (PMLR).
Scopus13 WoS62017 Flaxman, S., Teh, Y. W., & Sejdinovic, D. (2017). Poisson intensity estimation with reproducing kernels. In A. Singh, & J. Zhu (Eds.), ARTIFICIAL INTELLIGENCE AND STATISTICS Vol. 54 (pp. 270-279). Fort Lauderdale, FL: MICROTOME PUBLISHING.
WoS82016 Park, M., Jitkrittum, W., & Sejdinovic, D. (2016). K2-ABC: Approximate bayesian computation with kernel embeddings. In A. Gretton, & C. C. Robert (Eds.), Proceedings of the 19th International Conference on Artificial Intelligence and Statistics, AISTATS 2016 Vol. 51 (pp. 398-407). Cadiz, Spain: MLR Press.
Scopus59 WoS302016 Franchi, G., Angulo, J., & Sejdinovic, D. (2016). Hyperspectral image classification with support vector machines on kernel distribution embeddings. In Proceedings International Conference on Image Processing Icip Vol. 2016-August (pp. 1898-1902). AZ, Phoenix: IEEE.
DOI Scopus6 WoS62016 Paige, B., Sejdinovic, D., & Wood, F. (2016). Super-sampling with a reservoir. In 32nd Conference on Uncertainty in Artificial Intelligence 2016 Uai 2016 (pp. 567-576).
Scopus42016 Flaxman, S., Sejdinovic, D., Cunningham, J. P., & Filippi, S. (2016). Bayesian learning of kernel embeddings. In 32nd Conference on Uncertainty in Artificial Intelligence 2016 Uai 2016 (pp. 182-191).
Scopus192016 Mitrovic, J., Sejdinovic, D., & Teh, Y. W. (2016). DR-ABC: Approximate Bayesian computation with kernel-based distribution regression. In Proceedings of Machine Learning Research Vol. 48 (pp. 2209-2218). New York City, NY, USA: MLR Press.
Scopus12 WoS142016 Vukobratovic, D., Jakovetic, D., Skachek, V., Bajovic, D., & Sejdinovic, D. (2016). Network function computation as a service in future 5G machine type communications. In 2016 9th International Symposium on Turbo Codes and Iterative Information Processing (ISTC) Vol. 2016-October (pp. 365-369). Brest, France: IEEE.
DOI Scopus32015 Jitkrittum, W., Gretton, A., Heess, N., Eslami, S. M. A., Lakshminarayanan, B., Sejdinovic, D., & Szabó, Z. (2015). Kernel-based just-in-time learning for passing expectation propagation messages. In M. Meila, & T. Heskes (Eds.), Uncertainty in Artificial Intelligence Proceedings of the 31st Conference Uai 2015 (pp. 405-414). NETHERLANDS, Amsterdam: AUAI PRESS.
Scopus17 WoS82015 Chwialkowski, K., Ramdas, A., Sejdinovic, D., & Gretton, A. (2015). Fast two-sample testing with analytic representations of probability measures. In Advances in Neural Information Processing Systems Vol. 2015-January (pp. 1981-1989).
Scopus1042015 Strathmann, H., Sejdinovic, D., Livingstone, S., Szabo, Z., & Gretton, A. (2015). Gradient-free Hamiltonian Monte Carlo with efficient Kernel exponential families. In Advances in Neural Information Processing Systems Vol. 2015-January (pp. 955-963).
Scopus462015 Vukobratovic, D., Sejdinovic, D., & Pizurica, A. (2015). Compressed Sensing using sparse binary measurements: A rateless coding perspective. In IEEE Workshop on Signal Processing Advances in Wireless Communications Spawc Vol. 2015-August (pp. 86-90). Stockholm, SWEDEN: IEEE.
DOI2014 Chwialkowski, K., Sejdinovic, D., & Gretton, A. (2014). A wild bootstrap for degenerate kernel tests. In Advances in Neural Information Processing Systems Vol. 4 (pp. 3608-3616).
