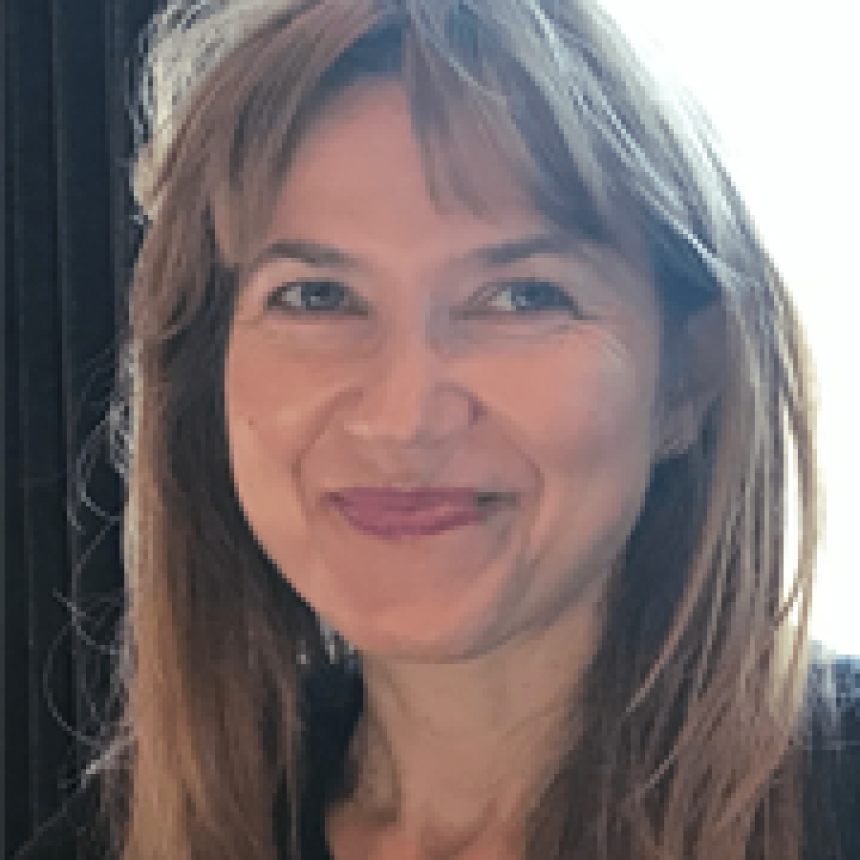
Dr Aneta Neumann
Researcher
School of Computer and Mathematical Sciences
Faculty of Sciences, Engineering and Technology
Eligible to supervise Masters and PhD - email supervisor to discuss availability.
Aneta Neumann is a researcher in the School of Computer and Mathematical Sciences at the University of Adelaide, Australia, and focuses on real world problems using evolutionary computation and machine learning methods. She is also part of the Integrated Mining Consortium at the University of Adelaide. Aneta graduated in Computer Science from the Christian-Albrechts-University of Kiel, Germany, and received her PhD from the University of Adelaide, Australia. Aneta is a co-designer and co-lecturer for the EdX Big Data Fundamentals course in the Big Data MicroMasters® program. She received an ACM-W scholarship, sponsored by Google, Microsoft, and Oracle, a Hans-Juergen and Marianna Ohff Research Grant, and the Best Paper Nominations at GECCO 2019, GECCO 2021, GECCO 2022, and the Best Paper Award at GECCO 2024.
She is co-organiser of the Workshop on AI-based Optimisation 2025 and a publicity co-chair for EMO 2025. She served as the co-chair of the Real-World Applications track at GECCO 2022-21, and is a co-chair of the Genetic Algorithms track at GECCO 2024-23. Her main research interests are bio-inspired computation methods, evolutionary diversity optimisation with a particular focus on dynamic and stochastic multi-objective optimization for real-world problems that occur in the mining and renewable energy industry, defence, cybersecurity, and public health. Moreover, her work contributes to understanding the fundamental link between bio-inspired computation, machine learning, and computational creativity. She investigates evolutionary image transition and animation in the area of Artificial Intelligence and examines how to develop designs and applications of artificial intelligent methods based on complex agent-based models.
- My Research
- Career
- Publications
- Grants and Funding
- Teaching
- Supervision
- Professional Activities
- Contact
Project title: Artificial Intelligence - Innovative approaches for increasing the productivity of South Australia’s copper and gold production. Project description: Artificial Intelligence is currently used in various ways to solve significant industry challenges. In this project, you will develop advanced AI technologies to help boost South Australia's copper and gold production. The project will cover a wide range of topics, including experimental investigations of algorithms and data analysis using machine learning techniques. The projects can be tailored to fit the background and interests of the students. The objective is to develop innovative solutions that can increase efficiency and productivity in the mining industry, ultimately leading to higher yields and profitability.
Project title: AI-based Time Use Optimization for Improving Health Outcomes. Optimising how you allocate your time can greatly impact your health and well-being. This project aims to design AI-based methods to promote health and well-being by optimising time usage. Using data from a large population-based cohort, you will optimize health outcomes for viable time plans with varying day structures. To achieve this, you will study how to to optimise multiple health outcomes simultaneously in the form of a multi-objective optimisation problem and determine trade-offs achievable with respect to different health outcomes. The projects can be carried out dependent on the background and interest of the students.
Project title: Developing an Energy Network Optimization Model for Hydrogen Networks Efficient energy network optimization model that enables the integration of clean and sustainable energy sources is important for achieving net-zero energy supply in Australia. This project will focus on developing an optimization model for integrated electrification and hydrogen networks in energy infrastructure. The student will investigate existing models, design and implement optimization algorithms for the energy network model to minimize costs for hydrogen hubs in Australia. The project requires a strong programming background, and knowledge of algorithms and optimization methods for solving real-world problems.
Project title: Advanced Ore Mine Optimisation under Uncertainty. Mining processes involve many uncertainties, mainly due to limited information about ore grades within the ore body. Using average models or an average gold/copper price can lead to weaknesses/limitations in the models, as they do not take into account the effect of potentially large losses due to deviations from expected values. In order to improve the estimation of a mining project’s value, you will model the geological uncertainties and establish confidence intervals for optimised solutions. The topic covers a wide range of areas, from experimental investigations of algorithms for ore grade estimation and mine design optimisation to data analysis using machine learning techniques such as deep neural networks. The project is suitable for a student interested both in a career in research or in industry.
Project title: Evolutionary Diversity Optimisation. Project description: Diversity optimisation is beneficial to many industrial application areas as it provides a large variety of high quality and innovative design choices. Diversity can drive innovation and deliver promising results in complex problems and optimisation. You will design and analyse algorithms for computing a diverse set of solutions that all meet given quality criteria, and explore the impact of different diversity strategies. A background on algorithms and programming knowledge is beneficial. The project is suitable for a student interested both in a career in research or in industry. We are able to offer financial assistance for undergraduate students at the UofA.
Project title: Towards Solving Real-World Optimization Problems: AI-based methods for the Traveling Thief Problem. In real-world optimization, it is common to face several sub-problems interacting and forming the main problem. There is an inter-dependency between the sub-problems, making it impossible to solve such a problem by focusing on only one component. The traveling thief problem (TTP) belongs to this category and is formed by the integration of the traveling salesperson problem (TSP) and the knapsack problem (KP). In this project, you will investigate a prominent multi-component optimisation problem, namely TTP, in the context of AI-based optimization. Moreover, we will examine the inter-dependency among the components of the problem and empirically determine the best method to solve this real-world problem. You will conduct an experimental investigation to examine the novel algorithms and compare the results to another recently introduced framework. The project is suitable for a student interested both in a career in research or in industry. The projects can be carried out dependent on the background and interest of the students.
Excellent and self-motivated students who are interested in this research topics and related areas are very welcome to contact me: aneta.neumann@adelaide.edu.au. 非常欢迎对我的研究主题感兴趣的学生与我联系
Co-organiser of the 2025 Workshop on AI-based Optimisation
(AI-OPT 2025) on 7-8 August 2025 at the University of Melbourne, Melbourne Connect. [ABSTRACT SUBMISSION], deadline: 15 May 2025.
Paper accepted at IJCAI 2025:
D. C. Dang, A. Neumann, F. Neumann, A. Opris, D. Sudholt: Theoretical Analysis of Evolutionary Algorithms with Quality Diversity for a Classical Path Planning Problem
6 Papers accepted at GECCO 2025:
- M. Xu, F. Neumann, A. Neumann, Y. Soon Ong: Quality Diversity Genetic Programming for Learning Scheduling Heuristics.
- J. Lengler, A. Neumann, F. Neumann: Runtime Analysis of Evolutionary Multitasking for Classical Benchmark Problems.
- S. Sadeghi Ahouei, A. Neumann, F. Neumann: Evolving Diverse Differentiating Stochastic Constraints Using Multi-objective Indicators.
- S. Pan, Y. J. Patel, A. Neumann, F. Neumann, T. Baeck: Evolving Hard Maximum Cut Instances for Quantum Approximate Optimization Algorithms. [arXiv]
- T. Pathirage Don, A. Neumann, F. Neumann: Evolutionary Multitasking for the Scenario-based Travelling Thief Problem.
- L. Wigney, A. Neumann, Y. Soon Ong , F. Neumann: On the Use of Matching Algorithms to Transfer Solutions for the Travelling Salesperson Problem.
