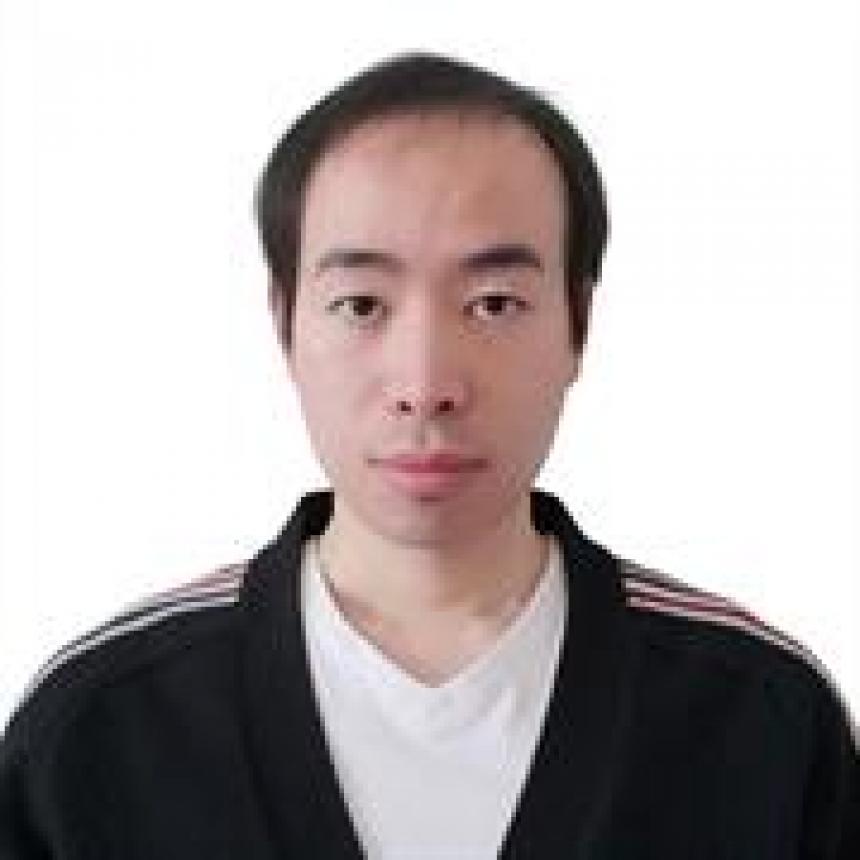
Dr Xinchen Zhang
Grant-Funded Researcher (A)
School of Electrical and Mechanical Engineering
Faculty of Sciences, Engineering and Technology
My PhD research is focused on fundamental research of particle-laden flows by numerical methods.
-
Language Competencies
Language Competency Chinese (Mandarin) Can read, write, speak, understand spoken and peer review English Can read, write, speak, understand spoken and peer review -
Education
Date Institution name Country Title University of Adelaide Australia PhD University of Adelaide Australia Master Northwestern Polytechnical University China Bachelor -
Research Interests
-
Journals
-
Conference Papers
Year Citation 2023 Zhang, X., Nathan, G., Tian, Z., & Chin, R. (2023). Spread of bi-disperse particles in the downstream domain of a turbulent co-flowing jet. In Proceedings of the 14th International ERCOFTAC Symposium on Engineering Turbulence Modelling and Measurements. Barcelona, Spain. 2022 Zhang, X., Zhang, Z., Chinnici, A., Sun, Z., Shi, J., Nathan, G., & Chin, R. (2022). Physics-informed data-driven RANS turbulence modelling for single-phase and particle-laden jet flows. In Proceedings of the 23nd Australasian Fluid Mechanics Conference. Sydney. 2021 Zhang, X., Nathan, G. J., Tian, Z. F., & Chin, R. C. (2021). Flow Regimes and Secondary Flow Motions in Horizontal Particle-laden Pipe Flows. In The 3rd International Symposium on Computational Particle Technology. Suzhou, China and Online. 2021 Zhang, X., Zonta, F., Tian, Z. F., Nathan, G. J., Chin, R. C., & Soldati, A. (2021). Dynamics of semi- and neutrally-buoyant particles in thermally stratified turbulent channel flow. In Euromech. Colloquium 609 Granular Patterns in Oscillatory Flows. Genoa, Italy. 2020 Zhang, X., Nathan, G., Tian, Z. F., & Chin, R. C. (2020). A numerical study of gravity effects on horizontal particle-laden pipe flows. In Proceedings of the 22nd Australasian Fluid Mechanics Conference AFMC2020 (pp. 4 pages). Australia: The University of Queensland.
2019 Zhang, X., Nathan, G., Tian, Z., & Chin, R. (2019). Numerical study of gravity effects on the symmetry and development of particle-laden flows. In 17th European Turbulence Conference. Torino, Italy. 2018 Zhang, X., & Chin, R. (2018). Numerical study of the effects of velocity ratio on co-flow jet characteristics. In Proceedings of the 21nd Australasian Fluid Mechanics Conference AFMC2018 (pp. 1-4). Adelaide, Australia: Australian Fluid Mechanics Society.
Connect With Me
External Profiles