Scopus412014 Sejdinovic, D., Strathmann, H., Garcia, M. L., Andrieu, C., & Gretton, A. (2014). Kernel Adaptive Metropolis-Hastings. In 31st International Conference on Machine Learning, ICML 2014 Vol. 32 (pp. 1665-1673). Bejing, PEOPLES R CHINA: JMLR-JOURNAL MACHINE LEARNING RESEARCH.
Scopus10 WoS122013 Sejdinovic, D., Gretton, A., & Bergsma, W. (2013). A kernel test for three-variable interactions. In Advances in Neural Information Processing Systems.
Scopus282012 Gretton, A., Sriperumbudur, B., Sejdinovic, D., Strathmann, H., Balakrishnan, S., Pontil, M., & Fukumizu, K. (2012). Optimal kernel choice for large-scale two-sample tests. In Advances in Neural Information Processing Systems Vol. 2 (pp. 1205-1213).
Scopus4842012 Piechocki, R. J., & Sejdinovic, D. (2012). Combinatorial channel signature modulation for wireless ad-hoc networks. In IEEE International Conference on Communications (pp. 4684-4689). IEEE.
DOI Scopus12012 Müller, A., Sejdinovic, D., & Piechocki, R. (2012). Approximate message passing under finite alphabet constraints. In ICASSP IEEE International Conference on Acoustics Speech and Signal Processing Proceedings (pp. 3177-3180). Kyoto, JAPAN: IEEE.
DOI Scopus3 WoS32012 Sejdinovic, D., Gretton, A., Sriperumbudur, B., & Fukumizu, K. (2012). Hypothesis testing using pairwise distances and associated kernels. In Proceedings of the 29th International Conference on Machine Learning Icml 2012 Vol. 2 (pp. 1111-1118).
Scopus172010 Sejdinovic, D., & Johnson, O. (2010). Note on noisy group testing: Asymptotic bounds and belief propagation reconstruction. In 2010 48th Annual Allerton Conference on Communication Control and Computing Allerton 2010 (pp. 998-1003). IEEE.
DOI Scopus712010 Sejdinović, D., Andrieu, C., & Piechocki, R. (2010). Bayesian sequential compressed sensing in sparse dynamical systems. In 2010 48th Annual Allerton Conference on Communication Control and Computing Allerton 2010 (pp. 1730-1736). IEEE.
DOI Scopus262009 Sejdinović, D., Piechocki, R. J., & Doufexi, A. (2009). AND-OR tree analysis of distributed LT codes. In Proceedings 2009 IEEE Information Theory Workshop on Networking and Information Theory Itw 2009 (pp. 261-265). Volos, GREECE: IEEE.
DOI Scopus62 WoS392009 Vukobratovic, D., Stankovic, V., Stankovic, L., & Sejdinovic, D. (2009). Precoded EWF codes for unequal error protection of scalable video. In Proceedings of the 5th International Conference on Mobile Multimedia Communications Mobimedia 2009. ICST.
DOI Scopus62009 Sejdinović, D., Piechocki, R. J., & Doufexi, A. (2009). Rateless distributed source code design. In Proceedings of the 5th International Conference on Mobile Multimedia Communications Mobimedia 2009. ICST.
DOI Scopus32008 Sejdinović, D., Piechocki, R. J., Doufexi, A., & Ismail, M. (2008). Rate adaptive binary erasure quantization with dual fountain codes. In Globecom IEEE Global Telecommunications Conference (pp. 1203-1207). New Orleans, LA: IEEE.
DOI Scopus1 WoS12008 Sejdinović, D., Ponnampalam, V., Piechocki, R. J., & Doufexi, A. (2008). The throughput analysis of different IR-HARQ schemes based on fountain codes. In IEEE Wireless Communications and Networking Conference Wcnc (pp. 267-272). Las Vegas, NV: IEEE.
DOI Scopus5 WoS32008 Sejdinović, D., Piechocki, R. J., Doufexi, A., & Ismail, M. (2008). Fountain coding with decoder side information. In IEEE International Conference on Communications (pp. 4477-4482). Beijing, PEOPLES R CHINA: IEEE.