Paper accepted at CEC 2025:
T. Pathirage Don, A. Neumann, F. Neumann (2025): Weighted-Scenario Optimisation for the Chance Constrained Traveling Thief Problem. In: IEEE Congress on Evolutionary Computation, IEEE Press
Paper accepted at EvoCOP 2025:
S. S. Ahouei, D. Antipov, A. Neumann, F. Neumann (2025): Feature-Based Evolutionary Diversity Optimization of Discriminating Instances for Chance-Constrained Optimization Problems. In: The 25th European Conference on Evolutionary Computation in Combinatorial Optimisation, EvoCOP 2025. [arXiv][EvoCOP]
Invited as a publicity co-chair for the International Conference on Evolutionary Multi-Criterion Optimization (EMO 2025), and to present a tutorial on Evolutionary Multi-Objective Algorithms for Constrained Single-Objective Combinatorial Optimization Problems: Theory and Applications
Space Optimization competition (SPoC3)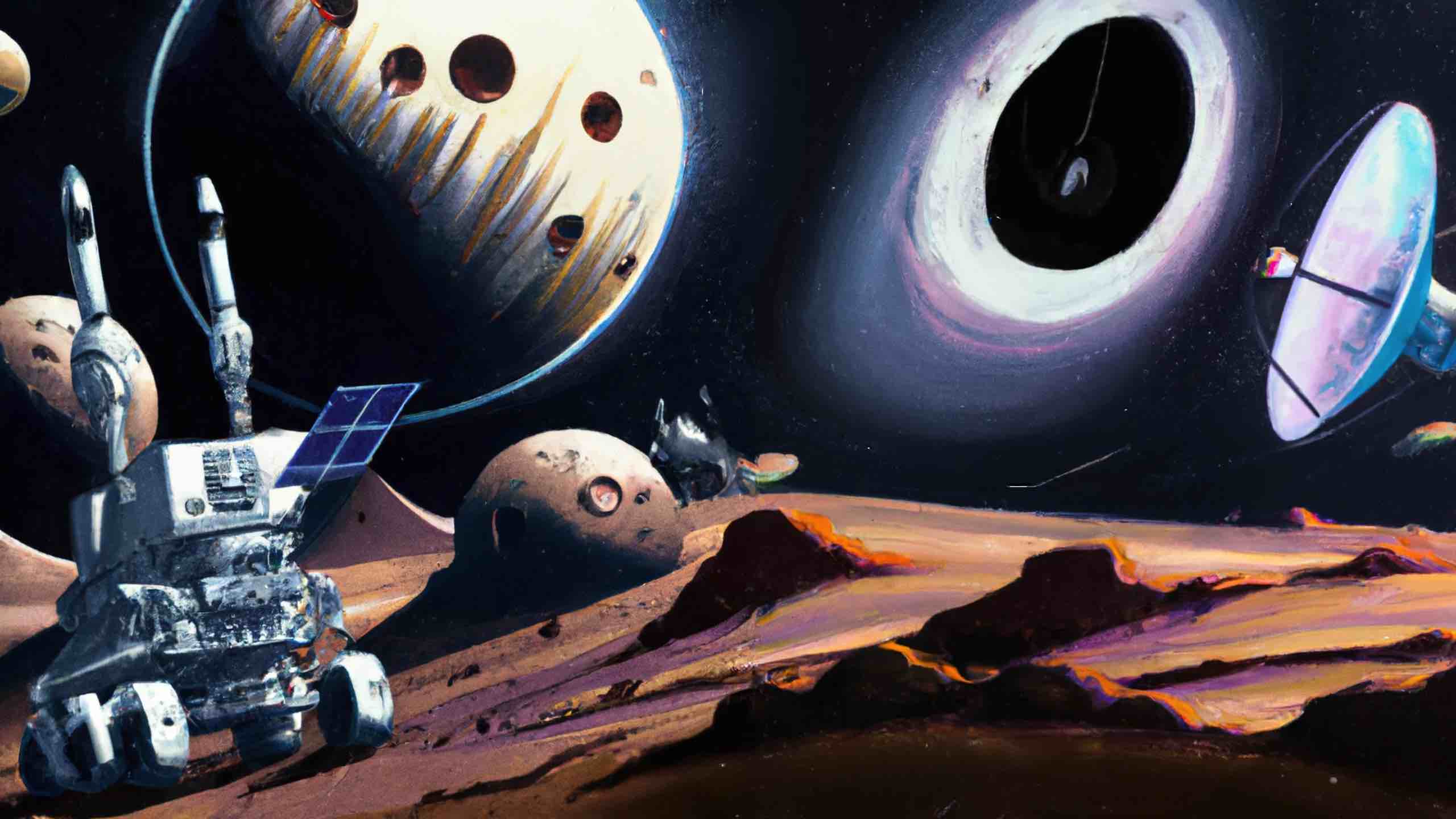
organised by the European Space Agency, 3rd place, M. Märtens F. Neumann, D. Antipov, A. Neumann, X. Yan
Articles accepted at the journal Springer Nature Algorithmica:
Fixed Parameter Multi-Objective Evolutionary Algorithms for the W-Separator Problem, S Baguley,T. Friedrich, A. Neumann, F. Neumann, M. Pappik, Z. Zeif, Dec 2024 [arXiv][paper]
Analysis of the (1+1) EA on LeadingOnes with Constraints, T. Friedrich, T. Kotzing, A. Neumann, F. Neumann, A. Radhakrishnan, Feb 2025 [arXiv][paper]
Best Paper Award at GECCO 2024, Real World Applications Track
Quality Diversity Approches for Time-Use Optimisation to Improve Health Outcomes, A. Nikfarjam, T. Stanford, A. Neumann, D. Dumuid, F. Neumann, Jul 2024 [ACM]
Article accepted at the journal Evolutionary Computation
Aneta Neumann and Frank Neumann: Optimizing Monotone Chance-Constrained Submodular Functions Using Evolutionary Multi-Objective Algorithms Evolutionary Computationn, Dec 2024 [paper][arXiv]
6 Papers accepted at PPSN 2024, Parallel Problem Solving from Nature
- K. Perera, F. Neumann, A. Neumann (2024): Multi-objective evolutionary approaches for the knapsack problem with stochastic profits. [Springer][arXiv]
- X. Yan, A. Neumann, F. Neumann (2024): Sliding window bi-objective evolutionary algorithms for optimizing chance-constrained monotone submodular functions. [Springer][arXiv]
- D. Antipov, A. Neumann, F. Neumann, A. M. Sutton (2024): Runtime analysis of evolutionary diversity optimization on the multi-objective (LeadingOnes, TrailingZeros) problem. [Springer][arXiv]
- A. V. Do, M. Guo, A. Neumann, F. Neumann (2024): Evolutionary multi-objective diversity optimization. [Springer][arXiv]
- J. G. Harder, A. Neumann, F. Neumann (2024): Analysis of evolutionary diversity optimisation for the maximum matching problem. [Springer][arXiv]
- D. Antipov, A. Neumann, F. Neumann (2024): Local optima in diversity optimization: non-trivial offspring population is essential. [Springer][arXiv]
Best Paper Nomination GECCO 2024
for the work "Quality Diversity Approaches for Time-Use Optimisation to Improve Health Outcomes", A. Nikfarjam, T. Stanford, A. Neumann, D. Dumuid, F. Neumann, at the Genetic and Evolutionary Computation Conference 2024, (Track Real World Applications), [ACM]
11 Papers accepted at GECCO 2024:
- K. Perera, A. Neumann, Multi-objective Evolutionary Algorithms with Sliding Window Selection for the Dynamic Chance-Constrained Knapsack Problem, [arXiv]
- S. Gounder, F. Neumann, A. Neumann, Evolutionary Diversity Optimisation for Sparse Directed Communication Networks [ACM]
- I. Hewa Pathiranage, F. Neumann, D. Antipov, A. Neumann, Effective 2- and 3-Objective MOEA/D Approaches for the Chance Constrained Knapsack Problem, [ACM]
- I. Hewa Pathiranage, F. Neumann, D. Antipov, A. Neumann, Using 3-Objective Evolutionary Algorithms for the Dynamic Chance Constrained Knapsack Problem, [arXiv]
- T. Pathirage Don, A. Neumann, F. Neumann, The Chance Constrained Travelling Thief Problem: Problem Formulations and Algorithms
- F. Ye, F. Neumann, J. de Nobel, A. Neumann, T. Bäck, What Performance Indicators to Use for Self-Adaptation in Multi-Objective Evolutionary Algorithms
- D. Antipov, A. Neumann, F. Neumann, A Detailed Experimental Analysis of Evolutionary Diversity Optimization for OneMinMax
- A. Nikfarjam, T. Stanford, A. Neumann, D. Dumuid, F. Neumann, Quality Diversity Approaches for Time-Use Optimisation to Improve Health Outcomes
- B. Doerr, J. Knowles, A. Neumann, F. Neumann, A Block-Coordinate Descent EMO Algorithm: Theoretical and Empirical Analysis, [arXiv]
- X. Yan, A. Neumann, F. Neumann, Sampling-based Pareto Optimization for Chance-constrained Monotone Submodular Problems, [arXiv]
- S. Sadeghi Ahouei, J. de Nobel, A. Neumann, T. Bäck, F. Neumann, Evolving Reliable Differentiating Constraints for the Chance-constrained Maximum Coverage Problem
Tutorial and paper accepted at IEEE WCCI 2024:
- Evolutionary Diversity Optimization for Combinatorial Optimization; Organizers: A. Neumann, F. Neumann
- I. Hewa Pathiranage, A. Neumann, Multi-Objective Evolutionary Optimization for Large-Scale Open Pit Mine Scheduling, [IEEE]
Paper accepted at AAAI 2024:
M. Guo, J. Li, A. Neumann, F. Neumann, H. Nguyen: Limited Query Graph Connectivity Test [arXiv]
Paper accepted at NeurIPS 2023:
A.V. Do, A Neumann, F. Neumann, A. M. Sutton: Rigorous Runtime Analysis of MOEA/D for Solving Multi-Objective Minimum Weight Base Problems [arXiv] [ACM]
Article accepted at the journal ACM Transactions on Evolutionary Learning and Optimization (TELO) 2024:
D. Goel, M. Ward, A. Neumann, F. Neumann, H. Nguyen, M. Guo: Hardening Active Directory Graphs via Evolutionary Diversity Optimization based Policies [Journal]
Article accepted at the journal ACM Transactions on Evolutionary Learning and Optimization (TELO) 2023:
A. Nikfarjam, A. Neumann, F. Neumann: On the Use of Quality Diversity Algorithms for the Traveling Thief Problem [Journal]
Invited to participate in a Lorentz Center Workshop in the Netherlands: Social Influence Analysis, 25-29 Sep 2023
Invited as a Keynote Speaker
at the Evolutionary Multi-Criterion Optimization, EMO Conference 2023, March 20-24, Leiden, The Netherlands.
Title: Mine Planning under Uncertainty: From Theory to Practice
Abstract: Evolutionary algorithms provide great flexibility in dealing with large-scale optimisation problems. Their wide applicability has made evolutionary computing techniques popular optimisation techniques for many real-world applications such as the mining industry. Long-term planning and production scheduling are among the most critical and complex tasks that involve many uncertainties in the area of mining. In this talk, I will discuss the application of evolutionary computation to problems with stochastic constraints as well as how to transfer theoretical results to real-world applications. In particular, I will show how we integrated theoretical findings into mine planning under uncertainty and explain some approaches that have been incorporated into industrial software for mine planning.
Invited to participate in a Industrial Panel discussion at EMO 2023, 24 Mar 2023.
Invited to participate in a Dagstuhl Seminar
Multiobjective Optimization on a Budget, 3 - 8 Sep 2023, Germany.
6 Papers accepted at GECCO 2023:
- A. Neumann, S. Goulder, X. Yan, G. Sherman, B. Campbell, M. Guo, F. Neumann: Evolutionary Diversity Optimization for the Detection and Concealment of Spatially Defined Communication Networks
- D. Goel, A. Neumann, F. Neumann, H. Nguyen, M. Guo: Evolving Reinforcement Learning Environment to Minimize Learner's Achievable Reward: An Application on Hardening Active Directory Systems
- T. Friedrich, T. Kötzing, A. Neumann, F. Neumann, A. Radhakrishnan: Analysis of (1+1) EA on LeadingOnes with Constraints
- S. Baguley, T. Friedrich, A. Neumann, F. Neumann, M. Pappik, Z. Zeif: Fixed Parameter Multi-Objective Evolutionary Algorithms for the W-Separator Problem
- A. Marrero, E. Segredo, E. Hart, J. Bossek, A. Neumann: Generating diverse and discriminatory knapsack instances by searching for novelty in variable dimensions of feature-space
- J. Bossek, A. Neumann, F. Neumann: On the Impact of Basic Mutation Operators and Populations within Evolutionary Algorithms for the Dynamic Weighted Traveling Salesperson Problem
Paper accepted at the AAAI 2023:
Mingyu Guo, Max Ward, Aneta Neumann, Frank Neumann, Hung Nguyen: Scalable Edge Blocking Algorithms for Defending Active Directory Style Attack Graphs.
