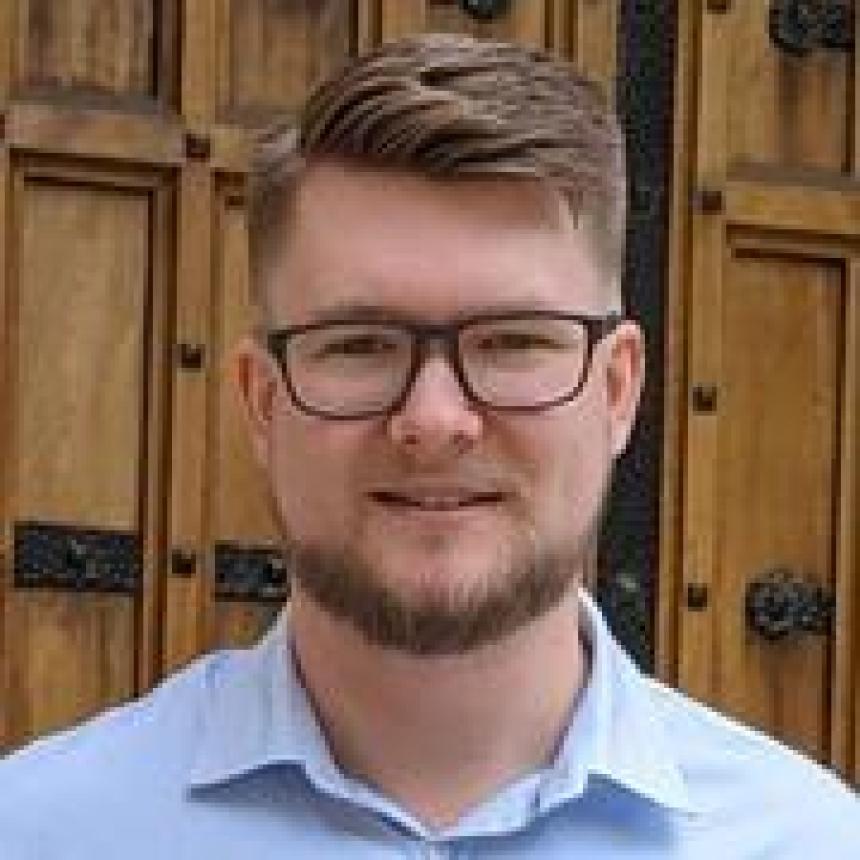
James Bockman
Higher Degree by Research Candidate
HDR Student
School of Computer and Mathematical Sciences
Faculty of Sciences, Engineering and Technology
As the use of autonomous systems in solving complex problems increases, so too does the demand for decision-making models capable of adapting in response to dynamic environments in real time. Suitable inference techniques in computer vision to achieve most decision enabling tasks exist, and the visual domain provides a rich source of data that can be used to inform agents. Time, however, is a critical constraint: existing inference techniques typically require a large number of computational resources and cannot produce decisions rapidly enough to enable agents to keep pace with human perception. The primary focus of my research is to address the requirement for real-time analysis of visual input enabling decision making in autonomous systems in addition to ways lower inference time can be exploited in the creation of new data modelling techniques.
-
Education
Date Institution name Country Title University of Adelaide Australia Bachelor of Science (Physics & Chemistry) University of Adelaide Australia Master of Data Science
-
Journals
Year Citation 2023 Sun, L., Bockman, J., & Sun, C. (2023). A Framework for Leveraging Inter-image Information in Stereo Images for Enhanced Semantic Segmentation in Autonomous Driving. IEEE Transactions on Instrumentation and Measurement, 72, 1.
Scopus92023 Sai, N., Bockman, J. P., Chen, H., Watson-Haigh, N., Xu, B., Feng, X., . . . Gilliham, M. (2023). StomaAI: an efficient and user-friendly tool for measurement of stomatal pores and density using deep computer vision. New Phytologist, 238(2), 904-915.
Scopus13 WoS3 Europe PMC102022 Sai, N., Bockman, J. P., Chen, H., Watson-Haigh, N., Xu, B., Feng, X., . . . Gilliham, M. (2022). SAI: Fast and automated quantification of stomatal parameters on microscope images.
Europe PMC32022 Howe, M., Bockman, J., Orenstein, A., Podgorski, S., Bahrami, S., & Reid, I. (2022). The Edge of Disaster: A Battle Between Autonomous Racing and Safety. 1st ICML Workshop on Safe Learning for Autonomous Driving (SL4AD),
2022. -
Conference Papers
Year Citation 2022 Sachdeva, R., Hammond, R., Bockman, J., Arthur, A., Smart, B., Craggs, D., . . . Reid, I. (2022). Autonomy and Perception for Space Mining. In 2022 International Conference on Robotics and Automation (ICRA) (pp. 4087-4093). Philadelphia, PA, USA: IEEE.
DOI Scopus4 -
Datasets
Year Citation - Bockman, J. (n.d.). resnet18-smoothl1-lr1e-4-bs124 Video and logs.
DOI -
Preprint
Year Citation 2024 Bockman, J., Howe, M., Orenstein, A., & Dayoub, F. (2024). AARK: An Open Toolkit for Autonomous Racing Research.
Algorithm Design & Data Structures - SS 2019
Introduction to Programming (MATLAB & C) - S1/2 2019, S1 2020
Courseware Developer - Introduction to MATLAB
Connect With Me
External Profiles