DOI Scopus42008 Vukobratovic, D., Stankovic, V., Sejdinovic, D., Stankovic, L., & Xiong, Z. (2008). Expanding Window Fountain codes for scalable video multicast. In 2008 IEEE International Conference on Multimedia and Expo Icme 2008 Proceedings (pp. 77-80). Hannover, GERMANY: IEEE.
DOI Scopus19 WoS62007 Sejdinović, D., Vukobratović, D., Doufexi, A., Šenk, V., & Piechocki, R. J. (2007). Expanding Window Fountain codes for unequal error protection. In M. B. Matthews (Ed.), Conference Record Asilomar Conference on Signals Systems and Computers (pp. 1020-1024). Pacific Grove, CA: IEEE.
DOI Scopus34 WoS132007 Vukobratović, D., Stanković, V., Sejdinović, D., Fagoonee, L., & Xiong, Z. (2007). Scalable bata multicast using expanding window fountain codes. In 45th Annual Allerton Conference on Communication Control and Computing 2007 Vol. 1 (pp. 344-351).
Scopus7 -
Preprint
Year Citation 2025 Wild, V., Wu, J., Sejdinovic, D., & Knoblauch, J. (2025). Near-Optimal Approximations for Bayesian Inference in Function Space. 2025 Moskvichev, P., & Sejdinovic, D. (2025). All Models Are Miscalibrated, But Some Less So: Comparing Calibration
with Conditional Mean Operators.2024 Fawkes, J., Hu, R., Evans, R. J., & Sejdinovic, D. (2024). Doubly Robust Kernel Statistics for Testing Distributional Treatment Effects.
Scopus12024 Sejdinovic, D. (2024). An Overview of Causal Inference using Kernel Embeddings. 2024 Doan, B. G., Shamsi, A., Guo, X. -Y., Mohammadi, A., Alinejad-Rokny, H., Sejdinovic, D., . . . Abbasnejad, E. (2024). Bayesian Low-Rank LeArning (Bella): A Practical Approach to Bayesian
Neural Networks.2022 Fawkes, J., Hu, R., Evans, R. J., & Sejdinovic, D. (2022). Doubly Robust Kernel Statistics for Testing Distributional Treatment
Effects.2021 Hu, R., Sejdinovic, D., & Evans, R. J. (2021). A Kernel Test for Causal Association via Noise Contrastive Backdoor
Adjustment.
-
Current Higher Degree by Research Supervision (University of Adelaide)
Date Role Research Topic Program Degree Type Student Load Student Name 2025 Principal Supervisor Function Space Variational Deep Learning Master of Philosophy Master Full Time Mr Rahul Rajendra Kumar 2024 Principal Supervisor Uncertainty Calibration of Probabilistic Machine Learning Models Master of Philosophy Master Full Time Mr Peter Moskvichev 2024 Principal Supervisor Multi-Agent Machine Learning for Object-Tracking Doctor of Philosophy Doctorate Full Time Mr Vinh Thanh Nguyen 2023 Principal Supervisor Kalman Filtering and Optimization Methods for Object Tracking Master of Philosophy Master Full Time Miss Vivienne Mei-Larn Niejalke -
Other Supervision Activities
Date Role Research Topic Location Program Supervision Type Student Load Student Name 2020 - ongoing Principal Supervisor Uncertainty quantification in large scale machine learning University of Oxford DPhil Doctorate Full Time Veit David Wild 2020 - 2024 Principal Supervisor Transforming kernel-based learners to incorporate domain knowledge from climate science University of Oxford DPhil Doctorate Full Time Shahine Bouabid 2020 - ongoing Co-Supervisor On the Achievability of Causal Fairness University of Oxford DPhil Doctorate Full Time Jake Fawkes 2019 - 2024 Principal Supervisor Quantifying and mitigating selection bias in probability and nonprobability samples University of Oxford DPhil Doctorate Full Time Valerie C. Bradley
Connect With Me
External Profiles