2 Papers accepted at CEC 2023:
1. F. Neumann, A. Neumann, C. Qian, A.V. Do, J. de Nobel, D. Vermetten, S. Sadeghi Ahouei, F. Ye, H. Wang, T. Baeck: Benchmarking Algorithms for Submodular Optimization Probles Using IOHProfiler, IEEE 2023 Congress on Evolutionary Computation
2. M. Stimson, W. Reid, A. Neumann, S. Ratcliffe, F. Neumann: Improving Confidence in Evolutionary Mine Scheduling via Uncertainty Discounting, IEEE 2023 Congress on Evolutionary Computation
I serve as a Chair of the track Genetic Algorithm (GA) at the Genetic and Evolutionary Computation Conference, GECCO 2023.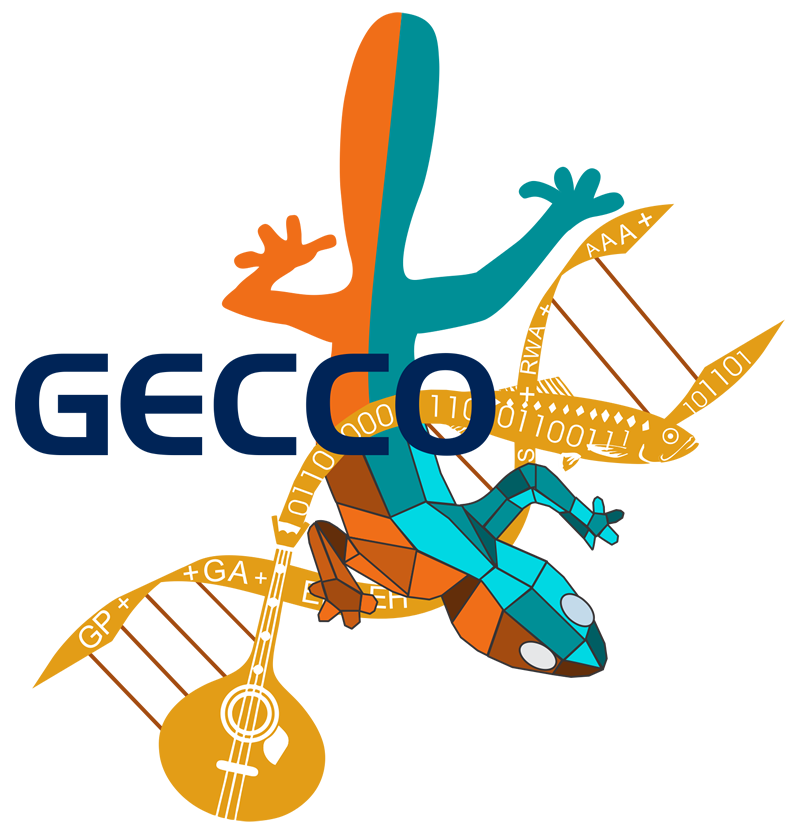
Article accepted at the journal ACM Transactions on Evolutionary Learning and Optimization 2022:
Analysis of Evolutionary Diversity Optimization for Permutation Problems. Authors: A.V. Do, M Guo, A. Neumann, F. Neumann [arXiv]
4 Papers accepted at PPSN 2022:
- Aneta Neumann, Yue Xie and Frank Neumann: Evolutionary Algorithms for Limiting the Effect of Uncertainty for the Knapsack Problem with Stochastic Profits [arXiv]
- Adel Nikfarjam, Aneta Neumann, Jakob Bossek and Frank Neumann: Co-Evolutionary Diversity Optimisation for the Traveling Thief Problem
- Adel Nikfarjam, Amirhossein Moosavi, Aneta Neumann and Frank Neumann: Computing High-Quality Solutions for the Patient Admission Scheduling Problem using Evolutionary Diversity Optimisation
- Yue Xie, Aneta Neumann, Ty Stanford, Charlotte Lund Rasmussen, Dorothea Dumuid and Frank Neumann: Evolutionary Time Use Optimization for Improving Children's Health Outcomes [arXiv]
Article accepted at the Journal Theoretical Computer Science 2022
Single- and Multi-Objective Evolutionary Algorithms for the Knapsack Problem with Dynamically Changing Constraints. Authors: V. Roostapour, A. Neumann, F. Neumann. [arXiv]
5 Papers accepted at GECCO 2022:
- Aneta Neumann, Denis Antipov, Frank Neumann: Coevolutionary Pareto Diversity Optimization [arXiv]
- Adel Nikfarjam, Aneta Neumann, Frank Neumann: Evolutionary Diversity Optimisation for the Traveling Thief Problem [arXiv]
- Anh Viet Do, Mingyu Guo, Aneta Neumann, Frank Neumann: Niching-based Evolutionary Diversity Optimization for the Traveling Salesperson Problem [arXiv]
- Diksha Goel, Max Hector Ward-Graham, Aneta Neumann, Frank Neumann, Hung Nguyen, Mingyu Gao: Defending Active Directory by Combining Neural Network based Dynamic Program and Evolutionary Diversity Optimisation [arXiv]
- Adel Nikfarjam, Aneta Neumann, Frank Neumann: On the Use of Quality Diversity Algorithms for the Traveling Thief Problem [arXiv]
I serve as a Chair of the track Real World Applications
(RWA) at the Genetic and Evolutionary Computation Conference, GECCO 2022
with Richard Allmendinger.
Invited to participate in a Round Table discussion at GECCO@WOMAN, GECCO 2022, 9 Jul 2022.
Paper accepted at the AAAI 2022:
Mingyu Guo, Jialiang Li, Aneta Neumann, Frank Neumann, Hung Nguyen: Practical Fixed-Parameter Algorithms for Defending Active Directory Style Attack Graphs [arXiv]
Competition "Evolutionary Submodular Optimisation" at GECCO 2022.
Tutorial "Evolutionary Submodular Optimisation" at GECCO 2021 and GECCO 2022.
Tutorial "Evolutionary Diversity Optimisation for Combinatorial Optimisation" at GECCO 2022.
Tutorials "Evolutionary Computation for Digital Art" and "Evolutionary Diversity Optimisation" at IEEE CEC 2021.
The 2022 Lorentz Center Workshop - (by-invitation only event):
Benchmarked: Optimization Meets Machine Learning 2022, Hybrid, Ort, Leiden 30 May –- 3 Jun 2022 Leiden, The Netherlands.
Article accepted at the journal of Artificial Intelligence (AIJ)
Pareto optimization for subset selection with dynamic cost constraints. Authors: V. Roostapour, A. Neumann, F. Neumann, T. Friedrich, [arXiv]
Best Paper Nomination (GECCO 2021) -
for the work ``Analysis of Evolutionary Diversity Optimisation for Permutation Problems'', A.V Do, M. Guo, A, Neumann, F. Neumann at the Genetic and Evolutionary Computation Conference 2021, (Track Genetic Algorithms).
Co-Chair of the Real World Applications (RWA)
track at the Genetic and Evolutionary Computation Conference, GECCO 2021
with Richard Allmendinger.
Tutorial on Evolutionary Submodular Optimisation at GECCO 2021
with Frank Neumann and Chao Qian accepted at Genetic and Evolutionary Computation Conference (GECCO) 2021.
Tutorials on Evolutionary Computation for Digital Art and Evolutionary Diversity Optimisation at IEEE CEC 2021.
Co-organiser of Special Issue of Theoretical Computer Science titled "Theoretical Foundations of Evolutionary Computation''
with Per Kristian Lehre and Chao Qian, Submission Deadline: December 31, 2021.
Paper accepted at FOGA 2021:
Computing Diverse Sets of High Quality TSP Tours by EAX-Based Evolutionary Diversity Optimisation, Authors: A. Nikfarjam, J. Bossek, A. Neumann, F. Neumann, [arXiv], [download]
Paper with industry partner: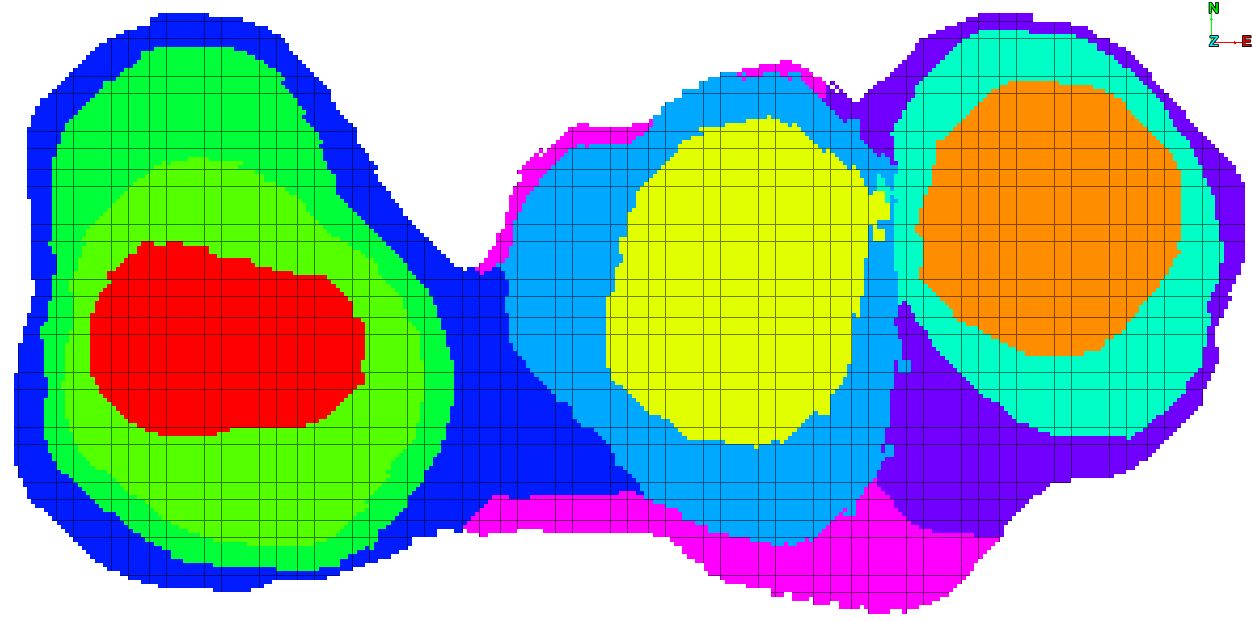
Maptek accepted at GECCO 2021 Companion, Workshop on Industrial Applications of Metaheuristics: Advanced Mine Optimisation under Uncertainty Using Evolution, [download]
6 Papers accepted at GECCO 2021:
- Diversifying Greedy Sampling and Evolutionary Diversity Optimisation for Constrained Monotone Submodular Functions. Authors: Aneta Neumann, J. Bossek, F. Neumann, [arXiv]
- Heuristic Strategies for Solving Complex Interacting Stockpile Blending Problem with Chance Constraints. Authors: Y. Xie, Aneta Neumann, F. Neumann, [arXiv]
- Runtime Analysis of RLS and the (1+1) EA for the Chance-constrained Knapsack Problem with Correlated Uniform Weights. Authors: Y. Xie, Aneta Neumann, F. Neumann, A. M. Sutton, [arXiv]
- Analysis of Evolutionary Diversity Optimisation for Permutation Problems. Authors: A. V. Do, M. Guo, Aneta Neumann, F. Neumann, [arXiv]
- Breeding Diverse Packings for the Knapsack Problem by Means of Diversity-Tailored Evolutionary Algorithms. Authors: J. Bossek, Aneta Neumann, F. Neumann, [arXiv]
- Entropy-Based Evolutionary Diversity Optimisation for the Traveling Salesperson Problem. Authors: A. Nikfarjam, J. Bossek, Aneta Neumann, F. Neumann, [arXiv]
Paper accepted at CEC 2021:
Heuristic Strategies for Solving Complex Interacting Large-Scale Stockpile Blending Problems. Authors: Y. Xie, Aneta Neumann, F. Neumann, [arXiv]
Paper accepted at LION 2021:
Exact Counting and Sampling of Optima for the Knapsack Problem. Authors: J. Bossek, A. Neumann, F. Neumann, [arxiv]
The 2020 Lorentz Center Workshop (by-invitation only event):
Benchmarked: Optimization meets Machine Learning 9--13 November 2020, Leiden, The Netherlands.
Special Session accepted for WCCI 2020 - Theoretical Foundations of Bio-inspired Computation
Organizators: Per Kristian Lehre, Aneta Neumann, Chao Qian.
Tutorial on Evolutionary Diversity Optimisation
at the International Conference on Parallel Problem Solving from Nature (PPSN XVI) in 2020 with Jakob Bossek and Frank Neumann.
Tutorial on Evolutionary Computation for Digital Art at GECCO 2020
Tutorial on Evolutionary Computation for Digital Art with Frank Neumann accepted at Genetic and Evolutionary Computation Conference (GECCO) 2020. The tutorial slides Evolutionary Computation for Digital Art , Vimeo .
Papers accepted at PPSN 2020:
Optimising Chance-Constrained Submodular Functions Using Evolutionary Multi-Objective Algorithms, [arXiv] Jun 2020
Authors: Aneta Neumann and Frank Neumann
Optimising tours for the weighted traveling salesperson problem and the traveling thief problem: A structural comparison of solutions, [arXiv] Jun 2020
Authors: Jakob Bossek, Aneta Neumann and Frank Neumann
Evolving Sampling Strategies for One-Shot Optimization Tasks, [arXiv] Jun 2020
Authors: Jakob Bossek, Carola Doerr, Pascal Kerschke, Aneta Neumann and Frank Neumann
Papers accepted at GECCO 2020:
Specific Single- and Multi-Objective Evolutionary Algorithms for the Chance-Constrained Knapsack Problem, [arXiv] Apr 2020
Authors: Yue Xie, Aneta Neumann, Frank Neumann
Evolving Diverse Sets of Tours for the Travelling Salesperson Problem, [arXiv] Apr 2020
Authors: Viet Anh Do, Jakob Bossek, Aneta Neumann, Frank Neumann
Paper accepted at Evolutionary Computation Journal, MIT Press:
Evolutionary Image Transition and Painting Using Random Walks, [download] [arXiv], Mar 2020
Authors: Aneta Neumann, Bradley Alexander, Frank Neumann
Papers accepted at ECAI 2020:
Evolutionary Bi-objective Optimization for the Dynamic Chance-Constrained Knapsack Problem Based on Tail Bound Objectives, [arXiv] 2020
Authors: Hirad Assimi, Oscar Harper, Yue Xie, Aneta Neumann and Frank Neumann
Non-Monotone Submodular Maximization with Multiple Knapsacks in Static and Dynamic Settings,
Authors: Vanja Doskoc, Tobias Friedrich, Andreas Göbel, Aneta Neumann, Frank Neumann and Francesco Quinzan, [arXiv] 2020
Paper accepted at AAAI 2020: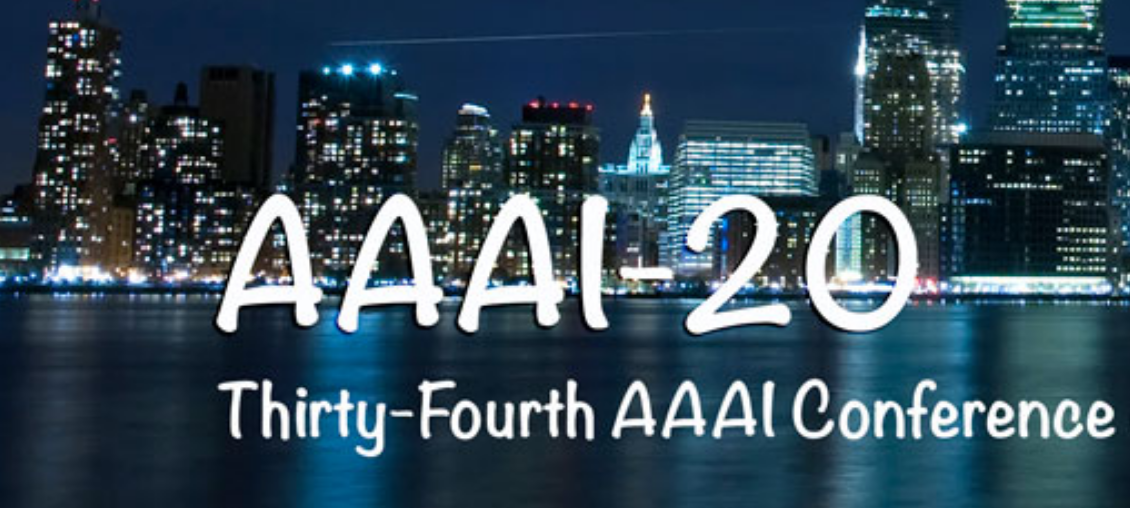
Optimization of Chance-Constrained Submodular Functions,
Authors: B. Doerr, C. Doerr, A. Neumann, F. Neumann, A. M. Sutton
[download], 2020
The 2019 Workshop on AI-based Optimisation (AI-OPT 2019)
Artificial Intelligence based optimisation techniques such as constraint programming, evolutionary computation, heuristic search, mixed integer programming, and swarm intelligence have found many applications in solving highly complex and challenging optimisation problems. Application domains include important areas such as cybersecurity, economics, engineering, renewable energy, health and supply chain management.
Tutorial on Evolutionary Computation for Digital Art at GECCO 2019
Tutorial on Evolutionary Computation for Digital Art with Frank Neumann accepted at Genetic and Evolutionary Computation Conference (GECCO) 2019. The tutorial slides Evolutionary Computation for Digital Art, Vimeo.
Paper accepted at FOGA 2019, Code:
Evolving Diverse TSP Instances by Means of Novel and Creative Mutation Operators, Authors: J. Bossek, P. Kerschke, A. Neumann, M. Wagner, F. Neumann, H. Trautmann
STEM WORKSHOPS in German with GOETHE INSTITUT
Papers accepted at GECCO 2019, Code:
Evolutionary Diversity Optimization Using Multi-Objective Indicators - Nominated for Best Paper Award in the track "Genetic Algorithms"
Authors: Aneta Neumann, Wanru Gao, Markus Wagner, Frank Neumann, [download] [researchgate] July 2019
Evolutionary Algorithms for the Chance-Constrained Knapsack Problem
Authors: Yue Xie, Oscar Harper, Hirad Assimi, Aneta Neumann, Frank Neumann, [download] [researchgate] July 2019
Tutorial on Evolutionary Computation for Digital Art at GECCO 2019
Tutorial on Evolutionary Computation for Digital Art with Frank Neumann accepted at Genetic and Evolutionary Computation Conference (GECCO 2019).
GMG Adelaide Forum: Secure and Integrated Energy and Mining Systems, 2019
Bringing the mining and energy industries together to achieve secure integration across the sector with Prof Stephen Grano, Executive Director of the University of Adelaide’s Institute for Mineral and Energy Resources (IMER).
Paper accepted at AAAI 2019:
V. Roostapour, A. Neumann, F. Neumann, T. Friedrich (2019): Pareto optimization for subset selection with dynamic cost constraints. In: Thirty-Third AAAI Conference on Artificial Intelligence, AAAI 2019, download, [arxiv], Jul 2019
Tutorial on Evolutionary Computation for Digital Art at AI 2018
Tutorial on Evolutionary Computation for Digital Art with Frank Neumann accepted at the Australasian Joint Conference on Artificial Intelligence (AI 2018), LNCS 11320, Springer. The tutorial slides Evolutionary Computation for Digital Art, last updated: 11 December 2018.
Aneta has been awarded a Hans-Juergen and Marianna Ohff Research Grant for 2018
This grant will support a research visit at the Algorithm Engineering group lead by Prof. Dr. Tobias Friedrich, the Hasso Plattner Institute Potsdam, Germany. Media: Research grant for Aneta Neumann
Aneta has been awarded an ACM-W scholarship 2018,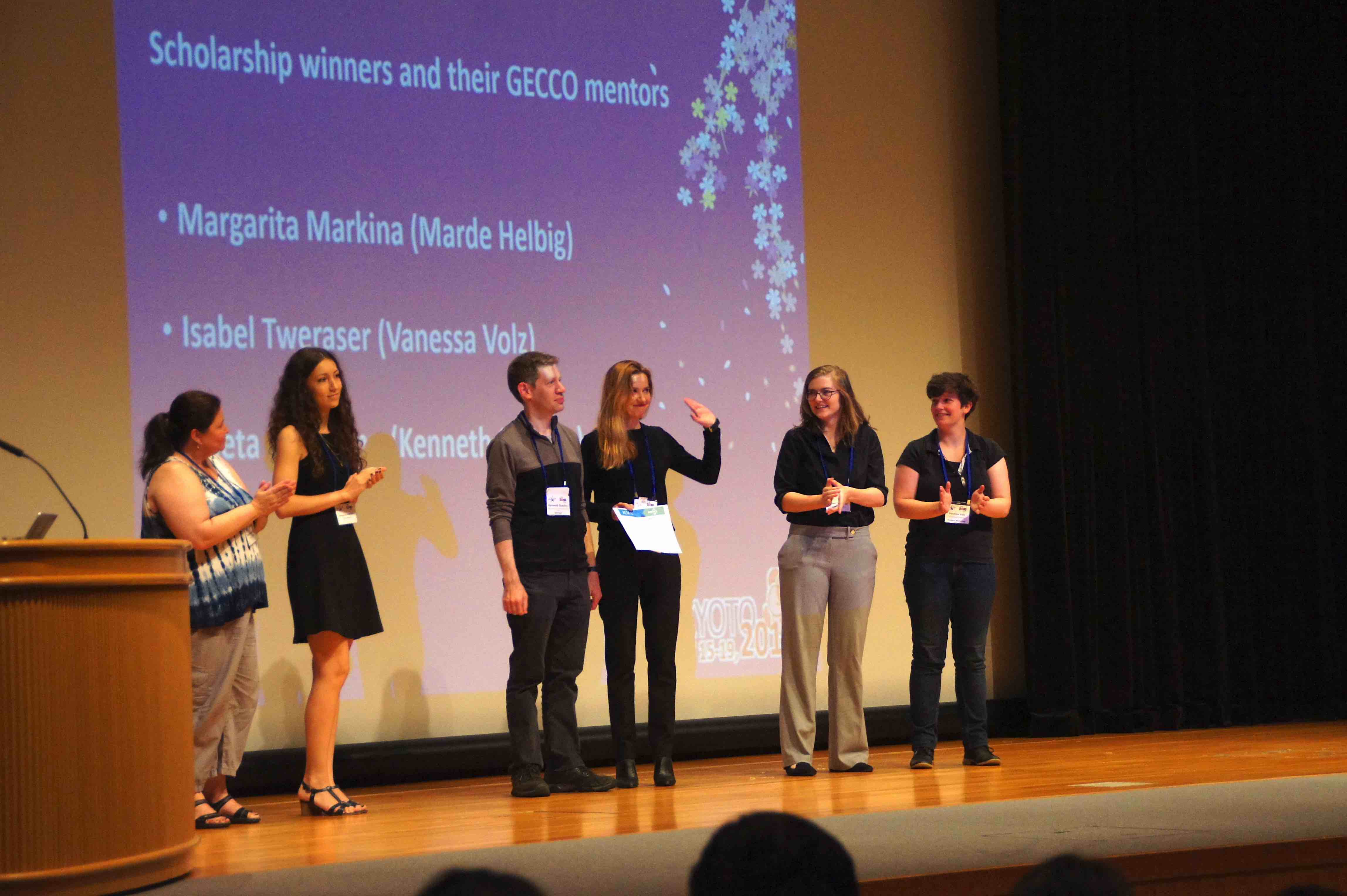
sponsored by Google, Microsoft, Oracle. Media:
Aneta Neumann Recipient of ACM-W Scholarship [09/2018], GECCO 2018, ACM-W Awards
Tutorial on Evolutionary Computation for Digital Art at GECCO 2018,
Tutorial on Evolutionary Computation for Digital Art with Frank Neumann accepted at Genetic and Evolutionary Computation Conference (GECCO) 2018. The tutorial slides Evolutionary Computation for Digital Art, last updated: 20 July 2018.
Big Data Fundamentals MOOC's course
Aneta is a co-designer and co-lecturer for Big Data Fundamentals (Start Date: Mar 1, 2019) course in the Big Data MicroMasters® program, an open online graduate level series of courses https://blogs.adelaide.edu.au/adelaidex/2017/05/24/adelaidex-launches-big-data-micromasters/.
Cover Page on SIGEVOlution
SIGEVOlution newsletter of the ACM Special Interest Group on Genetic and Evolutionary Computation, Volume 10, Issue 3, http://www.sigevolution.org/issues/SIGEVOlution1003.pdf
CS Researcher in SALA Art Exhibition
Aneta Neumann, a researcher from the School of Computer Science is exhibiting two mixed media artworks in Hub Central. The artworks are inspired by images produced by new methods in evolutionary image transition, pioneered by Aneta in her research toward her PhD. This research carried out within the Optimisation and Logistic Research Group in the School explores the fundamental link between evolutionary processes and generative art. The images will be on display in the Hub until the 18th of August. http://blogs.adelaide.edu.au/cs/2017/08/15/cs-researcher-in-sala-art-exhibition/
Research Interests:
artificial intelligence, machine learning, evolutionary computation, multi-objective optimisation, quality diversity, generative art, computational creativity
Publications:
Human Interactive EEG-Based Evolutionary Image Animation
Authors: Aneta Neumann and Frank Neumann
Accepted as a full paper for publication at the 2020 IEEE Symposium Series on Computational Intelligence
ABSTRACT: Evolutionary algorithms are adaptive algorithms that can alter their behaviour under changing circumstances.This makes them well-suited to act in interactive environments.We introduce a framework for human interactive animations based on EEG signals. EEG signals received through a brain computer interface headset are used as the fitness function and influence the animation based on the signals received. For our experimental investigation, we consider the recently introduced quasi-random animation process and alter the process through mutation operators that depend on the EEG signals received.Furthermore, the EEG signals trigger mutations and therefore alterations to the image animation process if their values are not in a predefined range. Our experimental study shows that a large variety of animations and intermediate images are obtainable in this way.
Evolutionary Bi-objective Optimization for the Dynamic Chance-Constrained Knapsack Problem Based on Tail Bound Objectives
Authors: Hirad Assimi, Oscar Harper, Yue Xie, Aneta Neumann and Frank Neumann
Accepted as a full paper for publication at the ECAI 2020, [arxiv], 2020
ABSTRACT: Real-world optimization problems are often stochastic and dynamic and it is important to tackle stochastic and dynamic environments in a common approach. In this paper, we consider the stochastic chance-constrained knapsack problem where the constraint bound dynamically changes over time. We introduce a Pareto optimization approach for this problem that make use of important tail inequalities such as Chebyshev's inequality and Chernoff bound to estimate the probability of exceeding a given constraint bound. The key part of our approach is the introduction of an additional objective which calculates the minimal constraint bound for which a given solution for the stochastic component would still meet the chance constraint. This objective helps to cater for dynamic changes to the stochastic problem. Our experimental investigations show that the Pareto optimization is highly effective and outperforms its corresponding single-objective approach.
Non-Monotone Submodular Maximization with Multiple Knapsacks in Static and Dynamic Settings
Authors: Vanja Doskoc, Tobias Friedrich, Andreas Göbel, Aneta Neumann, Frank Neumann and Francesco Quinzan
Accepted as a full paper for publication at the ECAI 2020, [arxiv], 2020
ABSTRACT: We study the problem of maximizing a non-monotone submodular function under multiple knapsack constraints. We propose a simple discrete greedy algorithm to approach this problem, and prove that it yields strong approximation guarantees for functions with bounded curvature. In contrast to other heuristics, this requires no problem relaxation to continuous domains and it maintains a constant-factor approximation guarantee in the problem size. In the case of a single knapsack, our analysis suggests that the standard greedy can be used in non-monotone settings. Additionally, we study this problem in a dynamic setting, in which knapsacks change during the optimization process. We modify our greedy algorithm to avoid a complete restart at each constraint update. This modification retains the approximation guarantees of the static case. We evaluate our results experimentally on a video summarization and sensor placement task. We show that our proposed algorithm competes with the state-of-the-art in static settings. Furthermore, we show that in dynamic settings with tight computational time budget, our modified greedy yields significant improvements over starting the greedy from scratch, in terms of the solution quality achieved.
Optimization of Chance-Constrained Submodular Functions,
Authors: B. Doerr, C. Doerr, A. Neumann, F. Neumann, A. M. Sutton
Accepted as a full paper for publication at the AAAI 2020
[download], 2020
ABSTRACT: Submodular optimization plays a key role in many real-world problems. In many real-world scenarios, it is also necessary to handle uncertainty, and potentially disruptive events that violate constraints in stochastic settings need to be avoided.In this paper, we investigate submodular optimization problems with chance constraints. We provide a first analysis on the approximation behavior of popular greedy algorithms for submodular problems with chance constraints. Our results show that these algorithms are highly effective when using surrogate functions that estimate constraint violations based on Chernoff bounds. Furthermore, we investigate the behavior of the algorithms on popular social network problems and show that high quality solutions can still be obtained even ift here are strong restrictions imposed by the chance constraint.
Evolving diverse TSP instances by means of novel and creative mutation operators
Authors: J. Bossek, P. Kerschke, A. Neumann, M. Wagner, F. Neumann, H. Trautmann
Accepted as a full paper for publication at the FOGA 2019,
[download], 2019
ABSTRACT: Evolutionary algorithms have successfully been applied to evolve problem instances that exhibit a significant difference in performance for a given algorithm or a pair of algorithms inter alia for the Traveling Salesperson Problem (TSP). Creating a large variety of instances is crucial for successful applications in the blooming field of algorithm selection. In this paper, we introduce new and creative mutation operators for evolving instances of the TSP. We show that adopting those operators in an evolutionary algorithm allows for the generation of benchmark sets with highly desirable properties: (1) novelty by clear visual distinction to established benchmark sets in the field, (2) visual and quantitative diversity in the space of TSP problem characteristics, and (3) significant performance differences with respect to the restart versions of heuristic state-of-the-art TSP solvers EAX and LKH. The important aspect of diversity is addressed and achieved solely by the proposed mutation operators and not enforced by explicit diversity preservation.
Quasi-random Agents for Image Transition and Animation
Accepted as for journal publication at Australian Journal of Intelligent Information Processing Systems,
Authors: Aneta Neumann, Frank Neumann, Tobias Friedrich
ABSTRACT: Quasi-random walks show similar features as standard random walks, but with much less randomness. We utilize this established model from discrete mathematics and show how agents carrying out quasi-random walks can be used for image transition and animation. The key idea is to generalize the notion of quasi-random walks and let a set of autonomous agents perform quasi-random walks painting an image. Each agent has one particular target image that they paint when following a sequence of directions for their quasi-random walk. The sequence can easily be chosen by an artist and allows them to produce a wide range of different transition patterns and animations.
Evolving Pictures in Image Transition Space
Authors: B. Alexander, D. Hin, A. Neumann, S. Ull-Karim
Accepted as a full paper for publication at the ICONIP 2019
[download], 2019
ABSTRACT: Evolutionary art creates novel images through a processes inspired by natural selection. Images are high dimensional objects, which can present challenges for evolutionary processes. Work to date has handled this problem by evolving compressed or encoded forms of images or by starting with prior images and evolving constrained variations of these. In this work we extend the prior-image concept by evolving interesting images in the transition-space between two bounding images. We define new feature metrics based on proximity to the two bounding images and show how these metrics, combined with other aesthetic features, can be used to drive the creation of new images incorporating features of both starting images. We extend this work further to evolve sets images that are diverse in one and two feature dimensions. Finally, we accelerate this evolutionary process using an autoencoder to capture the transition space and reduce the dimensionality of the search space.
Evolutionary Diversity Optimization Using Multi-Objective Indicators
Authors: Aneta Neumann, Wanru Gao, Markus Wagner, Frank Neumann
Accepted as a full paper for publication at the Genetic and Evolutionary Computation Conference, GECCO 2019, was nominated for Best Paper Award for in the track "Genetic Algorithms"
[download] [paper] [arxiv], 2019
ABSTRACT: Evolutionary diversity optimization aims to compute a diverse set of solutions where all solutions meet a given quality criterion. With this paper, we bridge the areas of evolutionary diversity optimization and evolutionary multi-objective optimization. We show how popular indicators frequently used in the area of multi-objective optimization can be used for evolutionary diversity optimization. Our experimental investigations for evolving diverse sets of TSP instances and images according to various features show that two of the most prominent multi-objective indicators, namely the hypervolume indicator and the inverted generational distance, provide excellent results in terms of visualization and various diversity indicators.
Evolutionary Algorithms for the Chance-Constrained Knapsack Problem
Authors: Yue Xie, Oscar Harper, Hirad Assimi, Aneta Neumann, Frank Neumann
Accepted as a full paper for publication at the Genetic and Evolutionary Computation Conference, GECCO 2019,
[download] [paper] [arxiv], 2019
ABSTRACT: Evolutionary algorithms have been widely used for a range of stochastic optimization problems. In most studies, the goal is to optimize the expected quality of the solution. Motivated by real-world problems where constraint violations have extremely disruptive effects, we consider a variant of the knapsack problem where the profit is maximized under the constraint that the knapsack capacity bound is violated with a small probability of at most $\alpha$. This problem is known as chance-constrained knapsack problem and chance-constrained optimization problems have so far gained little attention in the evolutionary computation literature. We show how to use popular deviation inequalities such as Chebyshev's inequality and Chernoff bounds as part of the solution evaluation when tackling these problems by evolutionary algorithms and compare the effectiveness of our algorithms on a wide range of chance-constrained knapsack instances.
Pareto optimization for subset selection with dynamic cost constraints
Authors: V. Roostapour, A. Neumann, F. Neumann, T. Friedrich
Accepted as a full paper for publication at Thirty-Third AAAI Conference on Artificial Intelligence, AAAI 2019,
ABSTRACT: In this paper, we consider subset selection problem for function f with constraint bound B which changes over time. We point out that adaptive variants of greedy approaches commonly used in the area of submodular optimization are not able to maintain their approximation quality. Investigating the recently introduced POMC Pareto optimization approach, we show that this algorithm efficiently computes a $\phi= (\alpha_f/2)(1-\frac{1}{e^{\alpha_f}})$-approximation, where αf is the submodularity ratio of f, for each possible constraint bound b ≤ B. Furthermore, we show that POMC is able to adapt its set of solutions quickly in the case that B increases. Our experimental investigations for the influence maximization in social networks show the advantage of POMC over generalized greedy algorithms.
On the Performance of Baseline Evolutionary Algorithms on the Dynamic Knapsack Problem
Accepted as a full paper for publication at Parallel Problem Solving from Nature (PPSN 2018), [download], BENCHMARK [download],
Authors: Vahid Roostapour, Aneta Neumann, Frank Neumann
ABSTRACT: Evolutionary algorithms are bio-inspired algorithms that can easily adapt to changing environments. In this paper, we study single- and multi-objective baseline evolutionary algorithms for the classical knapsack problem where the capacity of the knapsack varies over time. We establish different benchmark scenarios where the capacity changes every tau iterations according to a uniform or Normal distribution. Our experimental investigations analyze the behavior of our algorithms in terms of the magnitude of changes determined by parameters of the chosen distribution, the frequency determined by tau and the class of knapsack instance under consideration. Our results show that the multi-objective approaches using a population that caters for dynamic changes have a clear advantage on many benchmarks scenarios when the frequency of changes is not too high.
Discrepancy-based Evolutionary Diversity Optimisation
Accepted as a full paper for publication at GECCO 2018, [bibtex], [arxiv], [download],
Authors: Aneta Neumann, Wanru Gao, Carola Doerr, Frank Neumann, Markus Wagner
ABSTRACT: Diversity plays a crucial role in evolutionary computation. While diversity has been mainly used to prevent the population of an evolutionary algorithm from premature convergence, the use of evolutionary algorithms to obtain a diverse set of solutions has gained increasing attention in recent years. Diversity optimization in terms of features on the underlying problem allows to obtain a better understanding of possible solutions to the problem at hand and can be used for algorithm selection when dealing with combinatorial optimization problems such as the Traveling Salesperson Problem. We explore the use of the star-discrepancy measure to guide the diversity optimization process of an evolutionary algorithm.
In our experimental investigations, we consider our discrepancy-based diversity optimization approaches for evolving diverse sets of images as well as instances of the Traveling Salesperson problem where a local search is not able to find near optimal solutions. Our experimental investigations comparing three diversity optimization approaches show that a discrepancy-based diversity optimization approach using a tie-breaking rule based on weighted differences to surrounding feature points provides the best results in terms of the star discrepancy measure.
On the Use of Colour-based Segmentation in Evolutionary Image Composition
Accepted as a full paper for publication at IEEE CEC 2018 [download],
Authors: Aneta Neumann, Frank Neumann
ABSTRACT: Evolutionary algorithms have been widely used in the area of creativity in order to help create art and music. We consider the recently introduced evolutionary image composition approach based on feature covariance matrices [1] which allows composing two images into a new one based on their feature characteristics. When using evolutionary image composition it is important to obtain a good weighting of interesting regions of the two images. We use colour-based segmentation based on K-Means clustering to come up with such a weighting of the images. Our results show that this preserves the chosen colour regions of the images and leads to composed images that preserve colours better than the previous approach based on saliency masks [1]. Furthermore, we evaluate our composed images in terms of aesthetic feature and show that our approach based on colour-based segmentation leads to higher feature values for most of the investigated features.
Evolution of Images with Diversity and Constraints Using a Generator Network
Accepted as a full paper for publication at International Conference on Neural Information Processing (ICONIP 2018), [bibtex], [arxiv], [download],
Authors: Aneta Neumann, Christo Pyromallis, Bradley Alexander
ABSTRACT: Evolutionary search has been extensively used to generate artistic images. Raw images have high dimensionality which makes a direct search for an image challenge. In previous work, this problem has been addressed by using compact symbolic encodings or by constraining images with priors. Recent developments in deep learning have enabled a generation of compelling artistic images using generative networks that encode images with lower-dimensional latent spaces. To date, this work has focused on the generation of images concordant with one or more classes and transfer of artistic styles. There is currently no work which uses search in this latent space to generate images scoring high or low aesthetic measures. In this paper, we use evolutionary methods to search for images in two datasets, faces and butterflies and demonstrate the effect of optimising aesthetic feature scores in one or two dimensions. The work gives a preliminary indication of which feature measures promote the most interesting images and how some of these measures interact.
Evolutionary Image Composition Using Feature Covariance Matrices
The Genetic and Evolutionary Computation Conference (GECCO 2017) [ bibtex ] [download] [arxiv],
Authors: Aneta Neumann, Zygmunt L Szpak, Wojciech Chojnacki, Frank Neumann
ABSTRACT: Evolutionary algorithms have recently been used to create a wide range of artistic work. In this paper, we propose a new approach for the composition of new images from existing ones, that retain some salient features of the original images. We introduce evolutionary algorithms that create new images based on a fitness function that incorporates feature covariance matrices associated with different parts of the images. This approach is very flexible in that it can work with a wide range of features and enables targeting specific regions in the images. For the creation of the new images, we propose a population-based evolutionary algorithm with mutation and crossover operators based on random walks. Our experimental results reveal a spectrum of aesthetically pleasing images that can be obtained with the aid of our evolutionary process.
Evolution of Artistic Image Variants Through Feature Based Diversity Optimisation
The Genetic and Evolutionary Computation Conference (GECCO 2017) [bibtex] [download],
Authors: Bradley Alexander, James Kortman, Aneta Neumann
ABSTRACT: Measures aimed to improve the diversity of images and image features in evolutionary art help to direct search toward more novel and creative parts of the artistic search domain. To date, such measures have focused on relatively indirect means of ensuring diversity in the context of search to maximise an aesthetic or similarity metric. In recent work on TSP problem instance classification, selection based on a direct measure of each individual's contribution to diversity was successfully used to generate hard and easy TSP instances. In this work, we use an analogous search framework to evolve diverse variants of a source image in one and two feature dimensions. The resulting images show the spectrum of effects from transforming images to score across the range of each feature. The evolutionary process also reveals interesting correlations between feature values in both one and two dimensions.
Multi-objectiveness in the Single-objective Traveling Thief Problem
The Genetic and Evolutionary Computation Conference (GECCO 2017), poster, [bibtex] [download],
Authors: Mohamed El Yafrani, Shelvin Chand, Aneta Neumann, Belaid Ahiod, Markus Wagner
ABSTRACT: Multi-component problems are optimization problems that are composed of multiple interacting sub-problems. The motivation of this work is to investigate whether it can be better to consider multiple objectives when dealing with multiple interdependent components. Therefore, the Travelling Thief Problem, a relatively new benchmark problem, is investigated as a bi-objective problem. An NSGA-II adaptation for the bi-objective model is compared to three of the best known algorithms for the original single-objective problem. The results show that our approach generates diverse sets of solutions while being competitive with the state-of-the-art single-objective algorithms.
Evolutionary Image Transition Using Random Walks
Evolutionary and Biologically Inspired Music, Sound, Art and Design (EvoMUSART’17). Springer, Cham. [bibtex] [download], [download Springer]
Authors: Aneta Neumann, Bradley Alexander, Frank Neumann
ABSTRACT: We present a study demonstrating how random walk algorithms can be used for evolutionary image transition. We design different mutation operators based on uniform and biased random walks and study how their combination with a baseline mutation operator can lead to interesting image transition processes in terms of visual effects and artistic features. Using feature-based analysis we investigate the evolutionary image transition behaviour with respect to different features and evaluate the images constructed during the image transition process.
A Modified Indicator-based Evolutionary Algorithm (mIBEA)
IEEE Congress on Evolutionary Computation 2017, San Sebastián, [bibtex] [download],
Authors: Li,W, Ozcan, E, John, R, Drake, JH, Neumann, A, Wagner, M
ABSTRACT: Multi-objective evolutionary algorithms (MOEAs) based on the concept of Pareto-dominance have been successfully applied to many real-world optimisation problems. Recently, research interest has shifted towards indicator-based methods to guide the search process towards a good set of trade-off solutions. One commonly used approach of this nature is the indicator-based evolutionary algorithm (IBEA). In this study, we highlight the solution distribution issues within IBEA and propose a modification of the original approach by embedding an additional Pareto-dominance based component for selection. The improved performance of the proposed modified IBEA (mIBEA) is empirically demonstrated on the well-known DTLZ set of benchmark functions. Our results show that mIBEA achieves comparable or better hypervolume indicator values and epsilon approximation values in the vast majority of our cases (13 out of 14 under the same default settings) on DTLZ1-7. The modification also results in an over 8-fold speed-up for larger populations.
The Evolutionary Process of Image Transition in Conjunction with Box and Strip Mutation
International Conference on Neural Information Processing (ICONIP2016) [bibtex] [url] [download],
Authors: Aneta Neumann, Bradley Alexander, Frank Neumann
ABSTRACT: Evolutionary algorithms have been used in many ways to generate digital art. We study how the evolutionary processes can be used for evolutionary art and present a new approach to the transition of images. Our main idea is to define evolutionary processes for digital image transition, combining different variants of mutation and evolutionary mechanisms. We introduce box and strip mutation operators which are specifically designed for image transition. Our experimental results show that the process of an evolutionary algorithm in combination with these mutation operators can be used as a valuable way to produce unique generative art.
Evolutionary Image Transition Based on Theoretical Insights of Random Processes [download]
Authors: Aneta Neumann, Bradley Alexander, Frank Neumann
ABSTRACT: Evolutionary algorithms have been widely studied from a theoretical perspective. In particular, the area of runtime analysis has contributed significantly to a theoretical understanding and provided insights into the working behaviour of these algorithms. We study how these insights into evolutionary processes can be used for evolutionary art. We introduce the notion of evolutionary image transition which transfers a given starting image into a target image through an evolutionary process. Combining standard mutation effects known from the optimization of the classical benchmark function OneMax and different variants of random walks, we present ways of performing evolutionary image transition with different artistic effects.
Invited lectures/talks/scientific visit:
- The University of Sydney, Australia, Dec 2019
- The 2019 Workshop on AI-based Optimisation (AI-OPT 2019), Melbourne, Australia, Oct 2019
- The scientific visit to the Sorbonne University, Paris, France, May 2019
- The scientific visit to Algorithm Engineering Group at the Hasso Plattner Institute, Potsdam, Germany, Apr/May 2019
- AAAI Conference on Artificial Intelligence, AAAI 2019, USA, Jan/Feb 2019
- The scientific visit to Algorithm Engineering Group at the Hasso Plattner Institute, Potsdam, Germany, Oct 2018
- The scientific visit to the Sorbonne University, Paris, France, May 2018
- University of Münster, Germany, May 2018
- The scientific visit to Algorithm Engineering Group at the Hasso Plattner Institute, Potsdam, Germany, Apr 2018
- Goldsmiths, University of London, Nov 2017
- The scientific visit to Mixed Reality Laboratory at the University of Nottingham, UK, Oct/Nov 2017
- Invited talk, University of Sheffield, Department of Computer Science, UK, Oct 2017
- The scientific visit to Algorithm Engineering Group at the Hasso Plattner Institute, Potsdam, Germany, Apr/May 2017
- Invited talk, Goldsmiths, University of London, UK, Dec 2016
- Invited talk, University College London, Department of Computer Science, London, UK, Dec 2016
- The scientific visit to University of Sheffield, Department of Computer Science, UK, Dec 2016
- The scientific visit to University of Nottingham, School of Computer Science, UK, Nov 2016
Conference Programme Committee/Member:
- Association for the Advancement of Artificial Intelligence (AAAI), 2019, 2020, 2021, 2022
- The International Conference on Learning Representations (ICLR), 2020, 2021
- The International Conference on Machine Learning (ICML), 2020
- The International Conference on Computational Intelligence in Music, Sound, Art and Design, 2021
- The International Joint Conference on Artificial Intelligence (IJCAI), 2020, 2021
- The International Joint Conference on Neural Networks (IJCNN) 2021, 2022
- The Genetic and Evolutionary Computation Conference (GECCO), 2020, 2021, 2022
- The International Conference on Parallel Problem Solving from Nature (PPSN), 2020
- IEEE Congress on Evolutionary Computation (CEC), 2020, 2021, 2022
- The European Conference on Artificial Intelligence (ECAI), 2020
- International Conference on Neural Information Processing, (ICONIP), 2019, 2020, 2021, 2022
- The Evolutionary Computation Journal (ECJ), 2017, 2018, 2019, 2020, 2021
- Australasian Conference on Artificial Life and Computational Intelligence, 2016, 2017, 2018, 2019
- Association for Computing Machinery Membership (ACM), 2018, 2019
- IEEE Computational Intelligence Society Membership, 2017, 2018, 2019
- IEEE Theoretical Foundations of Bio-inspired Computation Task Force, 2017, 2018, 2019, 2020, 2021
- Association for Computing Machinery Membership, SIGEVO 2017-2019
- ECMS Volunteer & Ambassador Program, 2017, 2018, 2019
Presentations and exhibitions:
- SALA, South Australia Living Artists Festival, August 2016, 2017, 2018, 2020
- Media Article, CS Researcher in SALA Art Exhibition, the University of Adelaide
If you have any questions about my work or suggestions for this webpage, please send me an email.
Entry last updated: 9 May 2025
-
Appointments
Date Position Institution name 2023 - ongoing Researcher The University of Adelaide 2019 - 2022 Researcher The University of Adelaide 2016 - 2019 PhD Candidate The University of Adelaide -
Language Competencies
Language Competency English Can peer review German Can peer review Polish Can peer review -
Education
Date Institution name Country Title Kiel University Germany Diplom in Computer Science (Dipl.Inf.) Technical University of Dortmund Germany Vordiplom in Computer Science -
Postgraduate Training
Date Title Institution Country 2017 Introduction to University Teaching University of Adelaide Australia -
Research Interests
Artificial Intelligence Neural, Evolutionary and Fuzzy Computation Optimisation Applied Mathematics Artificial life and complex adaptive systems Artificial Intelligence & Image Processing Simulation and Modelling Renewable energy Energy storage, distribution and supply Public Health Human-computer interaction Medical and Health Sciences Building information modelling and management Stochastic Analysis and Modelling Risk Engineering Mining and extraction of energy resources Applied Statistics Bioinformatics Health Informatics Data engineering and data science Hydrogen-based energy systems Logistics and Supply Chain Management Modelling and simulation Hydrogen production from renewable energy Environmental Engineering Modelling Environmentally sustainable manufacturing activities Production and operations management Operations Research Sustainable design Transportation, logistics and supply chains Sustainability indicators Supply chains
-
Journals
Year Citation 2025 Baguley, S., Friedrich, T., Neumann, A., Neumann, F., Pappik, M., & Zeif, Z. (2025). Fixed Parameter Multi-Objective Evolutionary Algorithms for the <i>W</i>-Separator Problem. ALGORITHMICA, 87(4), 35 pages.
2025 Ahouei, S. S., Antipov, D., Neumann, A., & Neumann, F. (2025). Feature-Based Evolutionary Diversity Optimization of Discriminating Instances for Chance-Constrained Optimization Problems. Lecture Notes in Computer Science, 15610 LNCS, 184-199.
2025 Friedrich, T., Kötzing, T., Neumann, A., Neumann, F., & Radhakrishnan, A. (2025). Analysis of (1+1) EA on LeadingOnes with constraints. Algorithmica: An International Journal in Computer Science.
2025 Santoni, M. L., Raponi, E., Neumann, A., Neumann, F., Preuss, M., & Doerr, C. (2025). Illuminating the diversity-fitness trade-off in black-box optimization. Evolutionary Computation. 2024 Nikfarjam, A., Neumann, A., & Neumann, F. (2024). On the Use of Quality Diversity Algorithms for the Travelling Thief Problem.. ACM Trans. Evol. Learn. Optim., 4, 12. 2024 Dang, D. -C., Neumann, A., Neumann, F., Opris, A., & Sudholt, D. (2024). Theoretical Analysis of Quality Diversity Algorithms for a Classical Path Planning Problem.. CoRR, abs/2412.11446. 2024 Santoni, M. L., Raponi, E., Neumann, A., Neumann, F., Preuss, M., & Doerr, C. (2024). Illuminating the Diversity-Fitness Trade-Off in Black-Box Optimization.. CoRR, abs/2408.16393. 2024 Neumann, A., & Neumann, F. (2024). Optimising Monotone Chance-Constrained Submodular Functions Using Evolutionary Multi-Objective Algorithms. Evolutionary Computation.
2024 Nikfarjam, A., Neumann, A., & Neumann, F. (2024). On the Use of Quality Diversity Algorithms for The Traveling Thief Problem.. ACM Transactions on Evolutionary Learning and Optimization, 4(2), 1-22.
2023 Stimson, M., Reid, W., Neumann, A., Ratcliffe, S., & Neumann, F. (2023). Improving Confidence in Evolutionary Mine Scheduling via Uncertainty Discounting. 2023 IEEE Congress on Evolutionary Computation, CEC 2023, abs/2305.17957, 1-10.
Scopus32023 Ye, F., Neumann, F., Nobel, J. D., Neumann, A., & Bäck, T. (2023). What Performance Indicators to Use for Self-Adaptation in Multi-Objective Evolutionary Algorithms. CoRR, abs/2303.04611.
2023 Neumann, F., Neumann, A., Qian, C., Do, A. V., Nobel, J. D., Vermetten, D., . . . Bäck, T. (2023). Benchmarking Algorithms for Submodular Optimization Problems Using IOHProfiler. CoRR, abs/2302.01464, 1-9.
2023 Perera, K., Neumann, A., & Neumann, F. (2023). Evolutionary Multi-Objective Algorithms for the Knapsack Problems with Stochastic Profits. CoRR, abs/2303.01695.
2023 Lehre, P. K., Neumann, A., & Qian, C. (2023). Special Issue on Theoretical Foundations of Evolutionary Computation. Theor. Comput. Sci., 950, 113785.
2022 Do, A. V., Guo, M., Neumann, A., & Neumann, F. (2022). Analysis of Evolutionary Diversity Optimisation for Permutation Problems. ACM Transactions on Evolutionary Learning and Optimization, 2(3), 1-27.
Scopus172022 Roostapour, V., Neumann, A., & Neumann, F. (2022). Single- and multi-objective evolutionary algorithms for the knapsack problem with dynamically changing constraints. Theoretical Computer Science, 924, 129-147.
Scopus10 WoS22022 Roostapour, V., Neumann, A., Neumann, F., & Friedrich, T. (2022). Pareto optimization for subset selection with dynamic cost constraints.. Artif. Intell., 302, 103597. 2022 Do, A. V., Guo, M., Neumann, A., & Neumann, F. (2022). Niching-based Evolutionary Diversity Optimization for the Traveling Salesperson Problem.. CoRR, abs/2201.10316. 2022 Roostapour, V., Neumann, A., & Neumann, F. (2022). Single- and multi-objective evolutionary algorithms for the knapsack problem with dynamically changing constraints.. Theor. Comput. Sci., 924, 129-147. 2022 Do, A. V., Guo, M., Neumann, A., & Neumann, F. (2022). Analysis of Evolutionary Diversity Optimization for Permutation Problems.. ACM Trans. Evol. Learn. Optim., 2, 11:1. 2022 Roostapour, V., Neumann, A., Neumann, F., & Friedrich, T. (2022). Pareto optimization for subset selection with dynamic cost constraints. Artificial Intelligence, 302, 103597-1-103597-17.
Scopus40 WoS22021 Reid, W., Neumann, A., Ratcliffe, S., & Neumann, F. (2021). Advanced Ore Mine Optimisation under Uncertainty Using Evolution.. CoRR, abs/2102.05235. 2021 Guo, M., Li, J., Neumann, A., Neumann, F., & Nguyen, H. (2021). Practical Fixed-Parameter Algorithms for Defending Active Directory Style Attack Graphs.. CoRR, abs/2112.13175. 2021 Bossek, J., Neumann, A., & Neumann, F. (2021). Breeding Diverse Packings for the Knapsack Problem by Means of Diversity-Tailored Evolutionary Algorithms.. CoRR, abs/2104.13133. 2021 Nikfarjam, A., Bossek, J., Neumann, A., & Neumann, F. (2021). Computing Diverse Sets of High Quality TSP Tours by EAX-Based Evolutionary Diversity Optimisation.. CoRR, abs/2108.05005. 2021 Xie, Y., Neumann, A., Neumann, F., & Sutton, A. M. (2021). Runtime Analysis of RLS and the (1+1) EA for the Chance-constrained Knapsack Problem with Correlated Uniform Weights.. CoRR, abs/2102.05778. 2021 Xie, Y., Neumann, A., & Neumann, F. (2021). Heuristic Strategies for Solving Complex Interacting Stockpile Blending Problem with Chance Constraints.. CoRR, abs/2102.05303. 2021 Neumann, A., Alexander, B., & Neumann, F. (2021). Evolutionary Image Transition and Painting Using Random Walks. Evolutionary Computation, 28(4), 643-675.
2021 Nikfarjam, A., Bossek, J., Neumann, A., & Neumann, F. (2021). Entropy-Based Evolutionary Diversity Optimisation for the Traveling Salesperson Problem.. CoRR, abs/2104.13538. 2020 Xie, Y., Neumann, A., & Neumann, F. (2020). Specific Single- and Multi-Objective Evolutionary Algorithms for the Chance-Constrained Knapsack Problem.. CoRR, abs/2004.03205. 2020 Bossek, J., Neumann, A., & Neumann, F. (2020). Optimising Tours for the Weighted Traveling Salesperson Problem and the Traveling Thief Problem: A Structural Comparison of Solutions.. CoRR, abs/2006.03260. 2020 Neumann, A., & Neumann, F. (2020). Optimising Monotone Chance-Constrained Submodular Functions Using Evolutionary Multi-Objective Algorithms.. CoRR, abs/2006.11444. 2020 Assimi, H., Harper, O., Xie, Y., Neumann, A., & Neumann, F. (2020). Evolutionary Bi-objective Optimization for the Dynamic Chance-Constrained Knapsack Problem Based on Tail Bound Objectives.. CoRR, abs/2002.06766. 2020 Neumann, A., Bossek, J., & Neumann, F. (2020). Computing Diverse Sets of Solutions for Monotone Submodular Optimisation Problems.. CoRR, abs/2010.11486. 2020 Friedrich, T., Kötzing, T., Lagodzinski, J. A. G., Neumann, F., & Schirneck, M. (2020). Analysis of the (1 + 1) EA on subclasses of linear functions under uniform and linear constraints. Theoretical Computer Science, 832, 3-19.
Scopus14 WoS112020 Neumann, A., Alexander, B., & Neumann, F. (2020). Evolutionary Image Transition and Painting Using Random Walks. Evolutionary Computation, 28(4), 643-675.
Scopus11 WoS82020 Do, A. V., Bossek, J., Neumann, A., & Neumann, F. (2020). Evolving Diverse Sets of Tours for the Travelling Salesperson Problem.. CoRR, abs/2004.09188. 2020 Roostapour, V., Neumann, A., & Neumann, F. (2020). Evolutionary Multi-Objective Optimization for the Dynamic Knapsack Problem. CoRR, abs/2004.12574. 2019 Doerr, B., Doerr, C., Neumann, A., Neumann, F., & Sutton, A. M. (2019). Optimization of Chance-Constrained Submodular Functions.. CoRR, abs/1911.11451. 2019 Bossek, J., Kerschke, P., Neumann, A., Neumann, F., & Doerr, C. (2019). One-Shot Decision-Making with and without Surrogates.. CoRR, abs/1912.08956. 2018 Neumann, A., Gao, W., Wagner, M., & Neumann, F. (2018). Evolutionary Diversity Optimization Using Multi-Objective Indicators.. CoRR, abs/1811.06804. 2018 Roostapour, V., Neumann, A., Neumann, F., & Friedrich, T. (2018). Pareto Optimization for Subset Selection with Dynamic Cost Constraints.. CoRR, abs/1811.07806. 2016 Neumann, A., Alexander, B., & Neumann, F. (2016). The Evolutionary Process of Image Transition in Conjunction with Box and Strip Mutation.. CoRR, abs/1608.01783. - Goel, D., Ward, M., Neumann, A., Neumann, F., Nguyen, H., & Guo, M. (n.d.). Hardening Active Directory Graphs via Evolutionary Diversity Optimization based Policies. ACM Transactions on Evolutionary Learning and Optimization.
-
Conference Papers
-
Conference Items
Year Citation 2022 Goel, D., Ward-Graham, M. H., Neumann, A., Neumann, F., Nguyen, H., & Guo, M. (2022). Defending active directory by combining neural network based dynamic program and evolutionary diversity optimisation.. Poster session presented at the meeting of GECCO. ACM. 2020 Neumann, A., & Neumann, F. (2020). Evolutionary computation for digital art. Poster session presented at the meeting of GECCO 2020 Companion - Proceedings of the 2020 Genetic and Evolutionary Computation Conference Companion. ACM.
DOI Scopus12020 Bossek, J., Neumann, A., & Neumann, F. (2020). Evolutionary Diversity Optimisation. Poster session presented at the meeting of Parallel Problem Solving from Nature, PPSN 2020. 2019 Neumann, A., & Neumann, F. (2019). Evolutionary computation for digital art. Poster session presented at the meeting of Abstracts of the Genetic and Evolutionary Computation Conference Companion (GECCO 2019). Prague, The Czech Republic: Association for Computing Machinery (ACM).
DOI Scopus1 WoS12018 Neumann, A., & Neumann, F. (2018). Evolutionary computation for digital art. Poster session presented at the meeting of Abstracts of the Genetic and Evolutionary Computation Conference Companion (GECCO 2018). Kyoto, Japan: Association for Computing Machinery.
DOI Scopus2 -
Original Creative Works
Year Citation 2018 Authors: Neumann A. Title: Art Exhibition in SALA, the South Australian Living Arts Festival 2018. Description: N/A. Extent: 1-31 Aug 2018. 2016 Authors: Neumann A. Title: ART EXHIBITION SALA 2016, THE UNIVERSITY OF ADELAIDE STUDENT ART EXHIBITION, the South Australian Living Arts Festival 2016 (SALA2016). Description: N/A. Extent: ART EXHIBITION. -
Internet Publications
Year Citation 2019 Xie, Y., Harper, O., Assimi, H., Neumann, A., & Neumann, F. (2019). Evolutionary algorithms for the chance-constrained knapsack problem.. ACM. 2017 Neumann, A., Neumann, F., & Friedrich, T. (2017). Quasi-random Agents for Image Transition and Animation.. 2016 Neumann, A., Alexander, B., & Neumann, F. (2016). Evolutionary Image Transition Based on Theoretical Insights of Random Processes.. -
Preprint
Year Citation 2024 Yan, X., Neumann, A., & Neumann, F. (2024). Sliding Window Bi-Objective Evolutionary Algorithms for Optimizing Chance-Constrained Monotone Submodular Functions.. 2024 Pathiranage, I. H., Neumann, F., Antipov, D., & Neumann, A. (2024). Using 3-Objective Evolutionary Algorithms for the Dynamic Chance Constrained Knapsack Problem.. 2024 Antipov, D., Neumann, A., & Neumann, F. (2024). Local Optima in Diversity Optimization: Non-trivial Offspring Population is Essential.. 2024 Do, A. V., Guo, M., Neumann, A., & Neumann, F. (2024). Evolutionary Multi-Objective Diversity Optimization.. 2024 Antipov, D., Neumann, A., Neumann, F., & Sutton, A. M. (2024). Runtime Analysis of Evolutionary Diversity Optimization on the Multi-objective (LeadingOnes, TrailingZeros) Problem.. 2024 Yan, X., Neumann, A., & Neumann, F. (2024). Sampling-based Pareto Optimization for Chance-constrained Monotone Submodular Problems.. 2024 Doerr, B., Knowles, J., Neumann, A., & Neumann, F. (2024). A Block-Coordinate Descent EMO Algorithm: Theoretical and Empirical
Analysis.2024 Ahouei, S. S., Nobel, J. D., Neumann, A., Bäck, T., & Neumann, F. (2024). Evolving Reliable Differentiating Constraints for the Chance-constrained
Maximum Coverage Problem.2024 Harder, J. G., Neumann, A., & Neumann, F. (2024). Analysis of Evolutionary Diversity Optimisation for the Maximum Matching Problem.. 2024 Perera, K. K., & Neumann, A. (2024). Multi-Objective Evolutionary Algorithms with Sliding Window Selection
for the Dynamic Chance-Constrained Knapsack Problem.2024 Santoni, M. L., Raponi, E., Neumann, A., Neumann, F., Preuss, M., & Doerr, C. (2024). Illuminating the Diversity-Fitness Trade-Off in Black-Box Optimization. 2023 Guo, M., Li, J., Neumann, A., Neumann, F., & Nguyen, H. (2023). Limited Query Graph Connectivity Test. 2023 Friedrich, T., Kötzing, T., Neumann, A., Neumann, F., & Radhakrishnan, A. (2023). Analysis of the (1+1) EA on LeadingOnes with Constraints. 2023 Ye, F., Neumann, F., Nobel, J. D., Neumann, A., & Bäck, T. (2023). Towards Self-adaptive Mutation in Evolutionary Multi-Objective
Algorithms.2023 Do, A. V., Neumann, A., Neumann, F., & Sutton, A. M. (2023). Rigorous Runtime Analysis of MOEA/D for Solving Multi-Objective Minimum Weight Base Problems.. 2023 Do, A. V., Guo, M., Neumann, A., & Neumann, F. (2023). Diverse Approximations for Monotone Submodular Maximization Problems with a Matroid Constraint.. 2023 Goel, D., Neumann, A., Neumann, F., Nguyen, H., & Guo, M. (2023). Evolving Reinforcement Learning Environment to Minimize Learner's Achievable Reward: An Application on Hardening Active Directory Systems.. 2023 Guo, M., Li, J., Neumann, A., Neumann, F., & Nguyen, H. (2023). Limited Query Graph Connectivity Test.. 2022 Guo, M., Ward, M., Neumann, A., Neumann, F., & Nguyen, H. (2022). Scalable Edge Blocking Algorithms for Defending Active Directory Style Attack Graphs.. 2018 Neumann, A., Gao, W., Doerr, C., Neumann, F., & Wagner, M. (2018). Discrepancy-based Evolutionary Diversity Optimization..
2025 NHMRC Ideas Grant "Not everyone has the same 24 hours in a day: Addressing time inequality through AI-based personalised time-use interventions", National Health and Medical Research Council, 2025-2028,
2024 Defence Innovation Partnership Grant, Warm Starts and Goal-based Heuristics to Improve MARL Performance in Advanced Distributed Defence: University of Adelaide: Claudia Szabo, Mingyu Guo, Frank Neumann, Aneta Neumann, University of South Australia: Ryszard Kowalczyk, Jimmy Cao, DEWC Services: Tim McKay, Defence Science and Technology Group: Eranda Galhenage,
2022 Lorentz Center Grant, Social Influence Analysis, The Netherlands,
2022 The Department of Defence Grant, Artificial Intelligence for Decision-making, the Office of National Intelligence (ONI) and the Defence Science and Technology Group (DSTG), “Applying machine learning techniques to games on graphs for the detection and concealment of spatially defined communication networks”, Lead CI,
2022 The Department of Defence Grant, Artificial Intelligence for Decision-making, the Office of National Intelligence (ONI) and the Defence Science and Technology Group (DSTG), “Abstract Game Prototype for Cyber Attack/Defence, CI,
2022 The Department of Defence Grant, Artificial Intelligence for Decision-making, the Office of National Intelligence (ONI) and the Defence Science and Technology Group (DSTG), “Tackling the TTCP CAGE challenge using Monte-Carlo planning for large-scale POMDPs”, CI,
2022 Lorentz Center Grant, Benchmarked: Optimization Meets Machine Learning, Leiden, The Netherlands, Solo CI
2022 Travel support within the Premier’s Research and Industry Fund (PRIF) Research Consortium ‘Unlocking Complex Resources through Lean Processing’, University of Adelaide, Australia,
2020 Travel support within the Premier’s Research and Industry Fund (PRIF) Research Consortium ‘Unlocking Complex Resources through Lean Processing’, University of Adelaide, Australia,
2019 Travel support within the Premier’s Research and Industry Fund (PRIF) Research Consortium ‘Unlocking Complex Resources through Lean Processing’, University of Adelaide, Australia,
2018 Hans-Juergen and Marianna Ohff Research Grant,
2018 ACM-W scholarship sponsored by Google, Microsoft, Oracle,
2018 Association for Computing Machinery (ACM) Travel Grant,
2017 Association for Computing Machinery (ACM) Travel Grant,
2017 EvoStar Travel Bursaries Award,
2016 School of Computer Science Postgraduate Scholarship, University of Adelaide, Australia
- 2024, Invited Lecturer, Evolutionary Computation, 3-year and master students of Computer Science, Sem 2, 3 Units
- 2022, Lecturer and Course Coordinator, Evolutionary Computation, 3-year and master students of Computer Science, Sem 2, 3 Units
- 2021, Invited Lecturer, Evolutionary Computation, 3-year and master students of Computer Science, Sem 2, 3 Units
- 2020, Pearson Online Education, co-designer and co-lecturer Master of Data Science (online), Working with Big Data
- 2018, Lecturer, Mining Big Data, 3-year and master students of Computer Science, Sem 1, 3 Units
- 2017, EdX MOOC, co-designer and co-lecturer Big Data Fundamentals, MicroMasters
- 2017, Lecturer, Foundations of Computer Science, Master of Computing and Innovation, Sem 1, 6 Units
- 2017, University of Adelaide, School of Computer Science, Australia, Areas: Supervisor: Introduction to Programming Processing, EdX Course: Think. Create. Code, Sem 1
- 2016, University of Adelaide, School of Computer Science, Australia, Areas: Supervisor: Introduction to Programming Processing, EdX Course: Think. Create. Code, Sem 2
- 2012, University of Adelaide, School of Computer Science, Australia, Areas: Supervisor: Object-oriented programming in Java
- 2012, University of Adelaide, School of Computer Science, Australia, Areas: Supervisor: Internet Computing
- 2011, University of Adelaide, School of Computer Science, Australia, Areas: Supervisor: Object-oriented programming in Java
- 2011, University of Adelaide, School of Computer Science, Australia, Areas: Supervisor: Introduction to programming for engineers (Matlab/C)
-
Current Higher Degree by Research Supervision (University of Adelaide)
Date Role Research Topic Program Degree Type Student Load Student Name 2023 Principal Supervisor Artificial intelligence approaches for increasing the productivity in the mining Doctor of Philosophy Doctorate Full Time Mrs Ishara Udayanthi Hewa Pathiranage 2022 Co-Supervisor Bio-inspired Computing for Problems with Chance Constraints Doctor of Philosophy Doctorate Full Time Mr Xiankun Yan 2022 Principal Supervisor Bio-inspired Computing for Problems with Chance Constraints Doctor of Philosophy Doctorate Full Time Mrs Kokila Perera 2022 Co-Supervisor Bio-inspired algorithms for stochastic multi-component problems Doctor of Philosophy Doctorate Full Time Mr Thilina Pathirage Don -
Past Higher Degree by Research Supervision (University of Adelaide)
Date Role Research Topic Program Degree Type Student Load Student Name 2020 - 2023 Co-Supervisor Evolutionary Diversity Optimisation for Combinatorial Problems Doctor of Philosophy Doctorate Full Time Mr Adel Nikfarjam 2020 - 2024 Co-Supervisor Analysis of Search Heuristics for Diverse Solutions to Combinatorial Problems Doctor of Philosophy Doctorate Full Time Mr Viet Anh Do 2019 - 2021 Co-Supervisor Bio-Inspired Computing for Chance-Constrained Combinatorial Optimisation Problems Doctor of Philosophy Doctorate Full Time Miss Yue Xie -
Other Supervision Activities
Date Role Research Topic Location Program Supervision Type Student Load Student Name 2023 - ongoing Principal Supervisor Evolutionary Optimization for Real-word Applications The University of Adelaide - Doctorate - Ishara Hewa Pathiranage 2022 - ongoing Principal Supervisor Evolutionary Optimization with Chance-Constraints The University of Adelaide - Doctorate - Kokila Perera 2017 - ongoing Co-Supervisor Using Deep Learning to Discover Image Maximising Aesthetic Features Department of Computer Science - Other - Ryan Matulick 2017 - 2018 Co-Supervisor Evolution of Images with Diversity and Constraints using a Generator Network Department of Computer Science Computer Science Student and Winner of ECMS Summer Research Scholarship 2017 Other - Christo Pyromallis 2017 - 2017 Co-Supervisor Evolution of Images with Diversity and Constraints Using Deep-learned Surrogate Functions Department of Computer Science - Other - Christo Pyromallis 2016 - 2017 Co-Supervisor Evolution of artistic image variants through feature based diversity optimisation Department of Computer Science Computer Science Student and Winner of ECMS Summer Research Scholarship 2016 Other - James Kortman
-
Committee Memberships
Date Role Committee Institution Country 2018 - ongoing Member Australasian Joint Conference on Artificial Intelligence AI 2018 Australia 2018 - ongoing Member IEEE Congress on Evolutionary Computation - - 2016 - ongoing Member Australasian Conference on Artificial Life and Computational Intelligence (ACALCI 2017) - - -
Memberships
Date Role Membership Country 2018 - ongoing Member Association for Computing Machinery (ACM) United States 2017 - ongoing - ACM SIGEVO United States 2017 - ongoing - IEEE Theoretical Foundations of Bio-inspired Computation Task Force United Kingdom 2017 - ongoing - IEEE Membership United States 2017 - ongoing - IEEE Computational Intelligence Society United States 2017 - ongoing - IEEE Women in Engineering United States 2016 - ongoing - Australian Network for Art and Technology Australia 2016 - ongoing - ASIA PACIFIC NEURAL NETWORK SOCIETY (APNNS) Japan 2015 - ongoing - University Theatre Guild Australia
Connect With Me
External Profiles